AutoLoc: Weakly-supervised Temporal Action Localization
arXiv: Computer Vision and Pattern Recognition(2018)
摘要
Temporal Action Localization (TAL) in untrimmed video is important for many applications. But it is very expensive to annotate the segment-level ground truth (action class and temporal boundary). This raises the interest of addressing TAL with weak supervision, namely only video-level annotations are available during training). However, the state-of-the-art weakly-supervised TAL methods only focus on generating good Class Activation Sequence (CAS) over time but conduct simple thresholding on CAS to localize actions. In this paper, we first develop a novel weakly-supervised TAL framework called AutoLoc to directly predict the temporal boundary of each action instance. We propose a novel Outer-Inner-Contrastive (OIC) loss to automatically discover the needed segment-level supervision for training such a boundary predictor. Our method achieves dramatically improved performance: under the IoU threshold 0.5, our method improves mAP on THUMOS'14 from 13.7 from 7.4 weakly-supervised method achieves comparable results with some fully-supervised methods.
更多查看译文
关键词
Temporal action localization, Weak supervision, Outer-Inner-contrastive, Class activation sequence
AI 理解论文
溯源树
样例
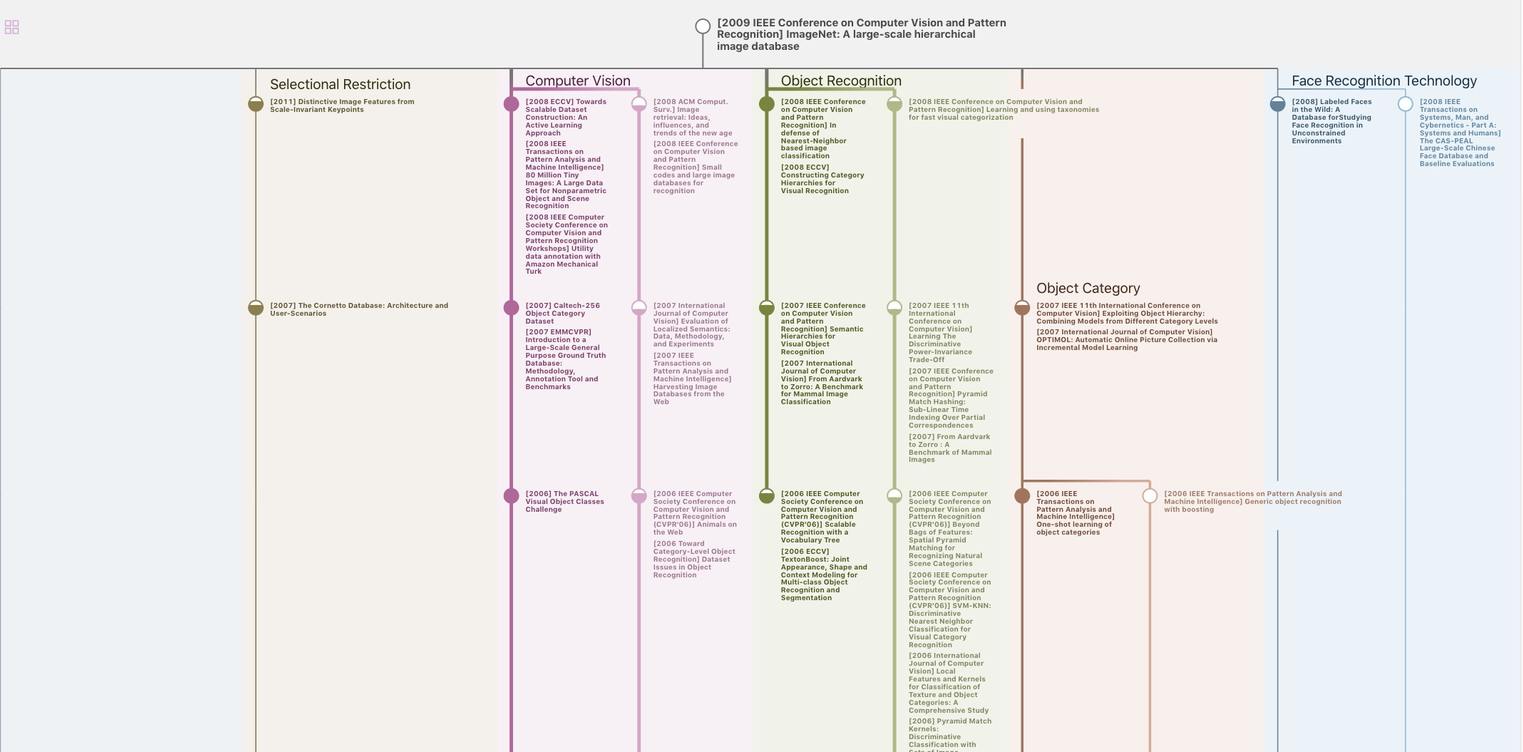
生成溯源树,研究论文发展脉络
Chat Paper
正在生成论文摘要