Deep context: end-to-end contextual speech recognition.
2018 IEEE Spoken Language Technology Workshop (SLT)(2018)
摘要
In automatic speech recognition (ASR) what a user says depends on the particular context she is in. Typically, this context is represented as a set of word n-grams. In this work, we present a novel, all-neural, end-to-end (E2E) ASR system that utilizes such context. Our approach, which we refer to as Contextual Listen, Attend and Spell (CLAS) jointly-optimizes the ASR components along with embeddings of the context n-grams. During inference, the CLAS system can be presented with context phrases which might contain-of-vocabulary (OOV) terms not seen during training. We compare our proposed system to a more traditional contextualization approach, which performs shallow-fusion between independently trained LAS and contextual n-gram models during beam search. Across a number of tasks, we find that the proposed CLAS system outperforms the baseline method by as much as 68% relative WER, indicating the advantage of joint optimization over individually trained components.
更多查看译文
关键词
Context modeling,Decoding,Training,Speech recognition,Standards,Acoustics,Task analysis
AI 理解论文
溯源树
样例
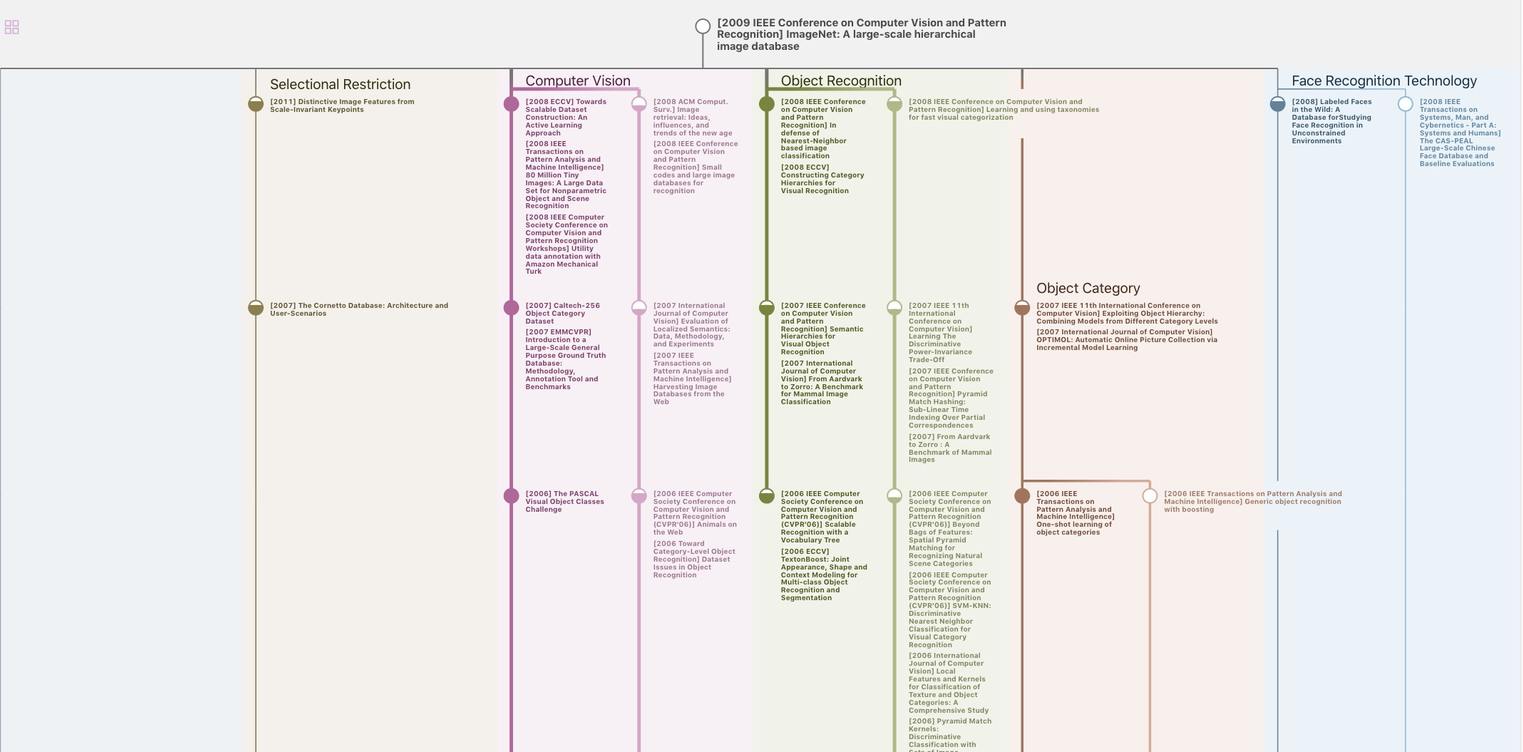
生成溯源树,研究论文发展脉络
Chat Paper
正在生成论文摘要