Efficient Principal Subspace Projection of Streaming Data Through Fast Similarity Matching
2018 IEEE INTERNATIONAL CONFERENCE ON BIG DATA (BIG DATA)(2018)
摘要
Big data problems frequently require processing datasets in a streaming fashion, either because all data are available at once but collectively are larger than available memory or because the data intrinsically arrive one data point at a time and must be processed online. Here, we introduce a computationally efficient version of similarity matching, a framework for online dimensionality reduction that incrementally estimates the top K-dimensional principal subspace of streamed data while keeping in memory only the last sample and the current iterate. To assess the performance of our approach, we construct and make public a test suite containing both a synthetic data generator and the infrastructure to test online dimensionality reduction algorithms on real datasets, as well as performant implementations of our algorithm and competing algorithms with similar aims. Among the algorithms considered we find our approach to be competitive, performing among the best on both synthetic and real data.
更多查看译文
关键词
principal component analysis,dimensionality reduction,online algorithms
AI 理解论文
溯源树
样例
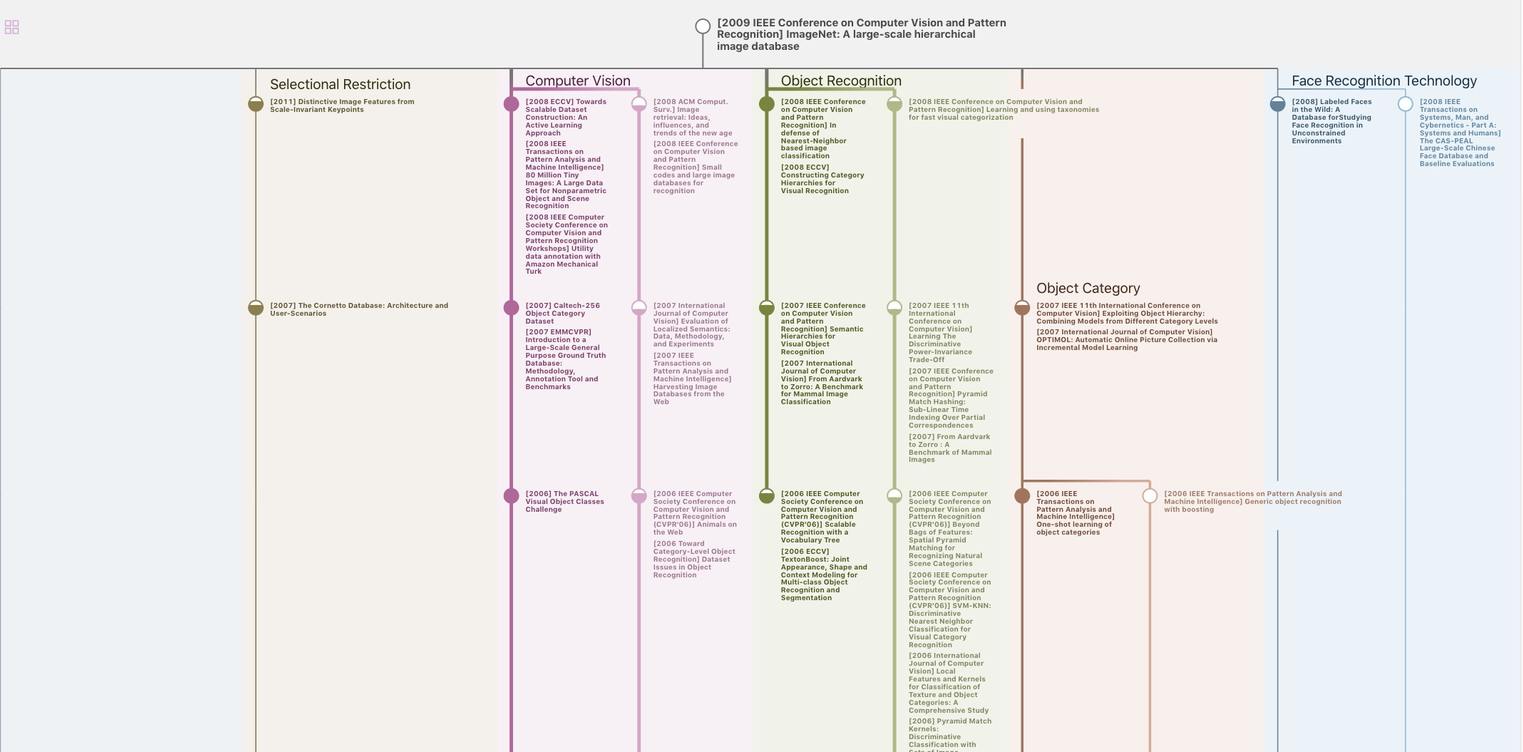
生成溯源树,研究论文发展脉络
Chat Paper
正在生成论文摘要