PCN: Point Completion Network
2018 International Conference on 3D Vision (3DV)(2018)
摘要
Shape completion, the problem of estimating the complete geometry of objects from partial observations, lies at the core of many vision and robotics applications. In this work, we propose Point Completion Network (PCN), a novel learning-based approach for shape completion. Unlike existing shape completion methods, PCN directly operates on raw point clouds without any structural assumption (e.g. symmetry) or annotation (e.g. semantic class) about the underlying shape. It features a decoder design that enables the generation of fine-grained completions while maintaining a small number of parameters. Our experiments show that PCN produces dense, complete point clouds with realistic structures in the missing regions on inputs with various levels of incompleteness and noise, including cars from LiDAR scans in the KITTI dataset.
更多查看译文
关键词
shape completion,learning on point clouds,3D reconstruction,point cloud registration
AI 理解论文
溯源树
样例
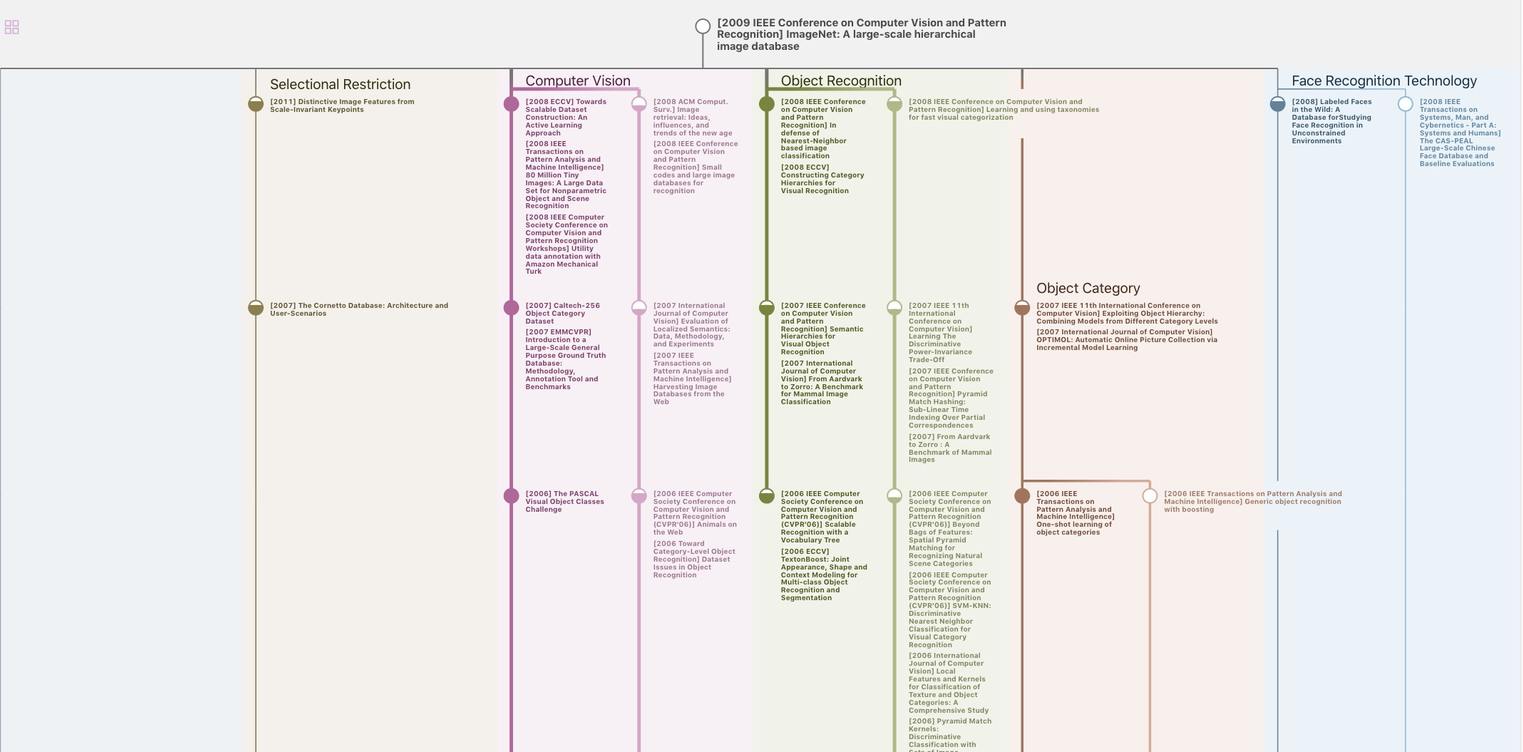
生成溯源树,研究论文发展脉络
Chat Paper
正在生成论文摘要