Multi-level preference regression for cold-start recommendations
Int. J. Machine Learning & Cybernetics(2017)
摘要
Due to the absence of historical ratings of new users/items, cold-start recommendation remains a challenge for collaborative filtering. Many matrix factorization based methods are used to predict new user’s/item’s latent profile before predicting ratings. This kind of methods is usually non-convex. In this work, we design a new convex framework for cold-start recommendations, multi-level preference regression (MPR), directly to predict the ratings rather than latent profiles. We suppose that ratings are mainly affected by three components: (1) correlation between user’s attributes (such as age and gender) and item’s attributes (such as genre and producer); (2) each user’s preference on item’s attributes; (3) item’s popularity in a group of users with some attributes. Adjusting the impact of the three components, we can tackle three cold-start scenarios of user, item, and system. In the MPR framework, three different learning strategies are discussed: pointwise regression, pairwise regression, and large-margin learning. Experimental results on three datasets demonstrate that the proposed model can achieve the state of the art in the user cold-start scenario and the best performance in other scenarios.
更多查看译文
关键词
Recommendation system,Cold-start recommendation,Preference regression
AI 理解论文
溯源树
样例
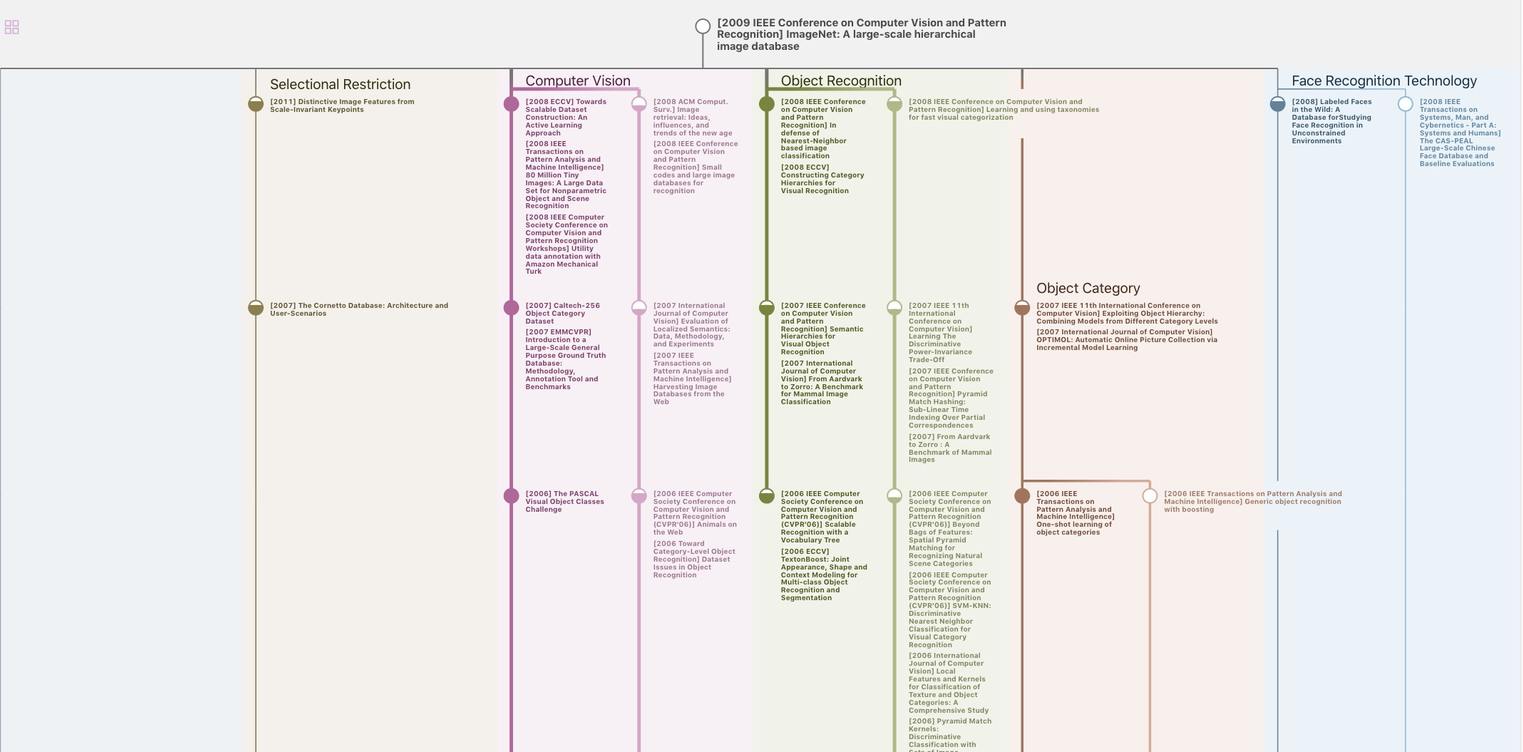
生成溯源树,研究论文发展脉络
Chat Paper
正在生成论文摘要