On-chip unsupervised learning in winner-take-all networks of spiking neurons.
BioCAS(2017)
摘要
The ability to learn re-occurring patterns in real-time sensory inputs in an unsupervised way is a key feature of neural networks that can enable them to carry out complex tasks directly, or to simplify the learning process of subsequent layers in powerful deep network configurations. Dedicated neuromorphic computing electronic systems can implement low-power real-time neural network inference engines. However, unsupervised online learning in these systems remains an open challenge. In this paper, we demonstrate spike-based unsupervised learning in a neuromorphic hardware device that has ultra low-power spiking neuron circuits and on-chip plasticity synapse circuits implemented with analog electronics. We configure populations of silicon neurons in a soft winner-take-all (WTA) network configuration, which enables them to learn the classification of different spike-rate input patterns in an unsupervised manner. We demonstrate the ability of this neuromorphic hardware to perform unsupervised learning of the pattern classification in real-time, and characterize its robustness as a function of network parameters and network configuration.
更多查看译文
关键词
spiking neurons,real-time sensory inputs,neural networks,learning process,powerful deep network configurations,dedicated neuromorphic computing electronic systems,real-time neural network inference engines,unsupervised online learning,neuromorphic hardware device,low-power spiking neuron circuits,on-chip plasticity synapse circuits,silicon neurons,different spike-rate input patterns,pattern classification,on-chip unsupervised learning,winner-take-all networks,WTA network configuration
AI 理解论文
溯源树
样例
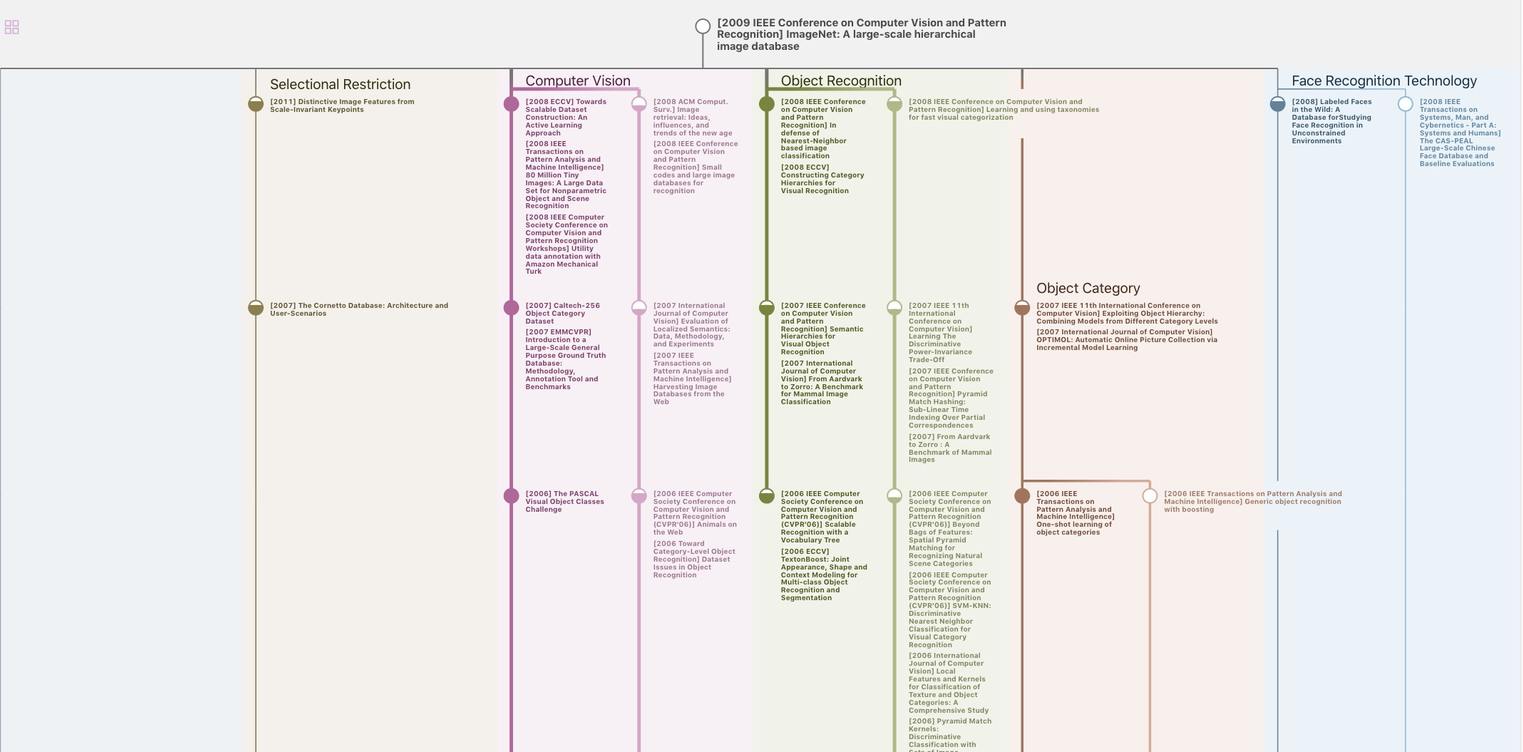
生成溯源树,研究论文发展脉络
Chat Paper
正在生成论文摘要