Active Selection Of Elements For Bayesian Nonnegative Matrix Factorization
SIU(2018)
摘要
In classical matrix completion problems, you can divide the matrix into two groups as observed and unknown elements. In this study, the matrices are composed of three different groups: observed data that is known and accessible at any time without any expense, unknown data that we are trying to predict, and data that is currently unknown but can be queried when desired. When the data in the last group is queried for the first time, a cost arises. From this observation, we want to estimate the unknown data in the second group with the least error by making as few queries as possible. Our goal is to choose the observations we are trying to question wisely. In this study, observation sequence selections were defined and compared in the MovieLens data set.
更多查看译文
关键词
Nonnegative Matrix Factorization,Gibbs Sampler,Active Learning,Recommendation Systems,Bayesian Inference via Monte Carlo
AI 理解论文
溯源树
样例
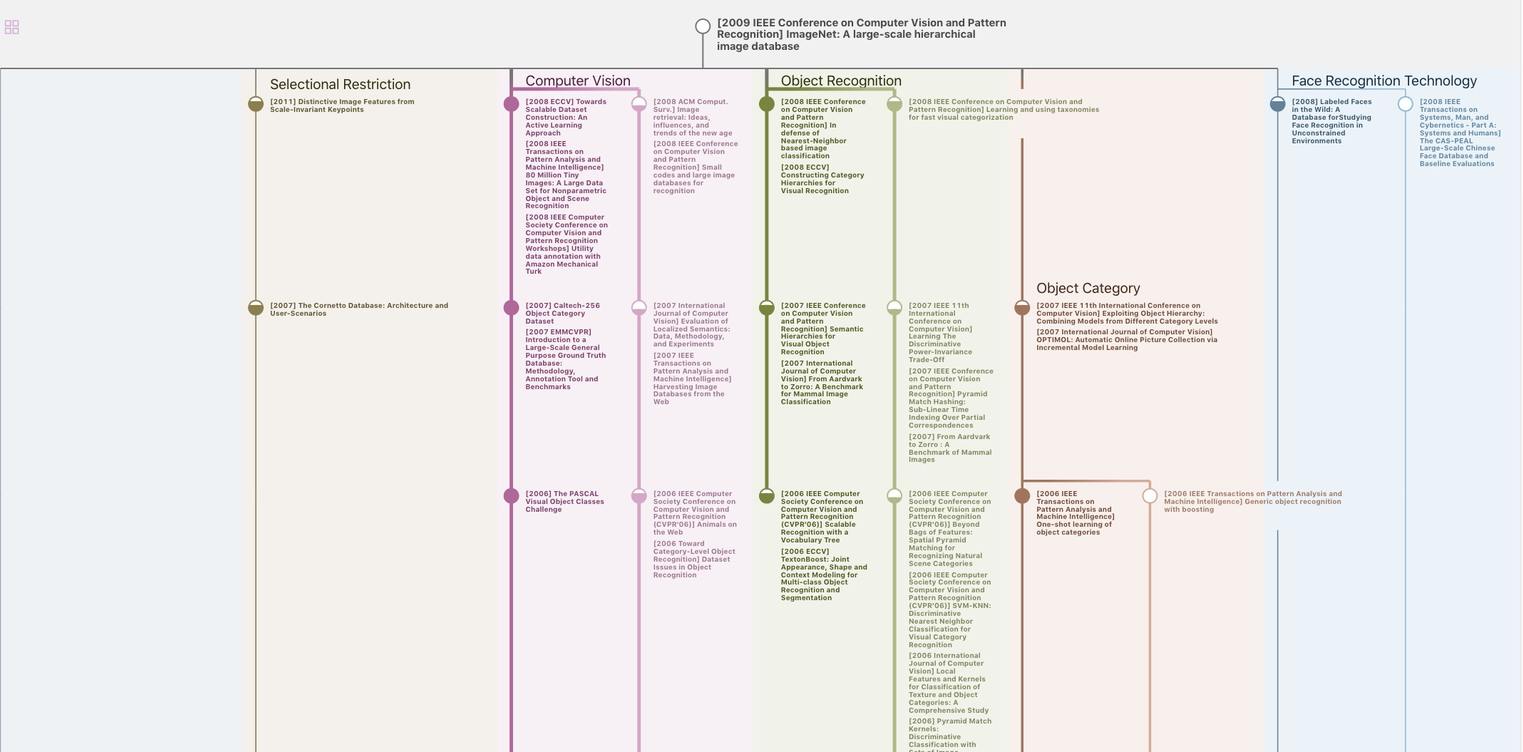
生成溯源树,研究论文发展脉络
Chat Paper
正在生成论文摘要