An Efficient Recommender System By Integrating Non-Negative Matrix Factorization With Trust And Distrust Relationships
2018 IEEE DATA SCIENCE WORKSHOP (DSW)(2018)
摘要
Matrix factorization (MF) has been proved to be an effective approach to build a successful recommender system. However, most current MF-based recommenders cannot obtain high prediction accuracy due to the sparseness of user-item matrix. Moreover, these methods suffer from the scalability issues when applying on large-scale real-world tasks. To tackle these issues, in this paper a social regularization method called TrustRSNMF is proposed that incorporates the social trust information of users in nonnegative matrix factorization framework. The proposed method integrates trust statements along with user-item ratings as an additional information source into the recommendation model to deal with the data sparsity and cold-start issues. In order to evaluate the effectiveness of the proposed method, a number of experiments are performed on two real-world datasets. The obtained results demonstrate significant improvements of the proposed method compared to state-of-the-art recommendation methods.
更多查看译文
关键词
Recommender systems, social Trust, matrix Factorization, distrust Relationships, collaborative filtering
AI 理解论文
溯源树
样例
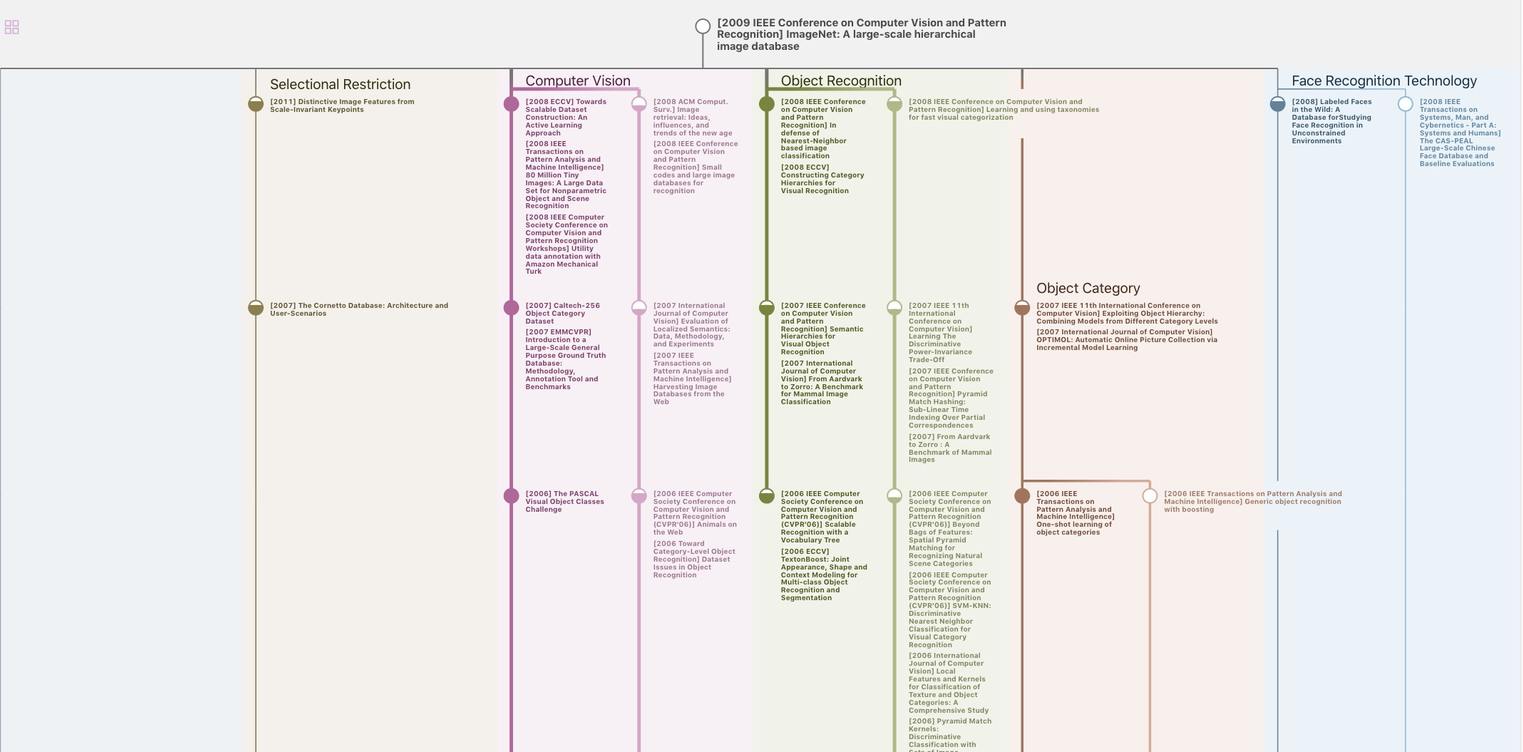
生成溯源树,研究论文发展脉络
Chat Paper
正在生成论文摘要