Dependence-Preserving Data Compaction For Scalable Forensic Analysis
PROCEEDINGS OF THE 27TH USENIX SECURITY SYMPOSIUM(2018)
摘要
Large organizations are increasingly targeted in long-running attack campaigns lastingmonths or years. When a break-in is eventually discovered, forensic analysis begins. System audit logs provide crucial information that underpins such analysis. Unfortunately, audit data collected over months or years can grow to enormous sizes. Large data size is not only a storage concern: forensic analysis tasks can become very slow when they must sift through billions of records. In this paper, we first present two powerful event reduction techniques that reduce the number of records by a factor of 4.6 to 19 in our experiments. An important benefit of our techniques is that they provably preserve the accuracy of forensic analysis tasks such as backtracking and impact analysis. While providing this guarantee, our techniques reduce on-disk file sizes by an average of 35x across our data sets. On average, our in-memory dependence graph uses just 5 bytes per event in the original data. Our system is able to consume and analyze nearly a million events per second.
更多查看译文
关键词
Data compaction,Computer science,Scalability,Computer engineering
AI 理解论文
溯源树
样例
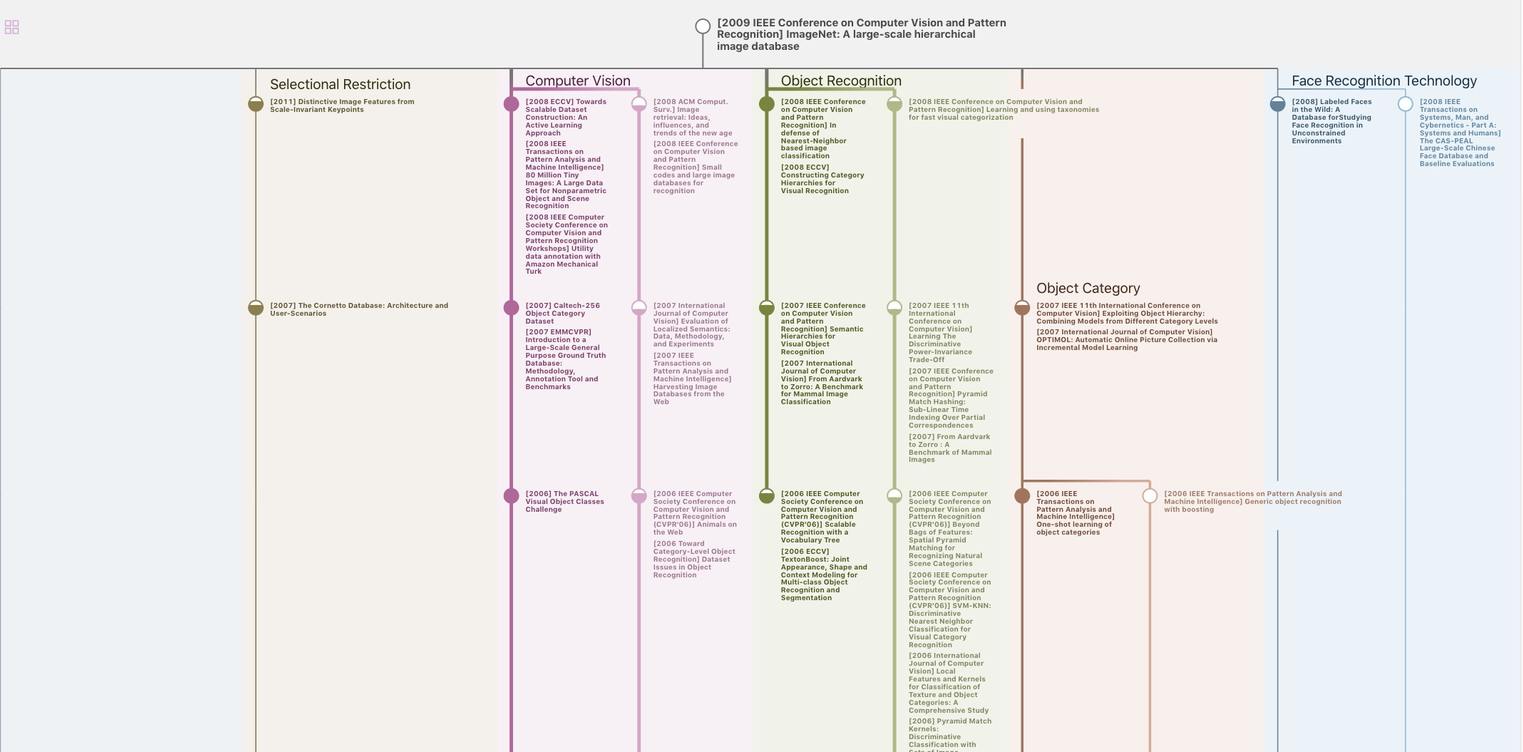
生成溯源树,研究论文发展脉络
Chat Paper
正在生成论文摘要