CDC-MRF for Hyperspectral Data Classification
INTELLIGENT COMPUTING METHODOLOGIES, ICIC 2018, PT III(2018)
摘要
This paper presents a new hyperspectral classification algorithm based on convolutional neural network (CNN). A CNN is first used to learn the posterior class distributions using a patch-wise training strategy to better utilize the spatial information. In order to further extract hyperspectral feature information, we propose a method of extracting features twice (CDC-MRF). In our method, we first use 2D CNN to extract spectral and spatial information of the hyperspectral data. Then, we use deconvolution layer to expand the size of sample which can make the patch contain more useful information. After that we make a second extraction of the features, use 2D CNN for secondary extraction features. After that, the spatial information is further considered by using a Markov random field prior, which encourages the neighboring pixels to have the same labels. Finally, a maximum posteriori segmentation model is efficiently computed by the alpha-expansion min-cut-based optimization algorithm. Experimental results show that, the proposed method achieves state-of-the-art performance on two benchmark HSI datasets.
更多查看译文
关键词
Convolutional neural network, Hyperspectral classification, Deconvolution
AI 理解论文
溯源树
样例
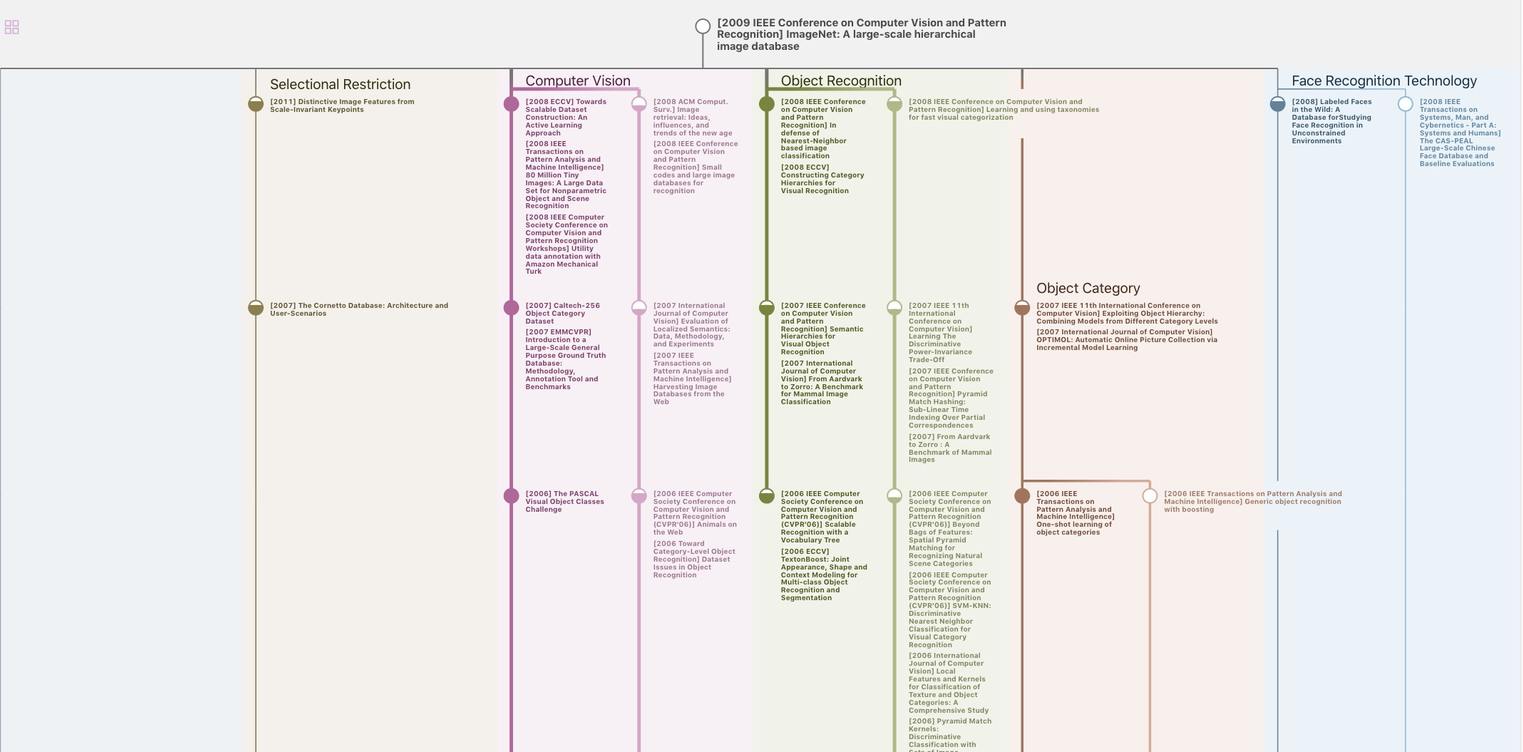
生成溯源树,研究论文发展脉络
Chat Paper
正在生成论文摘要