Convolutional Neural Networks Based for High Spectral Dimensional Data Classification.
DSC(2018)
摘要
Recent research has shown that deep learning is widely used to classify the high spectral dimensional data. Under limited samples, a novel method of high spectral dimensional data classification based on convolutional neural networks (CNNs) is proposed. By standardization and adding virtual samples, the sensitivity of models on the classification of objects and quantity of classification objects is reduced. The computational complexity and computation time are reduced by optimizing the gradient descent method and the learning rate calculation method. By setting up a suitable network structure, the generalization is improved. The experimental results show that compared with the traditional classification methods such as k-Nearest Neighbor (KNN), Logistic Regression (LR) and Support Vector Machine of Radial Basis Function Kernel (RBF-SVM), the method using convolutional neural network has more competitive results in computational efficiency and classification accuracy.
更多查看译文
关键词
high spectral dimensional data,classification,convolutional neural network,virtual samples,cyclical learning rates,gradient method with momentum and batch
AI 理解论文
溯源树
样例
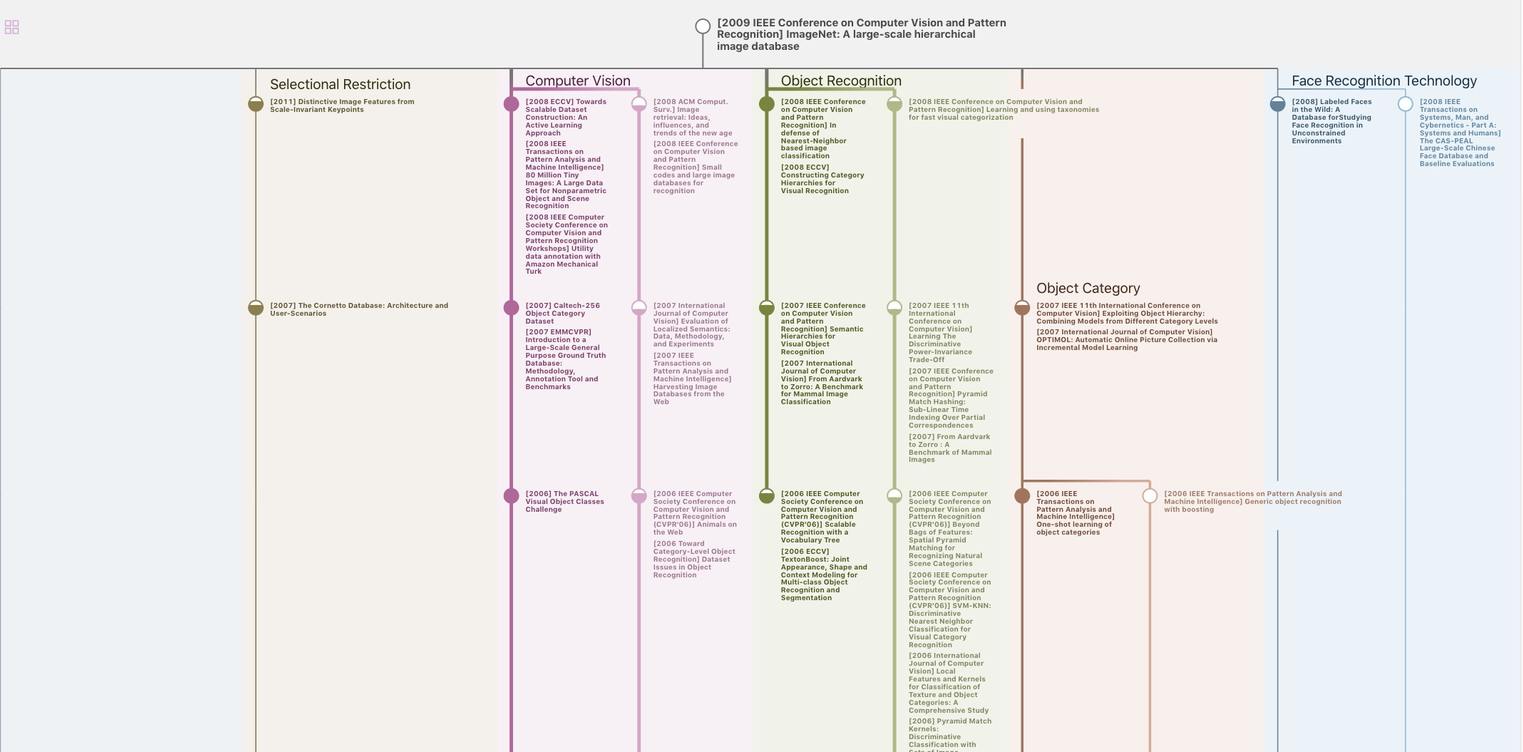
生成溯源树,研究论文发展脉络
Chat Paper
正在生成论文摘要