ULE: Learning User and Location Embeddings for POI Recommendation
2018 IEEE Third International Conference on Data Science in Cyberspace (DSC)(2018)
摘要
Point-of Interest recommendation has become an important application in Location-Based Social Networks. It recommends interesting but unvisited locations for users based on users' historical check-ins and other auxiliary information. To cope with check-ins, existing methods convert them into users' scores on Points-of-interest and fit the scores by users' and locations' latent factors. However, the way of conversion is not appropriate since check-ins are just repeated times, which have no clear correspondence with users' explicit preferences. To cope with auxiliary information, including geographical positions, social connections, etc., existing methods develop many different ways. However, most of them handle each type of auxiliary information separately, and they cannot combine different types of auxiliary information easily and closely in one model. In this paper, we propose a unified framework for Point-of-Interest recommendation which can overcome the two challenges. The framework is composed of two parts, corresponding to the modeling of check-ins and other auxiliary information respectively. First, we split the check-in into check-in relationship and a confidence in the relationship. Considering that one user's preferences to all Points-of-Interest are mutually influenced, we adopt a multinomial distribution to model each user's preferences distribution. Second, we argue that the influences of each type of auxiliary information can be reflected in the interactions of any pair of users or locations. For example, geographical influences are reflected in two locations which are geographically close to each other. We adopt multinomial distribution to model the distribution of each location's (user's) influences on other locations (users), forming a unified framework with the modeling of check-ins. We conduct extensive experiments on two real-world data sets, i.e., Foursquare and Gowalla. The experiment results demonstrate the effectiveness of our framework.
更多查看译文
关键词
Point of Interest,check ins,recommend system
AI 理解论文
溯源树
样例
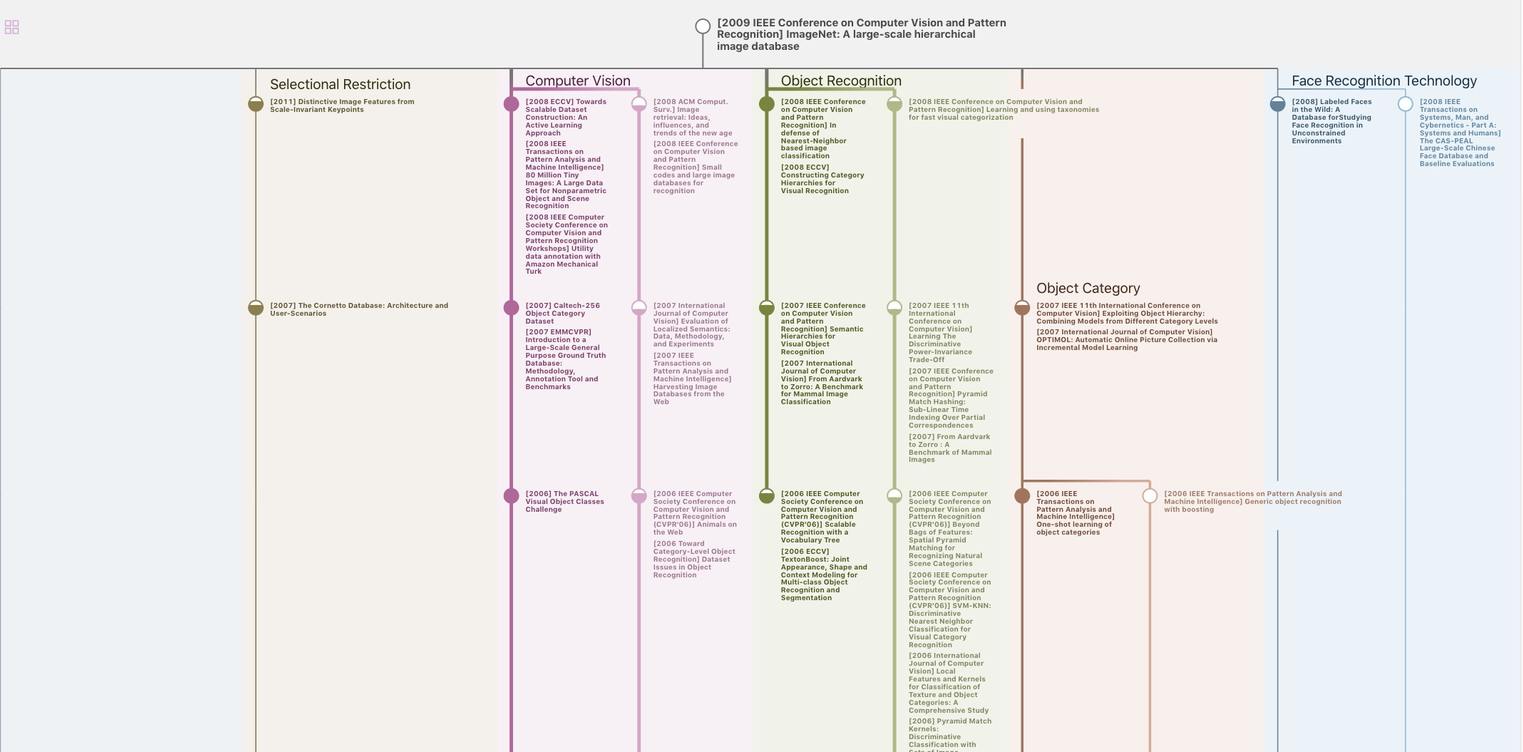
生成溯源树,研究论文发展脉络
Chat Paper
正在生成论文摘要