Genpass: A General Deep Learning Model For Password Guessing With Pcfg Rules And Adversarial Generation
2018 IEEE INTERNATIONAL CONFERENCE ON COMMUNICATIONS (ICC)(2018)
摘要
Password has become today's dominant method of authentication in social network. While the brute-force attack methods, such as HashCat and John the Ripper, are unpractical, the research then switches to the password guess. The stateof-the-art approaches, such as Markov Model and probabilistic context-free grammars(PCFG), are all based on statistical probability. These approaches have a low matching rate. The methods on neural network have been proved more accurate and practical for password guessing than traditional methods. However, a raw neural network model is not qualified for cross-sites attack since each data set has its own features.This paper proposes a general deep learning model for password guessing, called GENPass. GENPass can learn features from several data sets and ensure the output wordlist high accuracy in different data sets by using adversarial generation. The password generator of GENPass is PCFG+LSTM(PL), where LSTM is a kind of Recurrent Neural Network. We combine neural network with PCFG because we found people were used to set their passwords with meaningful strings. Compared with LSTM, PL increased the matching rate by 16%-30% in the cross-sites tests when learning from a single dataset. GENPass uses several PL models to learn datasets and generate passwords. The result shows that the matching rate of GENPass is 20% higher than that of simply mixing those datasets in the cross-sites test.
更多查看译文
关键词
PCFG+LSTM,statistical probability,PL models,cross-sites test,matching rate,Recurrent Neural Network,password generator,raw neural network model,probabilistic context-free grammars,Markov Model,brute-force attack methods,social network,adversarial generation,PCFG rules,password guessing,general deep learning model,GENPass
AI 理解论文
溯源树
样例
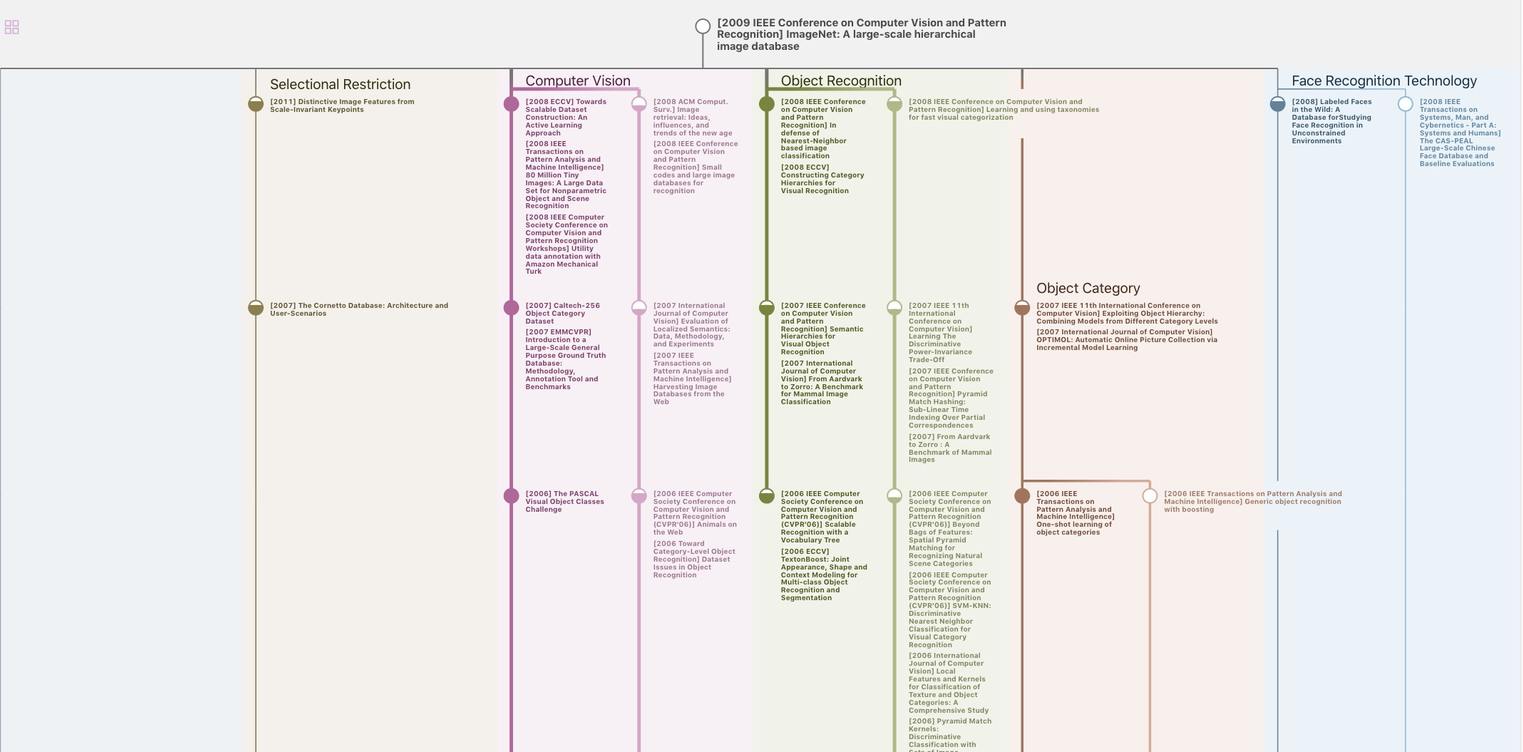
生成溯源树,研究论文发展脉络
Chat Paper
正在生成论文摘要