Trojaning Attack on Neural Networks.
NDSS(2018)
摘要
With the fast spread of machine learning techniques, sharing and adopting public machine learning models become very popular. This gives attackers many new opportunities. In this paper, we propose a trojaning attack on neuron networks. As the models are not intuitive for human to understand, the attack features stealthiness. Deploying trojaned models can cause various severe consequences including endangering human lives (in applications like auto driving). We first inverse the neuron network to generate a general trojan trigger, and then retrain the model with external datasets to inject malicious behaviors to the model. The malicious behaviors are only activated by inputs stamped with the trojan trigger. In our attack, we do not need to tamper with the original training process, which usually takes weeks to months. Instead, it takes minutes to hours to apply our attack. Also, we do not require the datasets that are used to train the model. In practice, the datasets are usually not shared due to privacy or copyright concerns. We use five different applications to demonstrate the power of our attack, and perform a deep analysis on the possible factors that affect the attack. The results show that our attack is highly effective and efficient. The trojaned behaviors can be successfully triggered (with nearly 100% possibility) without affecting its test accuracy for normal input data. Also, it only takes a small amount of time to attack a complex neuron network model. In the end, we also discuss possible defense against such attacks.
更多查看译文
AI 理解论文
溯源树
样例
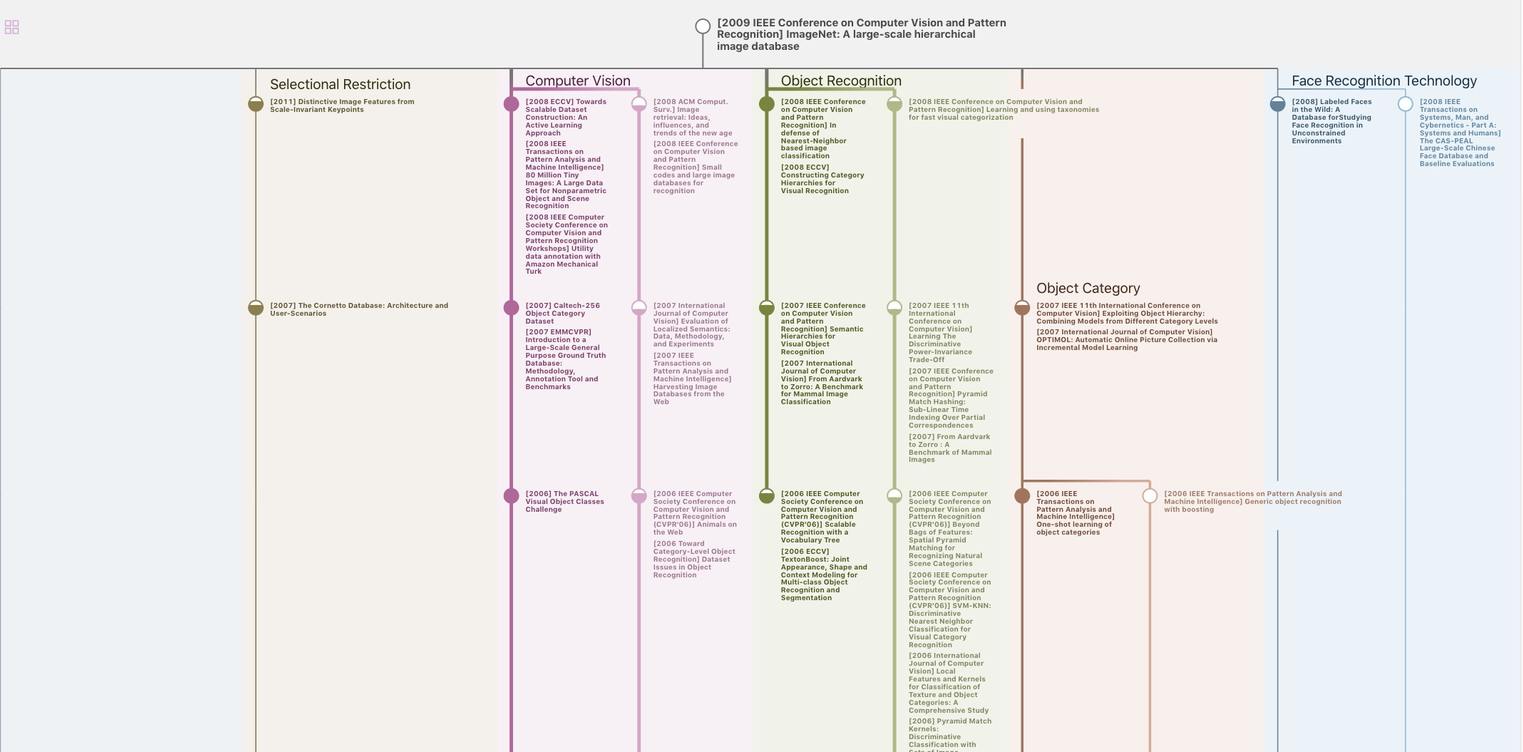
生成溯源树,研究论文发展脉络
Chat Paper
正在生成论文摘要