Effective automated pipeline for 3D reconstruction of synapses based on deep learning
BMC Bioinformatics(2018)
摘要
Background The locations and shapes of synapses are important in reconstructing connectomes and analyzing synaptic plasticity. However, current synapse detection and segmentation methods are still not adequate for accurately acquiring the synaptic connectivity, and they cannot effectively alleviate the burden of synapse validation. Results We propose a fully automated method that relies on deep learning to realize the 3D reconstruction of synapses in electron microscopy (EM) images. The proposed method consists of three main parts: (1) training and employing the faster region convolutional neural networks (R-CNN) algorithm to detect synapses, (2) using the z-continuity of synapses to reduce false positives, and (3) combining the Dijkstra algorithm with the GrabCut algorithm to obtain the segmentation of synaptic clefts. Experimental results were validated by manual tracking, and the effectiveness of our proposed method was demonstrated. The experimental results in anisotropic and isotropic EM volumes demonstrate the effectiveness of our algorithm, and the average precision of our detection (92.8% in anisotropy, 93.5% in isotropy) and segmentation (88.6% in anisotropy, 93.0% in isotropy) suggests that our method achieves state-of-the-art results. Conclusions Our fully automated approach contributes to the development of neuroscience, providing neurologists with a rapid approach for obtaining rich synaptic statistics.
更多查看译文
关键词
3D Reconstruction of synapses,Deep learning,Electron microscope,Synapse detection,Synapse segmentation
AI 理解论文
溯源树
样例
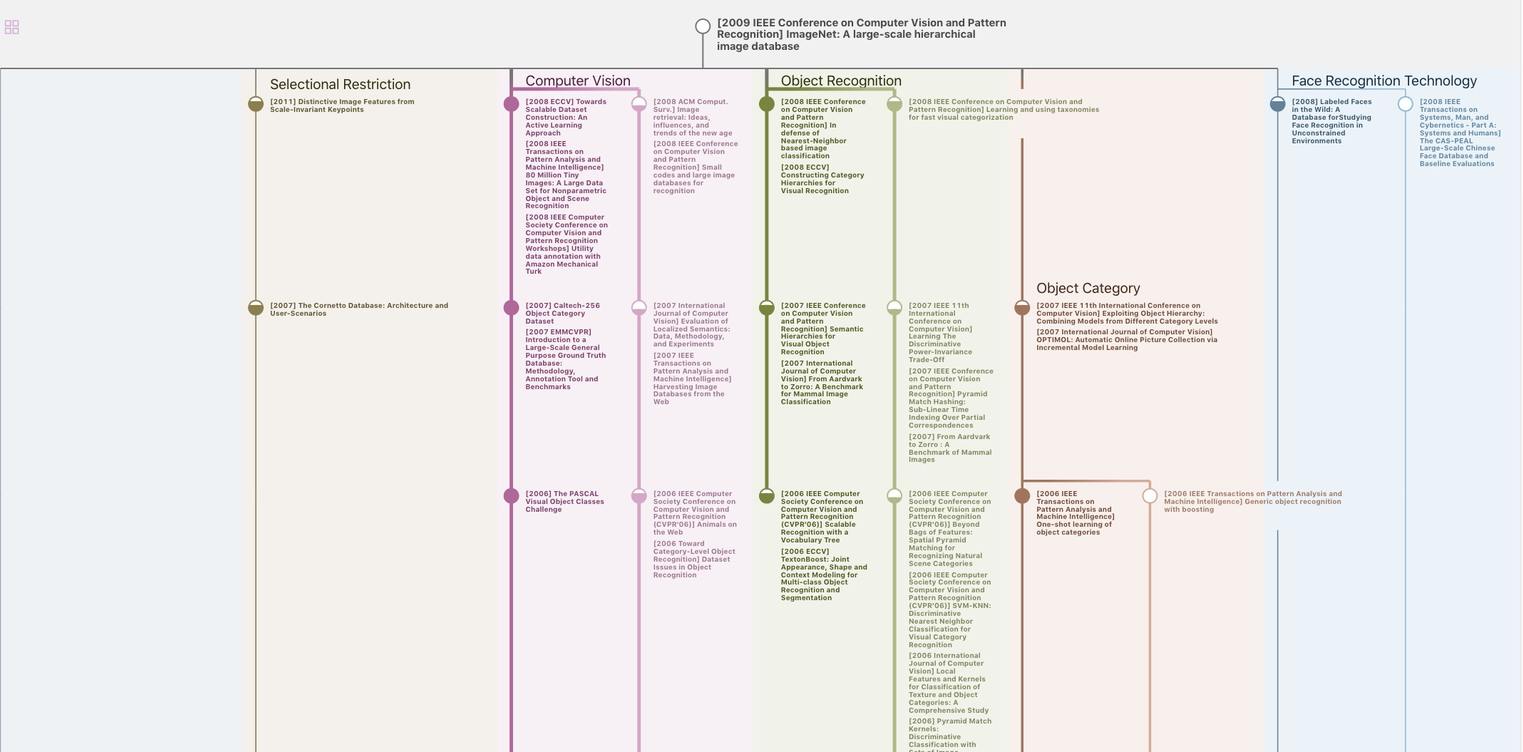
生成溯源树,研究论文发展脉络
Chat Paper
正在生成论文摘要