Co-Training of Audio and Video Representations from Self-Supervised Temporal Synchronization.
arXiv: Computer Vision and Pattern Recognition(2018)
摘要
There is a natural correlation between the visual and auditive elements of a video. In this work we leverage this connection to learn general and effective features for both audio and video analysis from self-supervised temporal synchronization. We demonstrate that a calibrated curriculum learning scheme, a careful choice of negative examples, and the use of a contrastive loss are critical ingredients to obtain powerful multi-sensory representations from models optimized to discern temporal synchronization of audio-video pairs. Without further finetuning, the resulting audio features achieve performance superior or comparable to the state-of-the-art on established audio classification benchmarks (DCASE2014 and ESC-50). At the same time, our visual subnet provides a very effective initialization to improve the accuracy of video-based action recognition models: compared to learning from scratch, our self-supervised pretraining yields a remarkable gain of +16.7% in action recognition accuracy on UCF101 and a boost of +13.0% on HMDB51.
更多查看译文
AI 理解论文
溯源树
样例
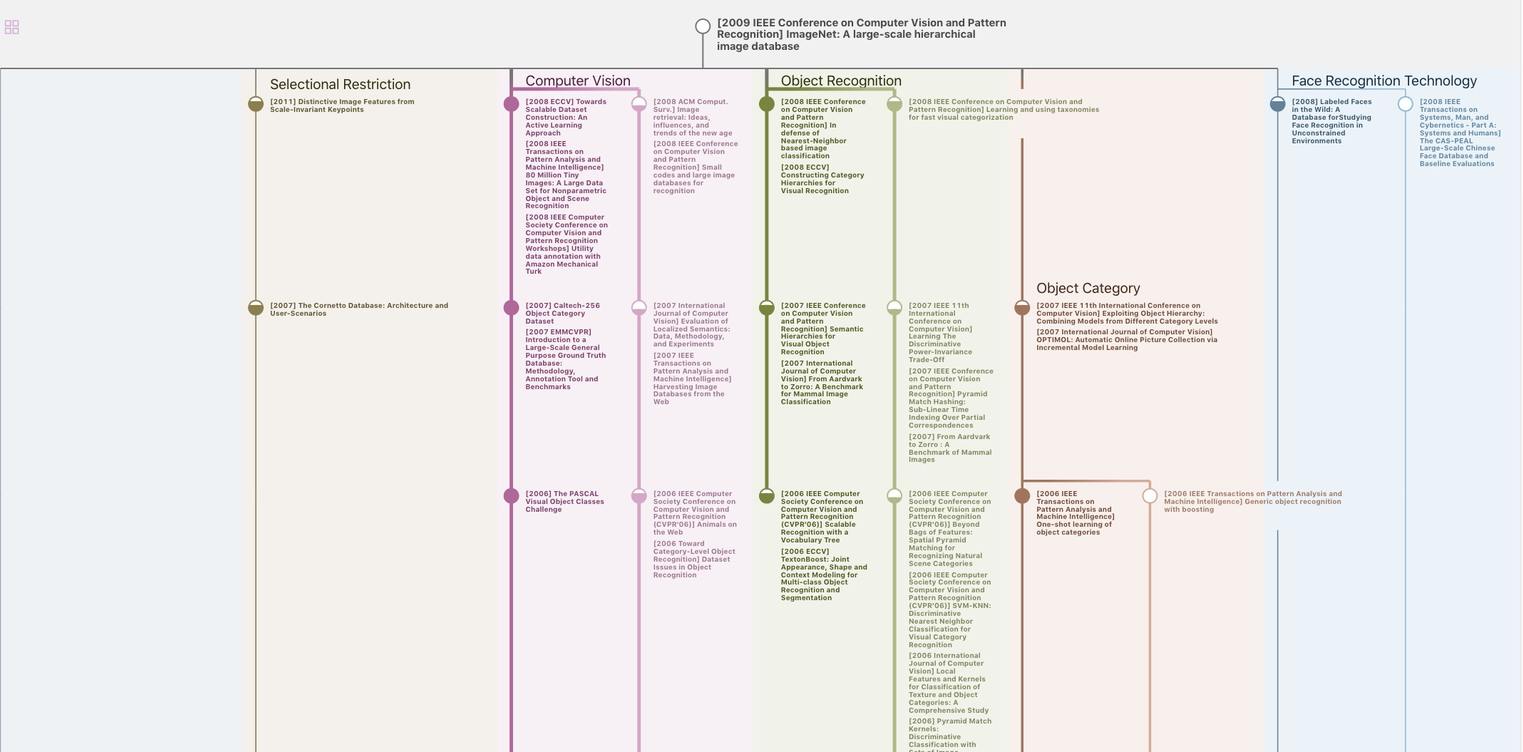
生成溯源树,研究论文发展脉络
Chat Paper
正在生成论文摘要