Importance weighted generative networks.
arXiv: Machine Learning(2018)
摘要
Deep generative networks can simulate from a complex target distribution, by minimizing a loss with respect to samples from that distribution. However, often we do not have direct access to our target distribution - our data may be subject to sample selection bias, or may be from a different but related distribution. We present methods based on importance weighting that can estimate the loss with respect to a target distribution, even if we cannot access that distribution directly, in a variety of settings. These estimators, which differentially weight the contribution of data to the loss function, offer both theoretical guarantees and impressive empirical performance.
更多查看译文
关键词
Importance weights, Generative networks, Bias correction
AI 理解论文
溯源树
样例
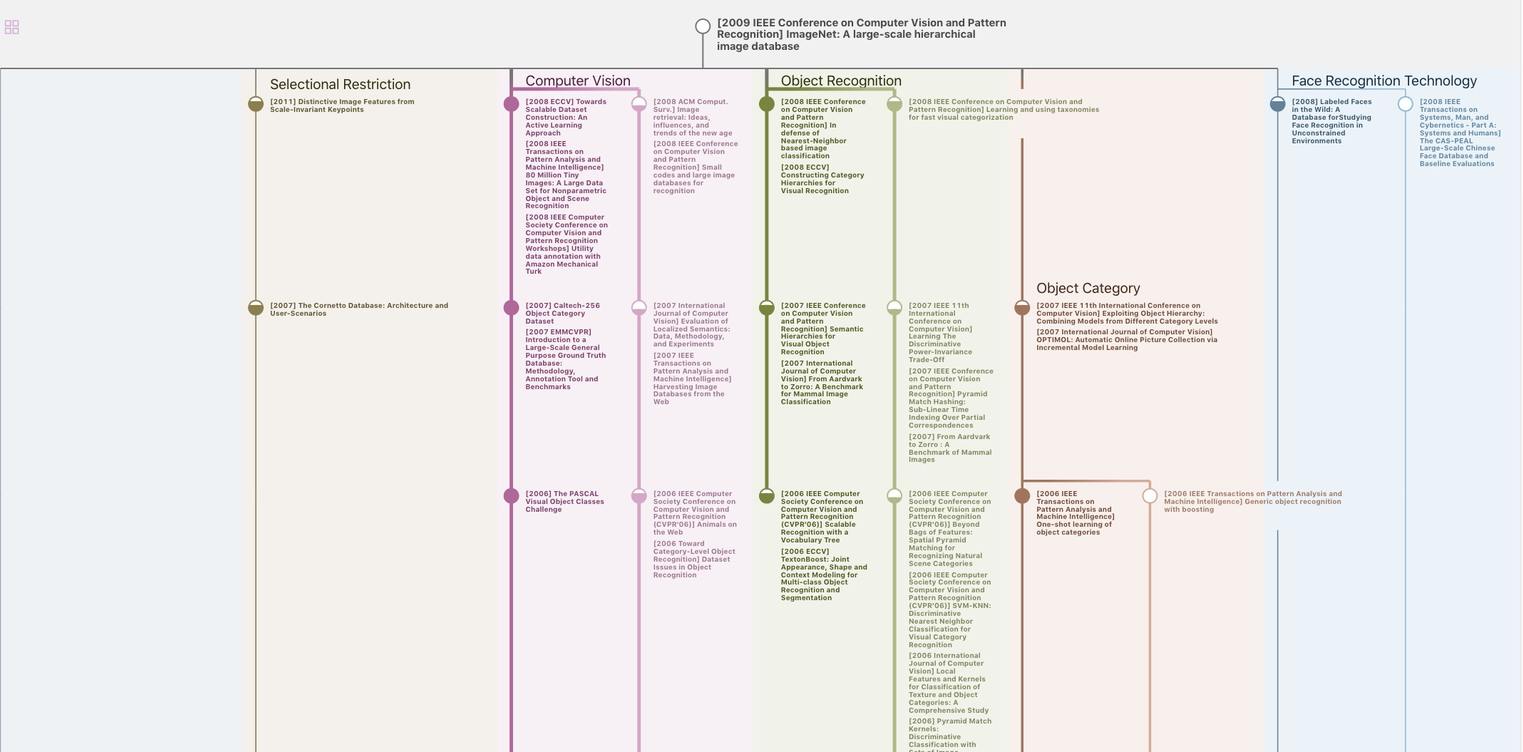
生成溯源树,研究论文发展脉络
Chat Paper
正在生成论文摘要