Computed Tomography Image Enhancement Using 3d Convolutional Neural Network
DEEP LEARNING IN MEDICAL IMAGE ANALYSIS AND MULTIMODAL LEARNING FOR CLINICAL DECISION SUPPORT, DLMIA 2018(2018)
摘要
Computed tomography (CT) is increasingly being used for cancer screening, such as early detection of lung cancer. However, CT studies have varying pixel spacing due to differences in acquisition parameters. Thick slice CTs have lower resolution, hindering tasks such as nodule characterization during computer-aided detection due to partial volume effect. In this study, we propose a novel 3D enhancement convolutional neural network (3DECNN) to improve the spatial resolution of CT studies that were acquired using lower resolution/slice thicknesses to higher resolutions. Using a subset of the LIDC dataset consisting of 20,672 CT slices from 100 scans, we simulated lower resolution/thick section scans then attempted to reconstruct the original images using our 3DECNN network. A significant improvement in PSNR (29.3087dB vs. 28.8769dB, p-value < 2.2e - 16) and SSIM (0.8529dB vs. 0.8449dB, p-value < 2.2e - 16) compared to other state-of-art deep learning methods is observed.
更多查看译文
关键词
Super resolution, Computed tomography, Medical imaging, Convolutional neural network, Image enhancement, Deep learning
AI 理解论文
溯源树
样例
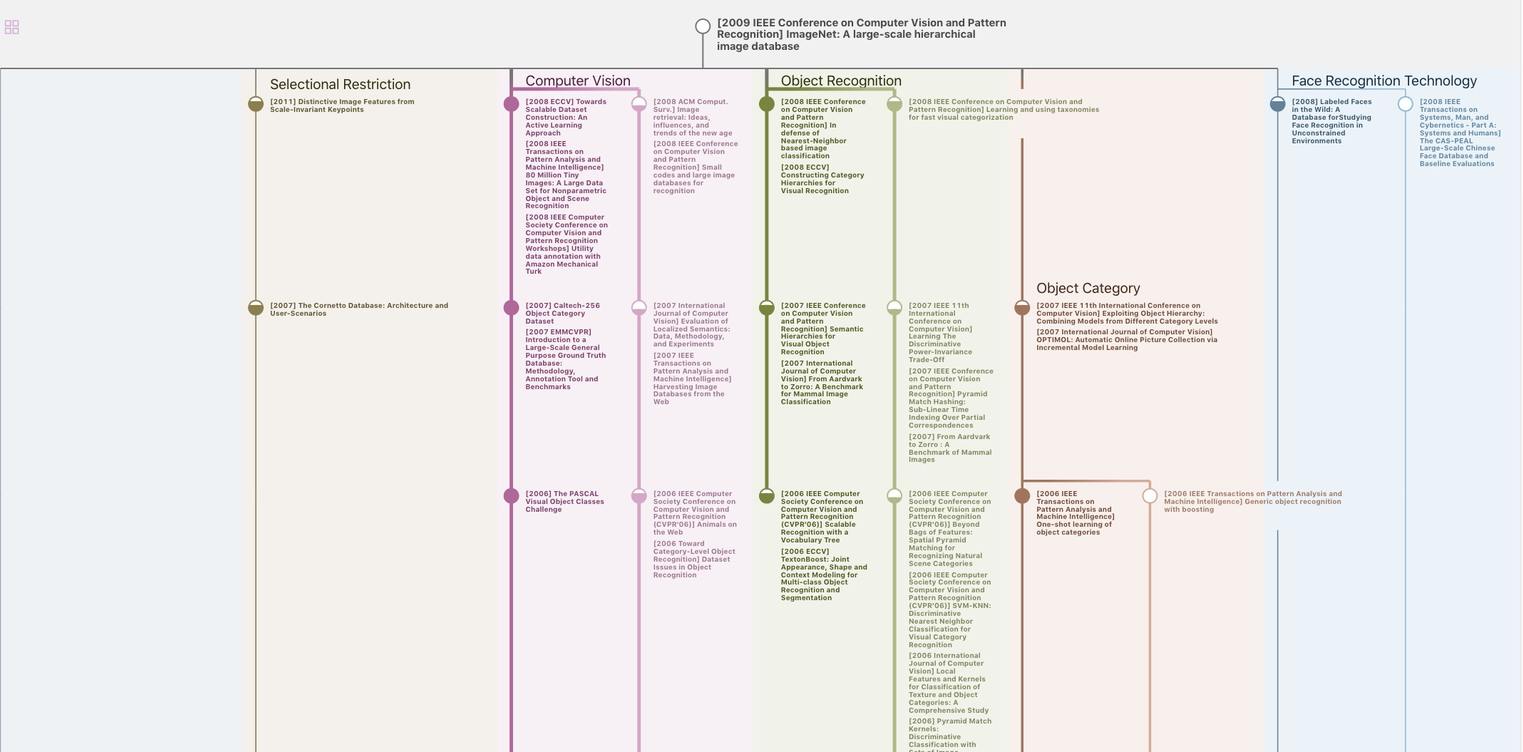
生成溯源树,研究论文发展脉络
Chat Paper
正在生成论文摘要