Dynamic Spectrum Matching With One-Shot Learning
CHEMOMETRICS AND INTELLIGENT LABORATORY SYSTEMS(2019)
摘要
Convolutional neural networks (CNN) have been shown to provide a good solution for classification problems that utilize data obtained from vibrational spectroscopy. Moreover, CNNs are capable of identifying substances from noisy spectra without the need for additional preprocessing. However, their application in practical spectroscopy is restricted due to two reasons. First the effectiveness of classification using CNNs diminishes rapidly when only a small number of spectra per substance are available for training (which is a typical situation in real applications). Secondly, to accommodate new, previously unseen, substance classes the network must be retrained which is computationally intensive. Here we address these issues by reformulating a multi-class classification problem with a large number of classes to a binary classification problem for which the available data is sufficient for representation learning. Hence, we define the learning task as identifying pairs of inputs as belonging to the same class or different classes. We achieve this using a Siamese convolutional neural network. A novel sampling strategy is proposed to address the imbalance problem in training the Siamese network. The trained network can classify samples of previously unseen substance classes using just a single reference sample (termed as one-shot learning in the machine learning community). Our results on three independent Raman datasets demonstrate much better accuracy than other practical systems to date, while allowing effortless updates of the system's database with new substance classes.
更多查看译文
关键词
Spectrum matching,Siamese network,One-shot learning,Convolutional neural networks
AI 理解论文
溯源树
样例
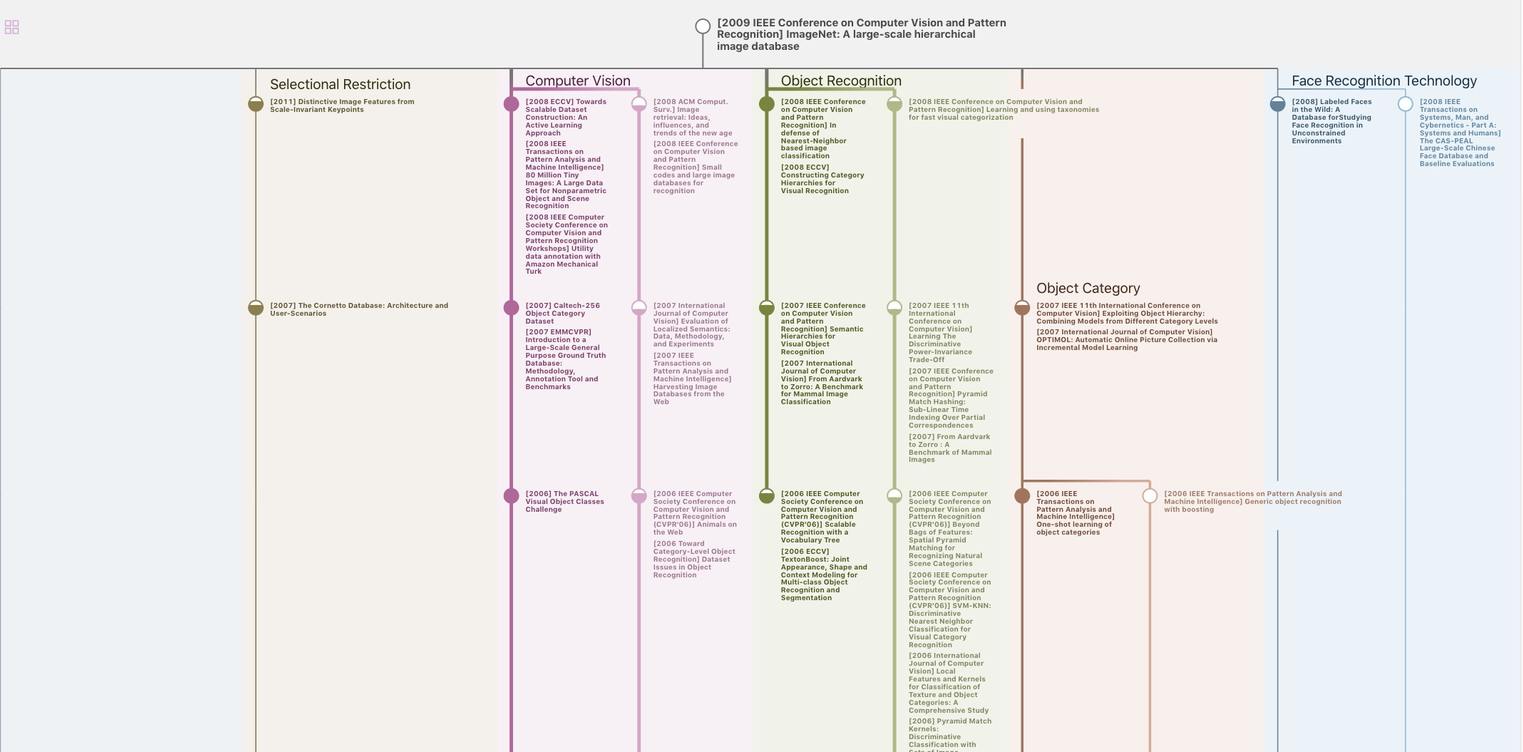
生成溯源树,研究论文发展脉络
Chat Paper
正在生成论文摘要