Mimic and Classify : A meta-algorithm for Conditional Independence Testing.
arXiv: Machine Learning(2018)
摘要
Given independent samples generated from the joint distribution $p(mathbf{x},mathbf{y},mathbf{z})$, we study the problem of Conditional Independence (CI-Testing), i.e., whether the joint equals the CI distribution $p^{CI}(mathbf{x},mathbf{y},mathbf{z})= p(mathbf{z}) p(mathbf{y}|mathbf{z})p(mathbf{x}|mathbf{z})$ or not. We cast this problem under the purview of the proposed, provable meta-algorithm, and Classify, which is realized in two-steps: (a) Mimic the CI distribution close enough to recover the support, and (b) Classify to distinguish the joint and the CI distribution. Thus, as long as we have a good generative model and a good classifier, we potentially have a sound CI Tester. With this modular paradigm, CI Testing becomes amiable to be handled by state-of-the-art, both generative and classification methods from the modern advances in Deep Learning, which in general can handle issues related to curse of dimensionality and operation in small sample regime. We show intensive numerical experiments on synthetic and real datasets where new mimic methods such conditional GANs, Regression with Neural Nets, outperform the current best CI Testing performance in the literature. Our theoretical results provide analysis on the estimation of null distribution as well as allow for general measures, i.e., when either some of the random variables are discrete and some are continuous or when one or more of them are discrete-continuous mixtures.
更多查看译文
AI 理解论文
溯源树
样例
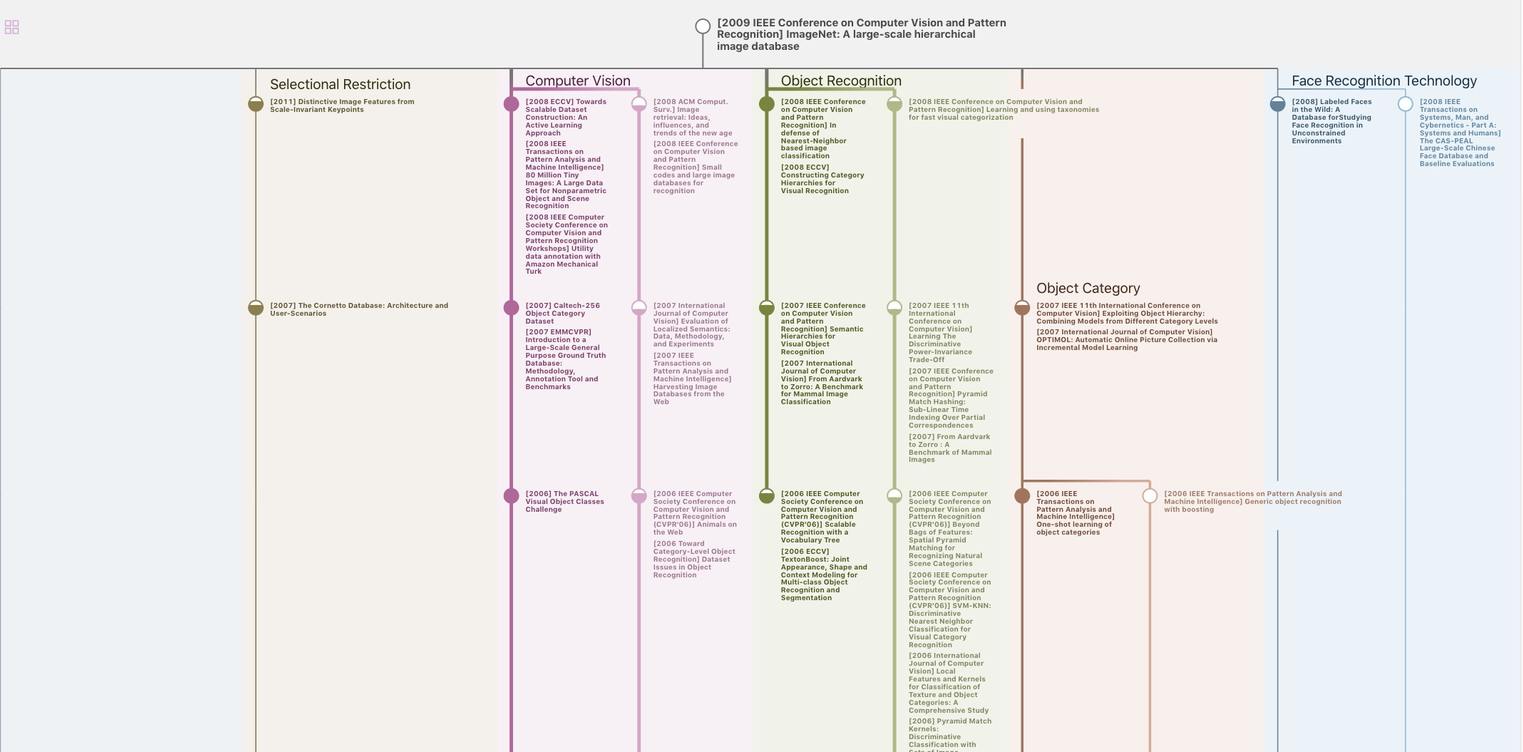
生成溯源树,研究论文发展脉络
Chat Paper
正在生成论文摘要