Run Procrustes, Run! On the convergence of accelerated Procrustes Flow.
arXiv: Learning(2018)
摘要
In this work, we present theoretical results on the convergence of non-convex accelerated gradient descent matrix factorization models. The technique is applied to matrix sensing problems with squared loss, for the estimation of a rank $r$ optimal solution $X^star in mathbb{R}^{n times n}$. We show that the acceleration leads to linear convergence rate, even under non-convex settings where the variable $X$ is represented as $U U^top$ for $U in mathbb{R}^{n times r}$. Our result has the same dependence on the condition number of the objective --and the optimal solution-- as that of the recent results on non-accelerated algorithms. However, acceleration is observed practice, both synthetic examples and two real applications: neuronal multi-unit activities recovery from single electrode recordings, and quantum state tomography on quantum computing simulators.
更多查看译文
关键词
accelerated procrustes flow,convergence
AI 理解论文
溯源树
样例
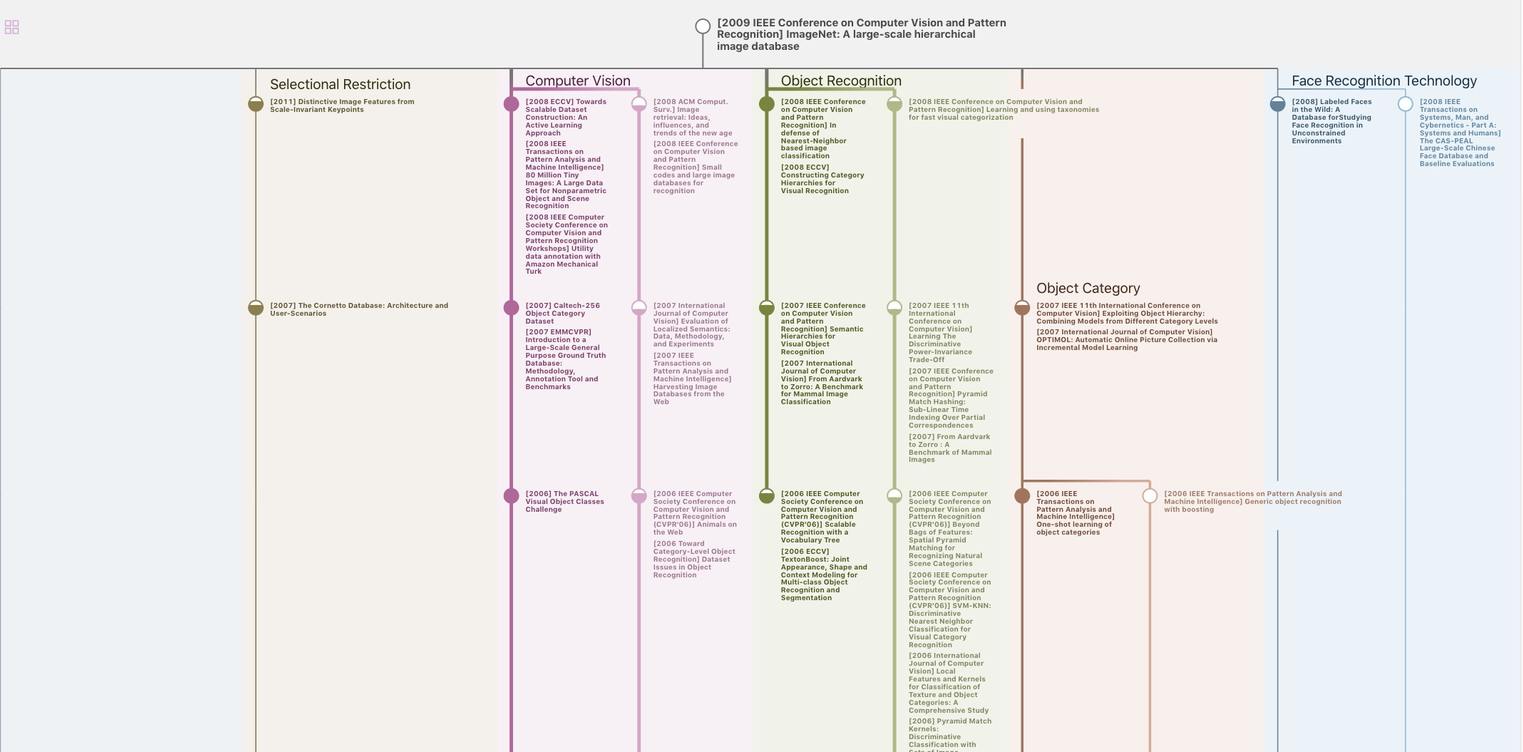
生成溯源树,研究论文发展脉络
Chat Paper
正在生成论文摘要