Learning to Search in Long Documents Using Document Structure
COLING(2018)
摘要
Reading comprehension models are based on recurrent neural networks that sequentially process the document tokens. As interest turns to answering more complex questions over longer documents, sequential reading of large portions of text becomes a substantial bottleneck. Inspired by how humans use document structure, we propose a novel framework for reading comprehension. We represent documents as trees, and model an agent that learns to interleave quick navigation through the document tree with more expensive answer extraction. To encourage exploration of the document tree, we propose a new algorithm, based on Deep Q-Network (DQN), which strategically samples tree nodes at training time. Empirically we find our algorithm improves question answering performance compared to DQN and a strong information-retrieval (IR) baseline, and that ensembling our model with the IR baseline results in further gains in performance.
更多查看译文
关键词
long documents,search,structure,learning
AI 理解论文
溯源树
样例
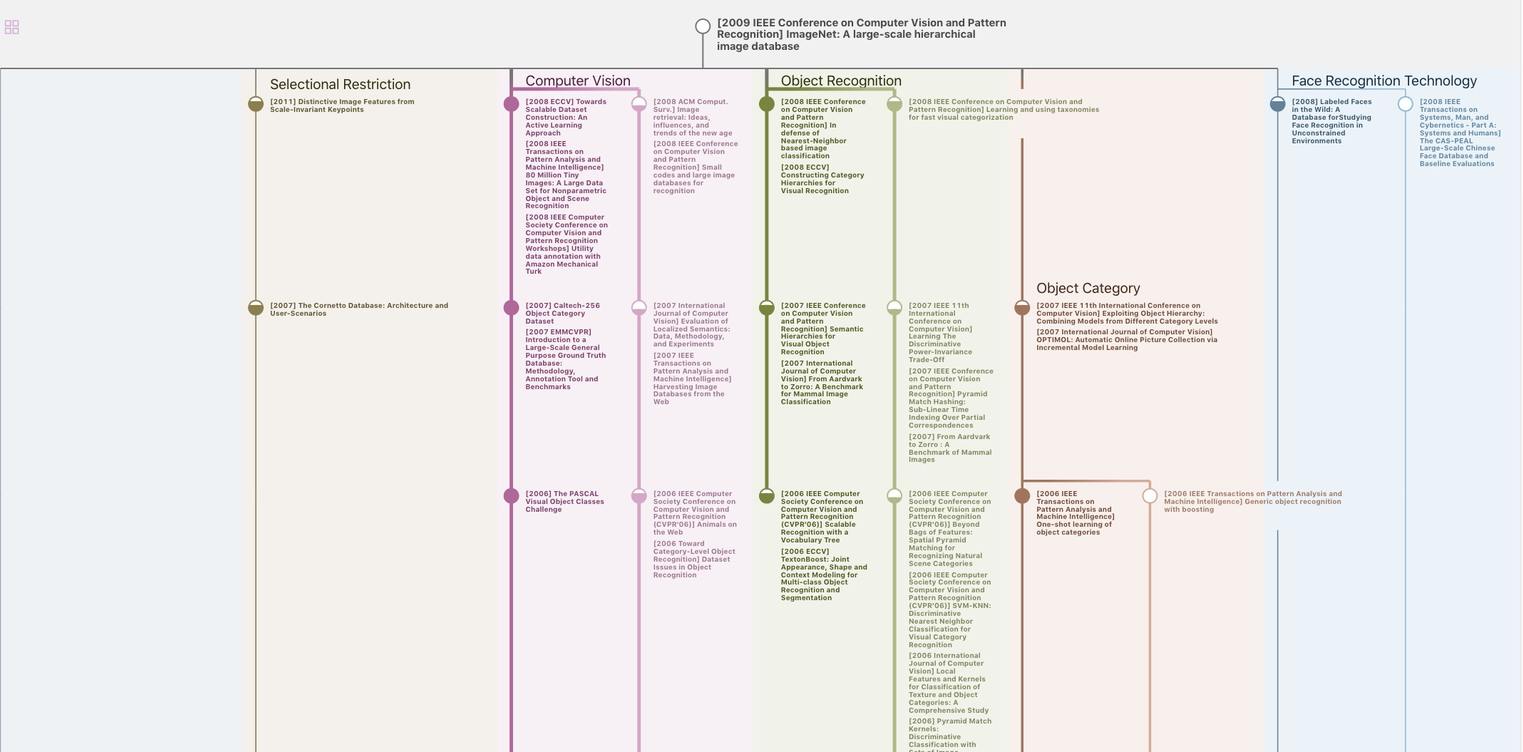
生成溯源树,研究论文发展脉络
Chat Paper
正在生成论文摘要