Instance Segmentation and Tracking with Cosine Embeddings and Recurrent Hourglass Networks
Medical Image Computing and Computer Assisted Intervention – MICCAI 2018: 21st International Conference, Granada, Spain, September 16-20, 2018, Proceedings, Part II(2018)
摘要
Different to semantic segmentation, instance segmentation assigns unique labels to each individual instance of the same class. In this work, we propose a novel recurrent fully convolutional network architecture for tracking such instance segmentations over time. The network architecture incorporates convolutional gated recurrent units (ConvGRU) into a stacked hourglass network to utilize temporal video information. Furthermore, we train the network with a novel embedding loss based on cosine similarities, such that the network predicts unique embeddings for every instance throughout videos. Afterwards, these embeddings are clustered among subsequent video frames to create the final tracked instance segmentations. We evaluate the recurrent hourglass network by segmenting left ventricles in MR videos of the heart, where it outperforms a network that does not incorporate video information. Furthermore, we show applicability of the cosine embedding loss for segmenting leaf instances on still images of plants. Finally, we evaluate the framework for instance segmentation and tracking on six datasets of the ISBI celltracking challenge, where it shows state-of-the-art performance.
更多查看译文
关键词
Cell,Tracking,Segmentation,Instances,Recurrent,Video,Embeddings
AI 理解论文
溯源树
样例
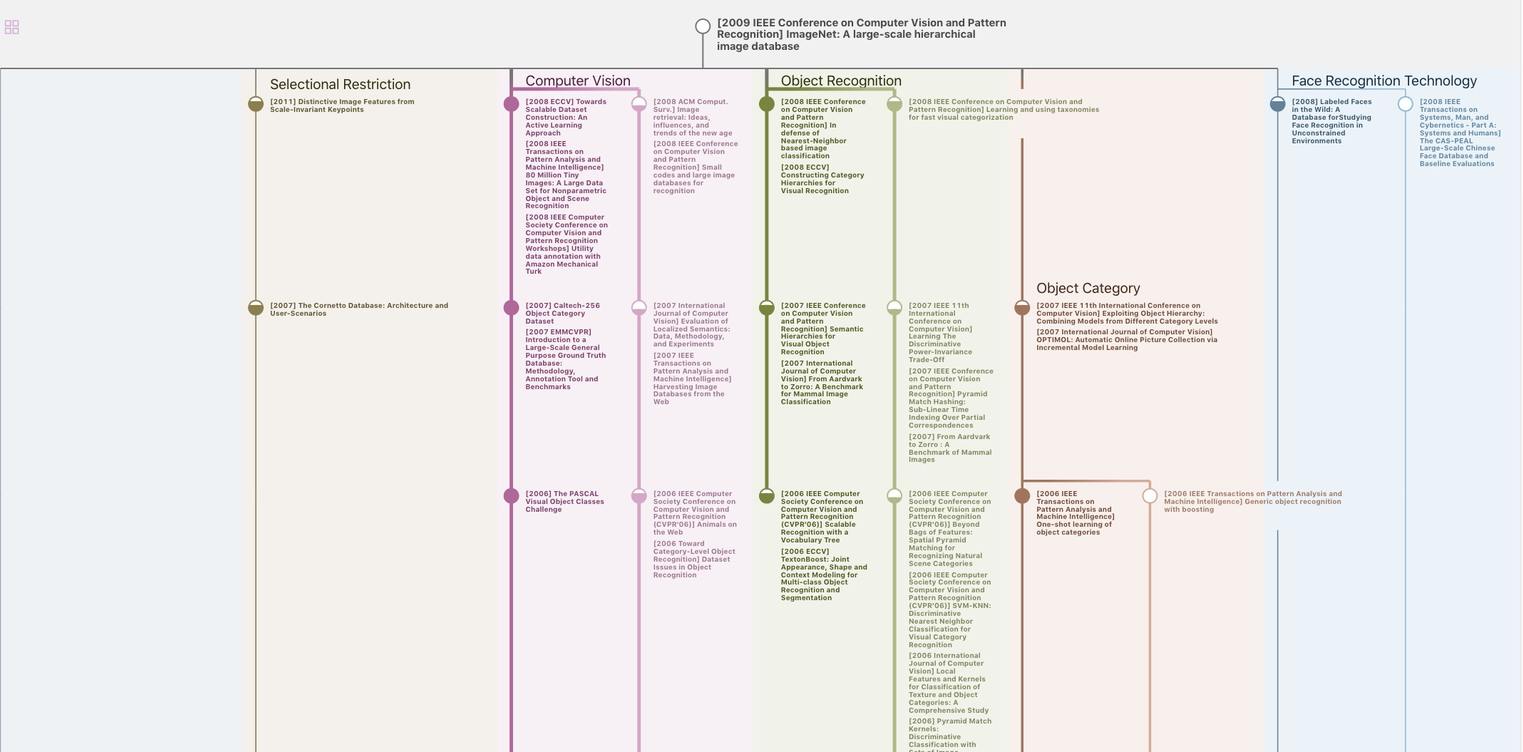
生成溯源树,研究论文发展脉络
Chat Paper
正在生成论文摘要