Boosting algorithms for uplift modeling.
arXiv: Learning(2018)
摘要
Uplift modeling is an area of machine learning which aims at predicting the causal effect of some action on a given individual. The action may be a medical procedure, marketing campaign, or any other circumstance controlled by the experimenter. Building an uplift model requires two training sets: the treatment group, where individuals have been subject to the action, and the control group, where no action has been performed. An uplift model allows then to assess the gain resulting from taking the action on a given individual, such as the increase in probability of patient recovery or of a product being purchased. This paper describes an adaptation of the well-known boosting techniques to the uplift modeling case. We formulate three desirable properties which an uplift boosting algorithm should have. Since all three properties cannot be satisfied simultaneously, we propose three uplift boosting algorithms, each satisfying two of them. Experiments demonstrate the usefulness of the proposed methods, which often dramatically improve performance of the base models and are thus new and powerful tools for uplift modeling.
更多查看译文
AI 理解论文
溯源树
样例
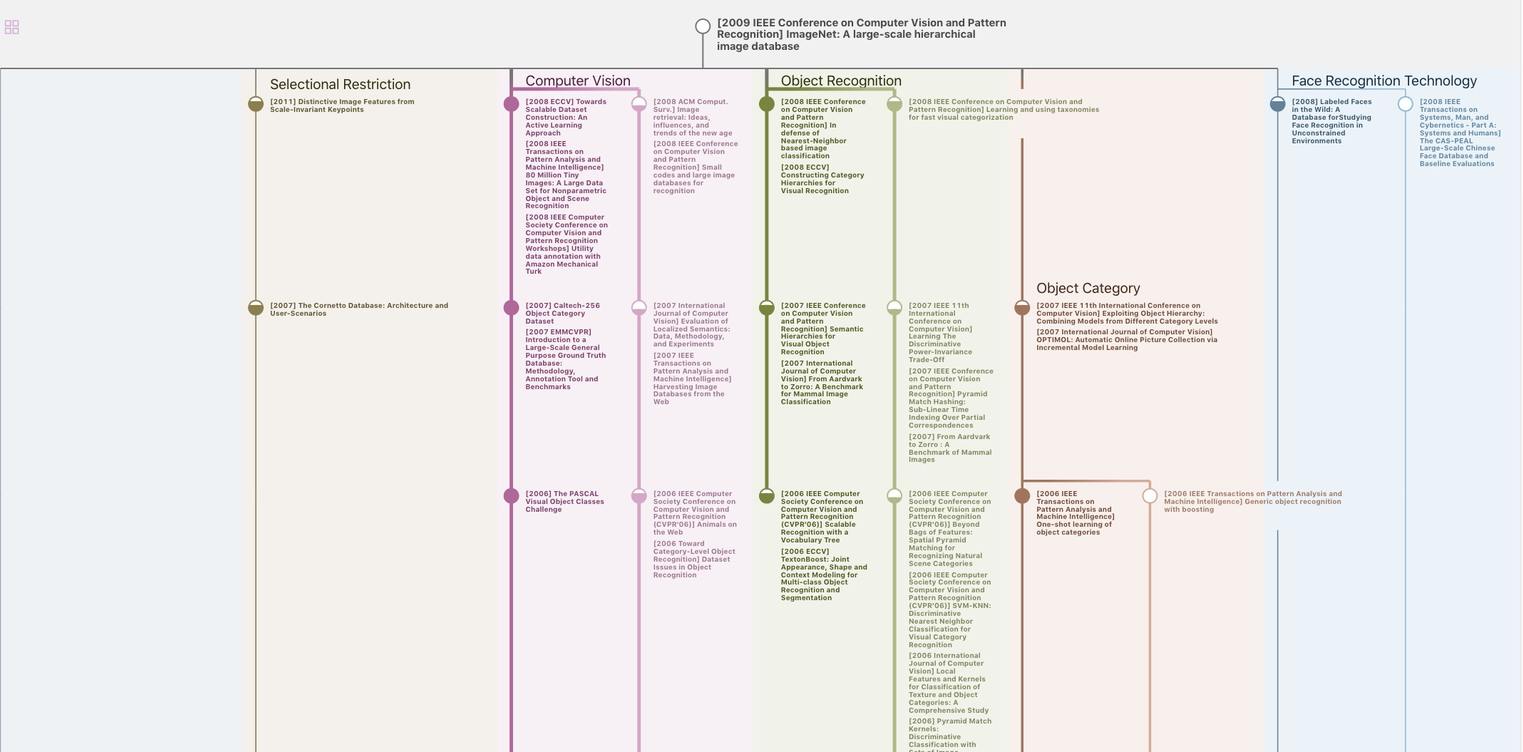
生成溯源树,研究论文发展脉络
Chat Paper
正在生成论文摘要