Efficient Probabilistic Inference In The Quest For Physics Beyond The Standard Model
arXiv: Learning(2019)
摘要
We present a novel probabilistic programming framework that couples directly to existing large-scale simulators through a cross-platform probabilistic execution protocol, which allows general-purpose inference engines to record and control random number draws within simulators in a language-agnostic way. The execution of existing simulators as probabilistic programs enables highly interpretable posterior inference in the structured model defined by the simulator code base. We demonstrate the technique in particle physics, on a scientifically accurate simulation of the tau (tau) lepton decay, which is a key ingredient in establishing the properties of the Higgs boson. Inference efficiency is achieved via inference compilation where a deep recurrent neural network is trained to parameterize proposal distributions and control the stochastic simulator in a sequential importance sampling scheme, at a fraction of the computational cost of a Markov chain Monte Carlo baseline.
更多查看译文
关键词
higgs boson,particle physics
AI 理解论文
溯源树
样例
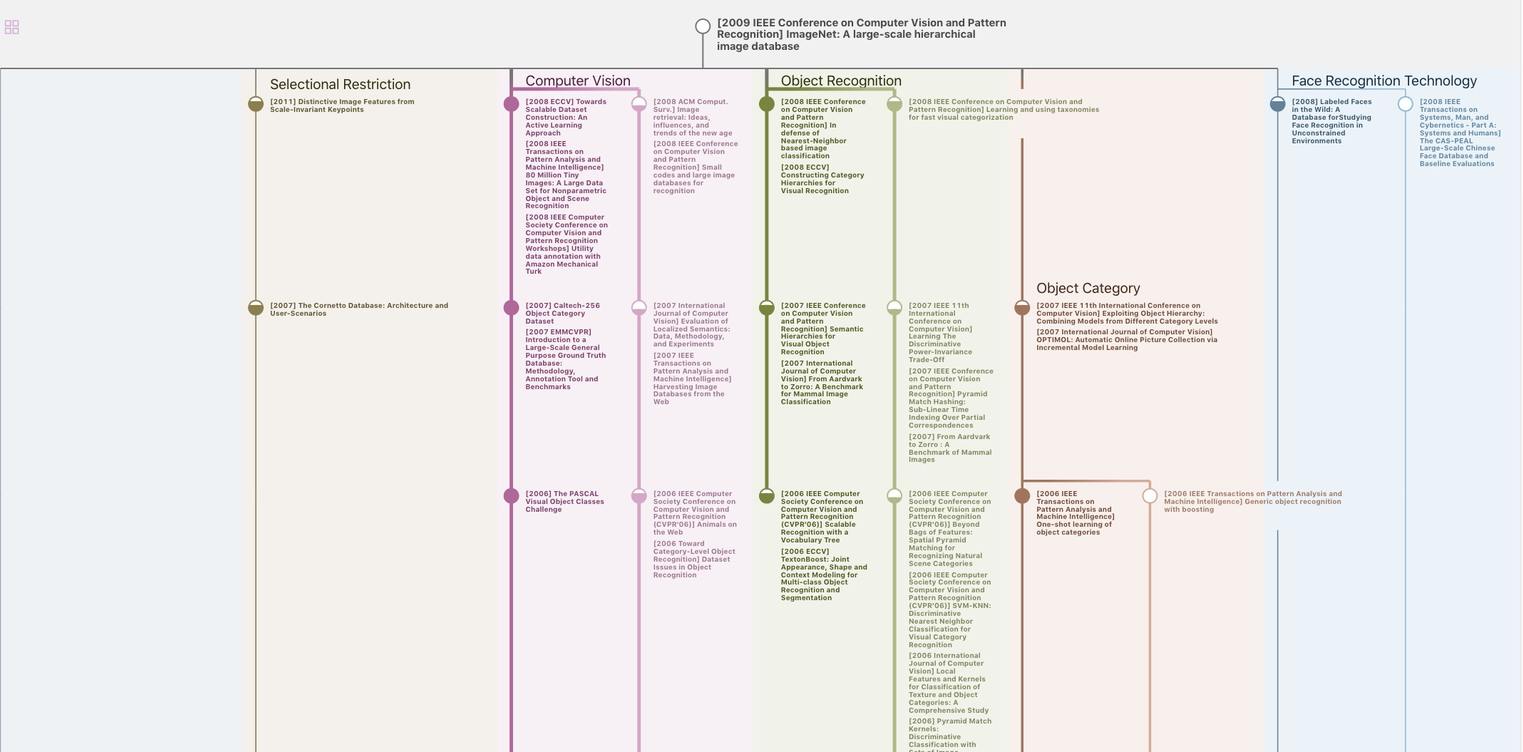
生成溯源树,研究论文发展脉络
Chat Paper
正在生成论文摘要