Towards a Unified Analysis of Random Fourier Features
JOURNAL OF MACHINE LEARNING RESEARCH(2019)
摘要
Random Fourier features is a widely used, simple, and effective technique for scaling up kernel methods. The existing theoretical analysis of the approach, however, remains focused on specific learning tasks and typically gives pessimistic bounds which are at odds with the empirical results. We tackle these problems and provide the first unified risk analysis of learning with random Fourier features using the squared error and Lipschitz continuous loss functions. In our bounds, the trade-off between the computational cost and the expected risk convergence rate is problem specific and expressed in terms of the regularization parameter and the number of effective degrees of freedom. We study both the standard random Fourier features method for which we improve the existing bounds on the number of features required to guarantee the corresponding minimax risk convergence rate of kernel ridge regression, as well as a data-dependent modification which samples features proportional to ridge leverage scores and further reduces the required number of features. As ridge leverage scores are expensive to compute, we devise a simple approximation scheme which provably reduces the computational cost without loss of statistical efficiency.
更多查看译文
关键词
Kernel methods, random Fourier features, stationary kernels, kernel ridge regression, Lipschitz continuous loss, support vector machines, logistic regression, ridge leverage scores
AI 理解论文
溯源树
样例
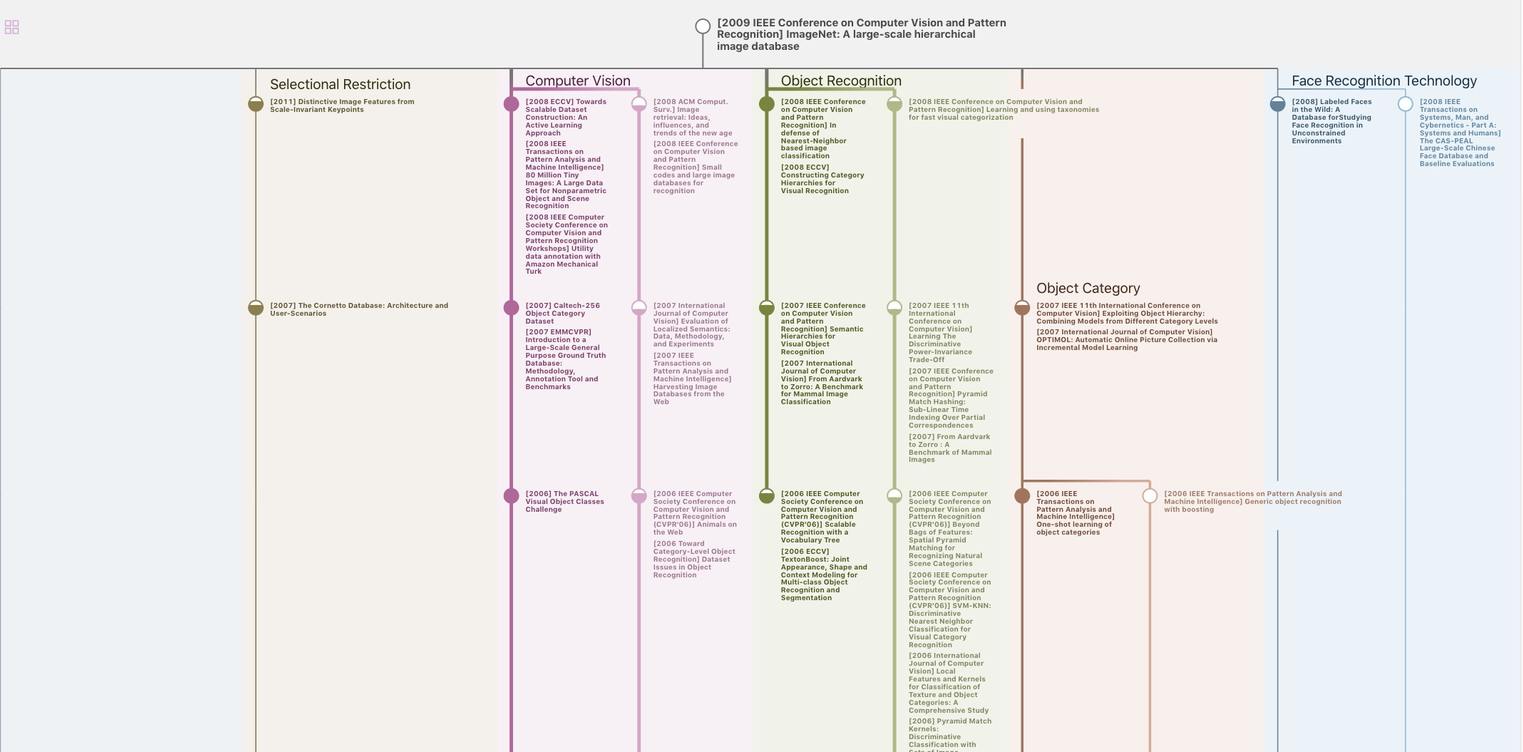
生成溯源树,研究论文发展脉络
Chat Paper
正在生成论文摘要