Synthesizing Diverse, High-Quality Audio Textures.
arXiv: Sound(2018)
摘要
Texture synthesis techniques based on matching the Gram matrix of feature activations in neural networks have achieved spectacular success in the image domain. In this paper we extend these techniques to the audio domain. We demonstrate that synthesizing diverse audio textures is challenging, and argue that this is because audio data is relatively low-dimensional. We therefore introduce two new terms to the original Grammian loss: an autocorrelation term that preserves rhythm, and a diversity term that encourages the optimization procedure to synthesize unique textures. We quantitatively study the impact of our design choices on the quality of the synthesized audio by introducing an audio analogue to the Inception loss which we term the VGGish loss. We show that there is a trade-off between the diversity and quality of the synthesized audio using this technique. We additionally perform a number of experiments to qualitatively study how these design choices impact the quality of the synthesized audio. Finally we describe the implications of these results for the problem of audio style transfer.
更多查看译文
关键词
high-quality high-quality,diverse
AI 理解论文
溯源树
样例
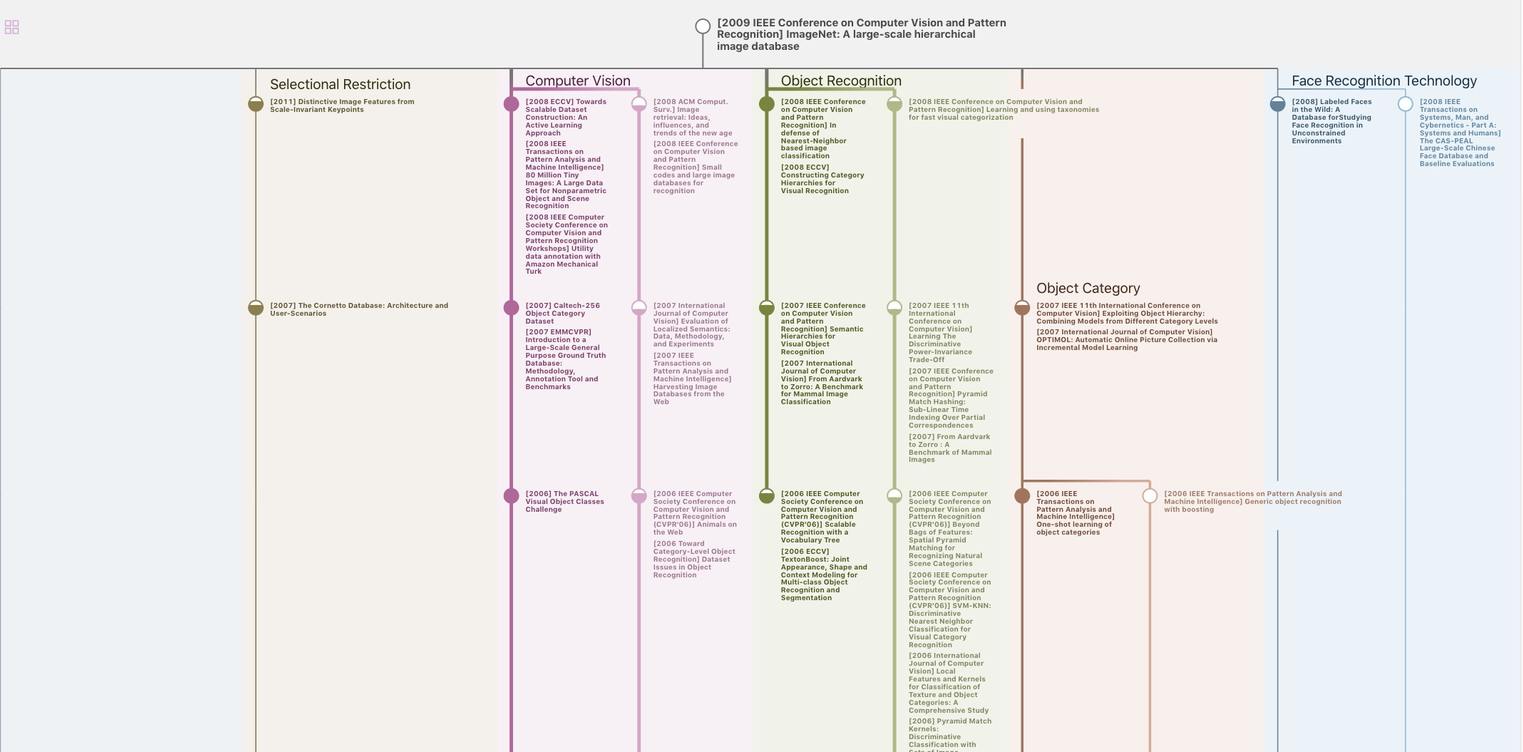
生成溯源树,研究论文发展脉络
Chat Paper
正在生成论文摘要