Combinatorial Bandits For Incentivizing Agents With Dynamic Preferences
UNCERTAINTY IN ARTIFICIAL INTELLIGENCE(2018)
摘要
The design of personalized incentives or recommendations to improve user engagement is gaining prominence as digital platform providers continually emerge. We propose a multi-armed bandit framework for matching incentives to users, whose preferences are unknown a priori and evolving dynamically in time, in a resource constrained environment. We design an algorithm that combines ideas from three distinct domains: (i) a greedy matching paradigm, (ii) the upper confidence bound algorithm (UCB) for bandits, and (iii) mixing times from the theory of Markov chains. For this algorithm, we provide theoretical bounds on the regret and demonstrate its performance via both synthetic and realistic (matching supply and demand in a bike-sharing platform) examples.
更多查看译文
关键词
incentivizing agents,dynamic preferences
AI 理解论文
溯源树
样例
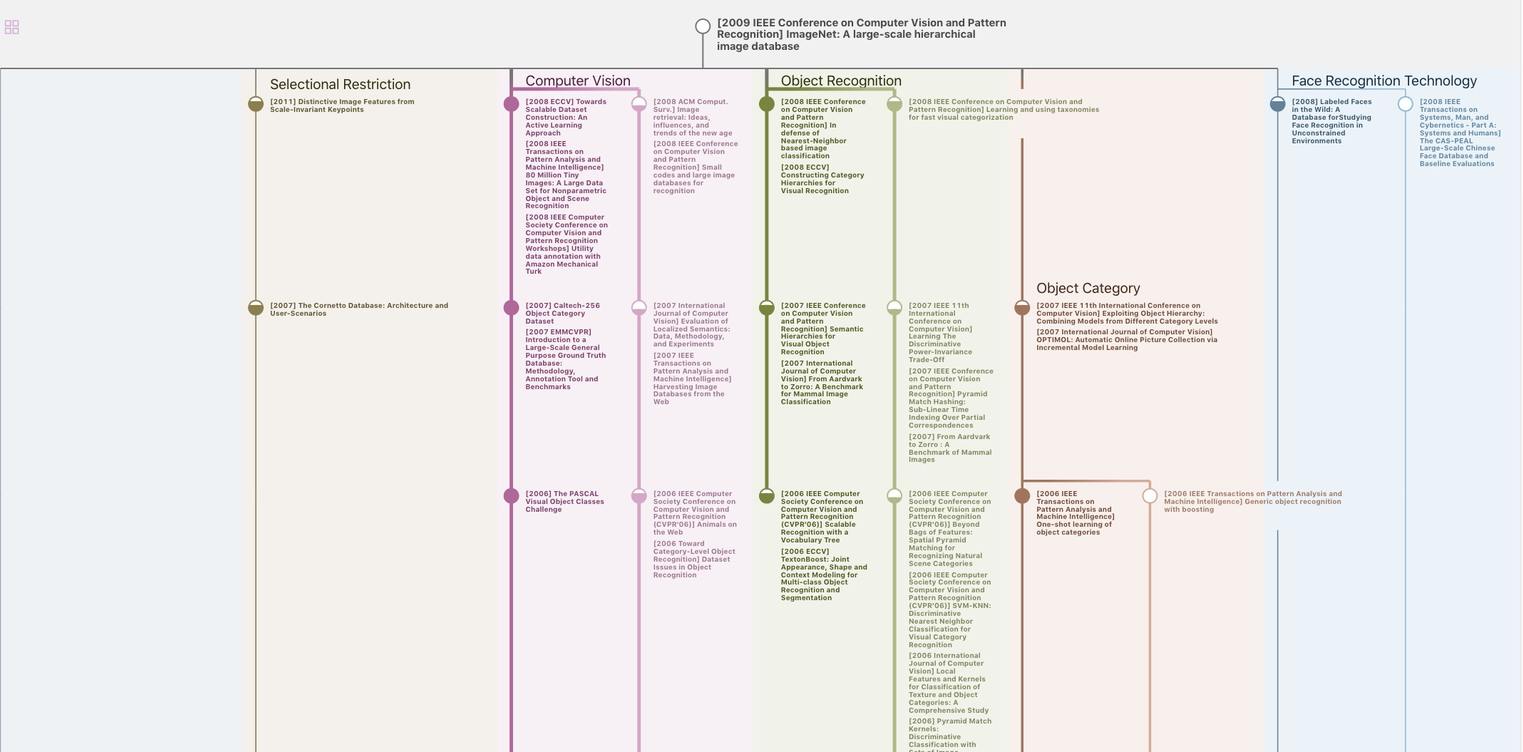
生成溯源树,研究论文发展脉络
Chat Paper
正在生成论文摘要