Hidden Markov random field model and Broyden-Fletcher-Goldfarb-Shanno algorithm for brain image segmentation.
JOURNAL OF EXPERIMENTAL & THEORETICAL ARTIFICIAL INTELLIGENCE(2018)
摘要
Many routine medical examinations produce images of patients suffering from various pathologies. With the huge number of medical images, the manual analysis and interpretation became a tedious task. Thus, automatic image segmentation became essential for diagnosis assistance. Segmentation consists in dividing the image into homogeneous and significant regions. We focus on hidden Markov random fields referred to as HMRF to model the problem of segmentation. This modelisation leads to a classical function minimisation problem. Broyden-Fletcher-Goldfarb-Shanno algorithm referred to as BFGS is one of the most powerful methods to solve unconstrained optimisation problem. In this paper, we investigate the combination of HMRF and BFGS algorithm to perform the segmentation operation. The proposed method shows very good segmentation results comparing with well-known approaches. The tests are conducted on brain magnetic resonance image databases (BrainWeb and IBSR) largely used to objectively confront the results obtained. The well-known Dice coefficient (DC) was used as similarity metric. The experimental results show that, in many cases, our proposed method approaches the perfect segmentation with a Dice Coefficient above .9. Moreover, it generally outperforms other methods in the tests conducted.
更多查看译文
关键词
Brain image segmentation,hidden Markov random field,Broyden-Fletcher-Goldfarb-Shanno algorithm,Dice coefficient criterion
AI 理解论文
溯源树
样例
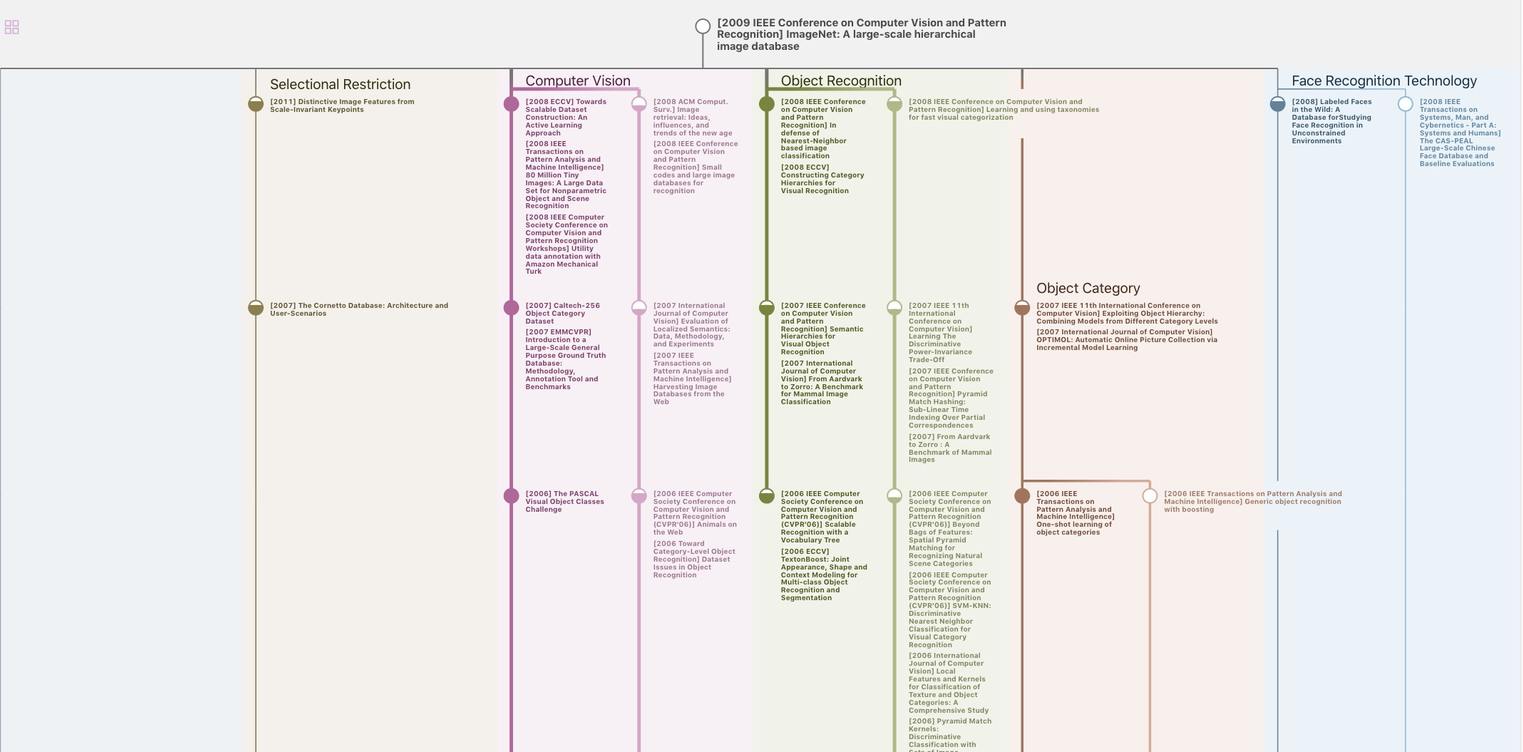
生成溯源树,研究论文发展脉络
Chat Paper
正在生成论文摘要