Chemical-induced disease extraction via recurrent piecewise convolutional neural networks
BMC Med. Inf. & Decision Making(2018)
摘要
Background Extracting relationships between chemicals and diseases from unstructured literature have attracted plenty of attention since the relationships are very useful for a large number of biomedical applications such as drug repositioning and pharmacovigilance. A number of machine learning methods have been proposed for chemical-induced disease (CID) extraction due to some publicly available annotated corpora. Most of them suffer from time-consuming feature engineering except deep learning methods. In this paper, we propose a novel document-level deep learning method, called recurrent piecewise convolutional neural networks (RPCNN), for CID extraction. Results Experimental results on a benchmark dataset, the CDR (Chemical-induced Disease Relation) dataset of the BioCreative V challenge for CID extraction show that the highest precision, recall and F-score of our RPCNN-based CID extraction system are 65.24, 77.21 and 70.77%, which is competitive with other state-of-the-art systems. Conclusions A novel deep learning method is proposed for document-level CID extraction, where domain knowledge, piecewise strategy, attention mechanism, and multi-instance learning are combined together. The effectiveness of the method is proved by experiments conducted on a benchmark dataset.
更多查看译文
关键词
Chemical-induced disease,Convolutional neural network,Deep learning,Relation extraction
AI 理解论文
溯源树
样例
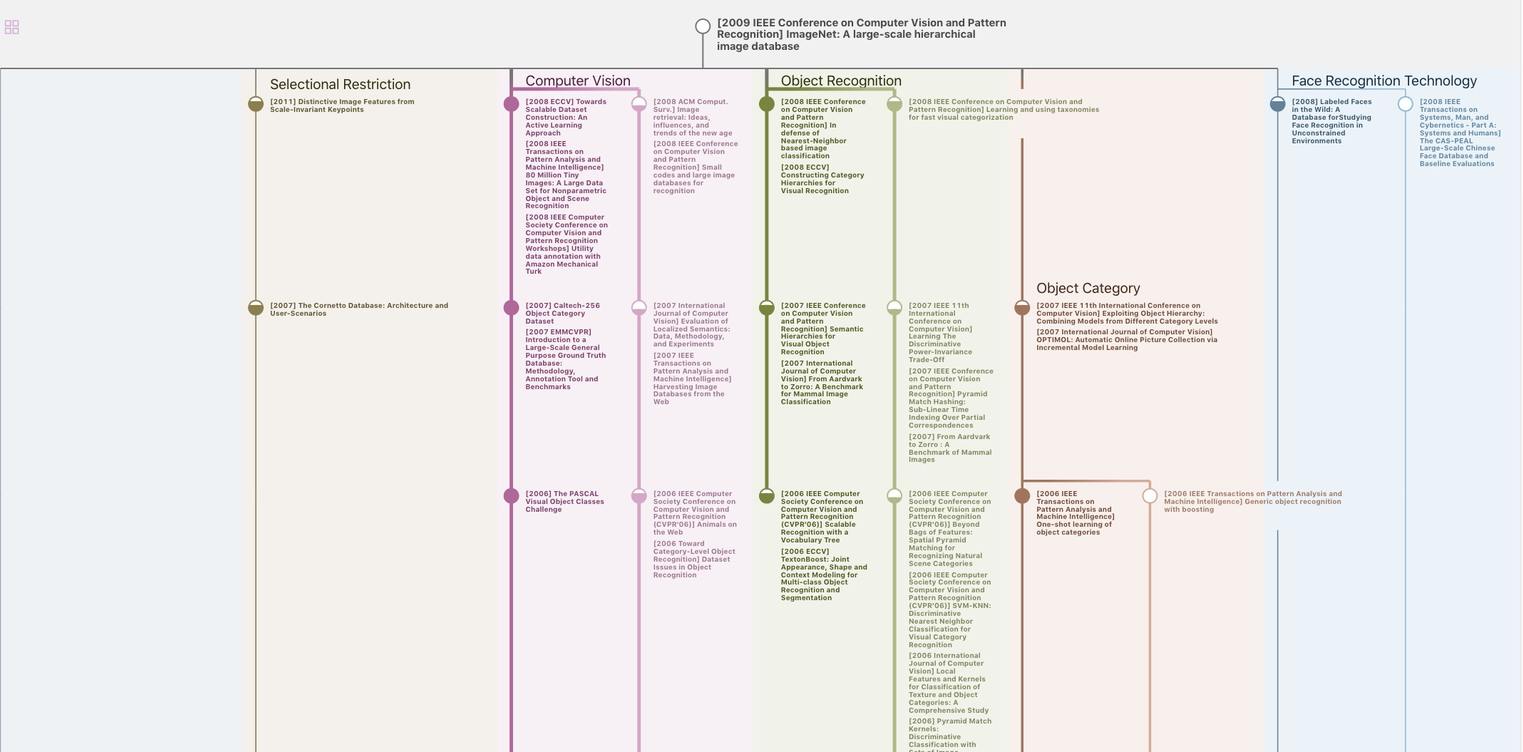
生成溯源树,研究论文发展脉络
Chat Paper
正在生成论文摘要