A novel ensemble decision tree based on under-sampling and clonal selection for web spam detection
Pattern Anal. Appl.(2017)
摘要
Currently, web spamming is a serious problem for search engines. It not only degrades the quality of search results by intentionally boosting undesirable web pages to users, but also causes the search engine to waste a significant amount of computational and storage resources in manipulating useless information. In this paper, we present a novel ensemble classifier for web spam detection which combines the clonal selection algorithm for feature selection and under-sampling for data balancing. This web spam detection system is called USCS. The USCS ensemble classifiers can automatically sample and select sub-classifiers. First, the system will convert the imbalanced training dataset into several balanced datasets using the under-sampling method. Second, the system will automatically select several optimal feature subsets for each sub-classifier using a customized clonal selection algorithm. Third, the system will build several C4.5 decision tree sub-classifiers from these balanced datasets based on its specified features. Finally, these sub-classifiers will be used to construct an ensemble decision tree classifier which will be applied to classify the examples in the testing data. Experiments on WEBSPAM-UK2006 dataset on the web spam problem show that our proposed approach, the USCS ensemble web spam classifier, contributes significant classification performance compared to several baseline systems and state-of-the-art approaches.
更多查看译文
关键词
Web spam detection, Ensemble learning, Clonal selection algorithm, Feature selection, Decision trees
AI 理解论文
溯源树
样例
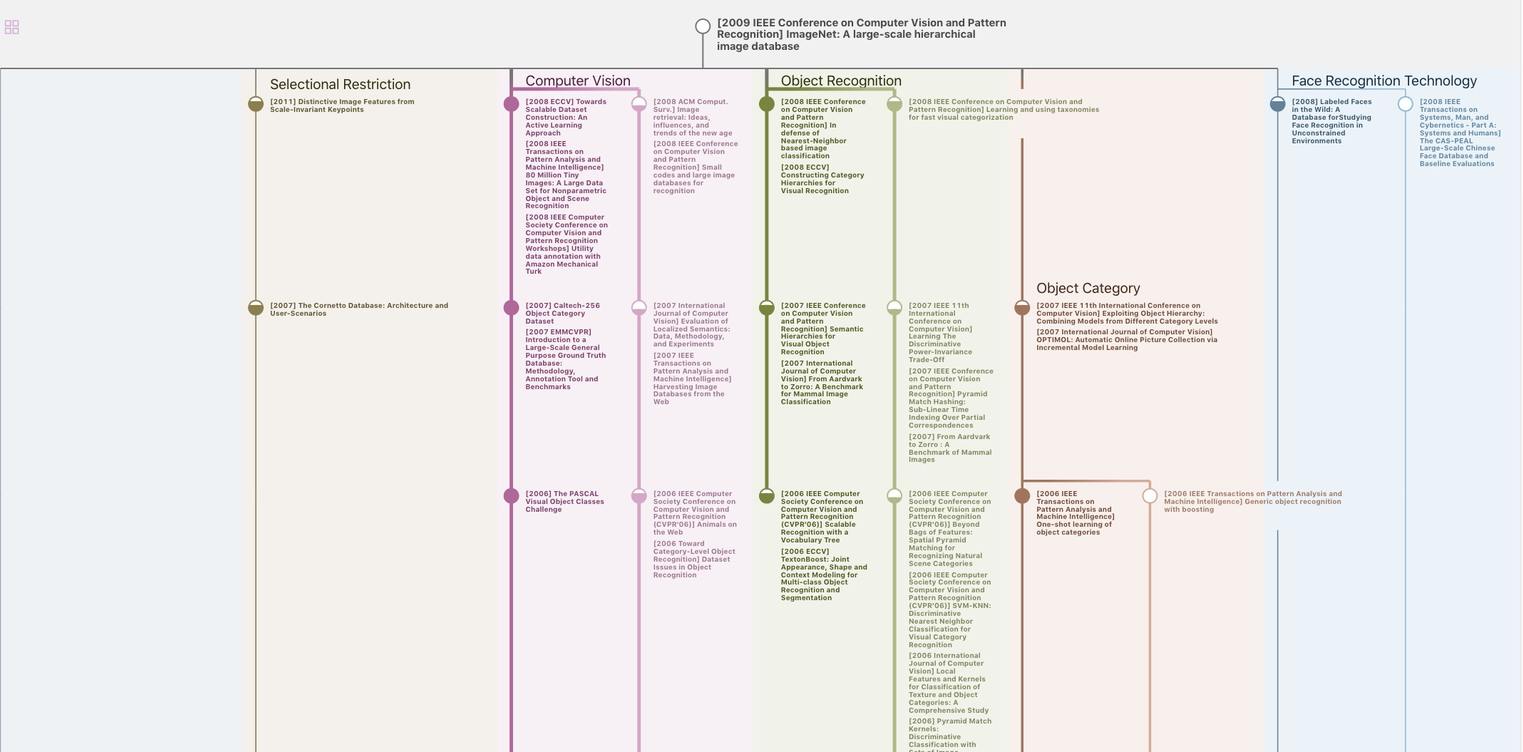
生成溯源树,研究论文发展脉络
Chat Paper
正在生成论文摘要