Efficient D2D content caching using multi-agent reinforcement learning
IEEE INFOCOM 2018 - IEEE CONFERENCE ON COMPUTER COMMUNICATIONS WORKSHOPS (INFOCOM WKSHPS)(2018)
摘要
To address the increase of multimedia traffic dominated by streaming videos, user equipment (UE) can collaboratively cache and share contents to alleviate the burden of base-stations. Since content popularity is regional specific and does not have a stationary distribution, a machine learning based caching strategy that exploits the knowledge of content demand history would be highly promising. In this paper, we design D2D caching strategies using multi-agent reinforcement learning (MARL) with non-perfect content popularity information. Specifically, we model the D2D caching problem as a multi-agent multi-armed bandit problem. Since the joint action space is too large to use traditional MARL methods, a belief-based modified combinatorial upper confidence bound (MCUCB) algorithm is proposed to technically solve the problem. Simulation results show that the belief-based MCUCB caching scheme outperforms other popular caching schemes in terms of cache byte hit rate and average downloading latency.
更多查看译文
关键词
D2D caching strategies,machine learning based caching strategy,belief-based modified combinatorial upper confidence algorithm,MCUCB algorithm,cache byte hit rate,popular caching schemes,belief-based MCUCB caching scheme,multiagent multiarmed bandit problem,D2D caching problem,nonperfect content popularity information,content demand history,machine learning,base-stations,user equipment,multiagent reinforcement learning,Efficient D2D content caching
AI 理解论文
溯源树
样例
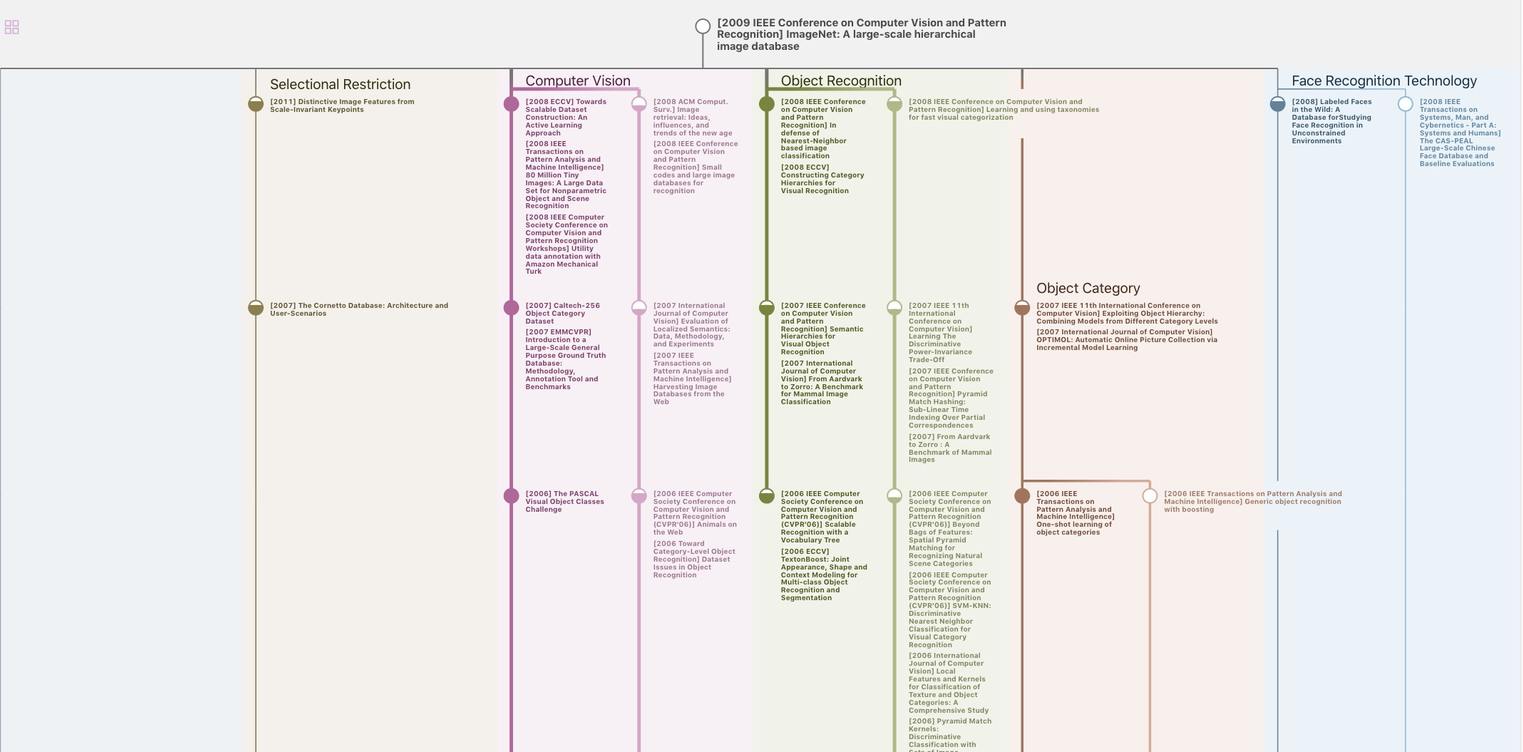
生成溯源树,研究论文发展脉络
Chat Paper
正在生成论文摘要