Traffic Speed Prediction with Neural Networks.
Operations Research Proceedings(2018)
摘要
With the increasing interest in creating Smart Cities, traffic speed prediction has attracted more attention in contemporary transportation research. Neural networks have been utilized in many studies to address this problem; yet, they have mainly focused on the short-term prediction while longer forecast horizons are needed for more reliable mobility and route planning. In this work we tackle the medium-term prediction as well as the short-term. We employ feedforward neural networks that combine time series forecasting techniques where the predicted speed values are fed into the network. We train our networks and select the hyper-parameters to minimize the mean absolute error. To test the performance of our method, we consider two multi-segment routes in Istanbul. The speed data are collected from floating cars for every minute over a 5-month horizon. Our computational results showed that accurate predictions can be achieved in medium-term horizon.
更多查看译文
关键词
Neural networks, Forecasting, Machine learning, Time series analysis, Exponential smoothing, Moving average, Traffic speed prediction
AI 理解论文
溯源树
样例
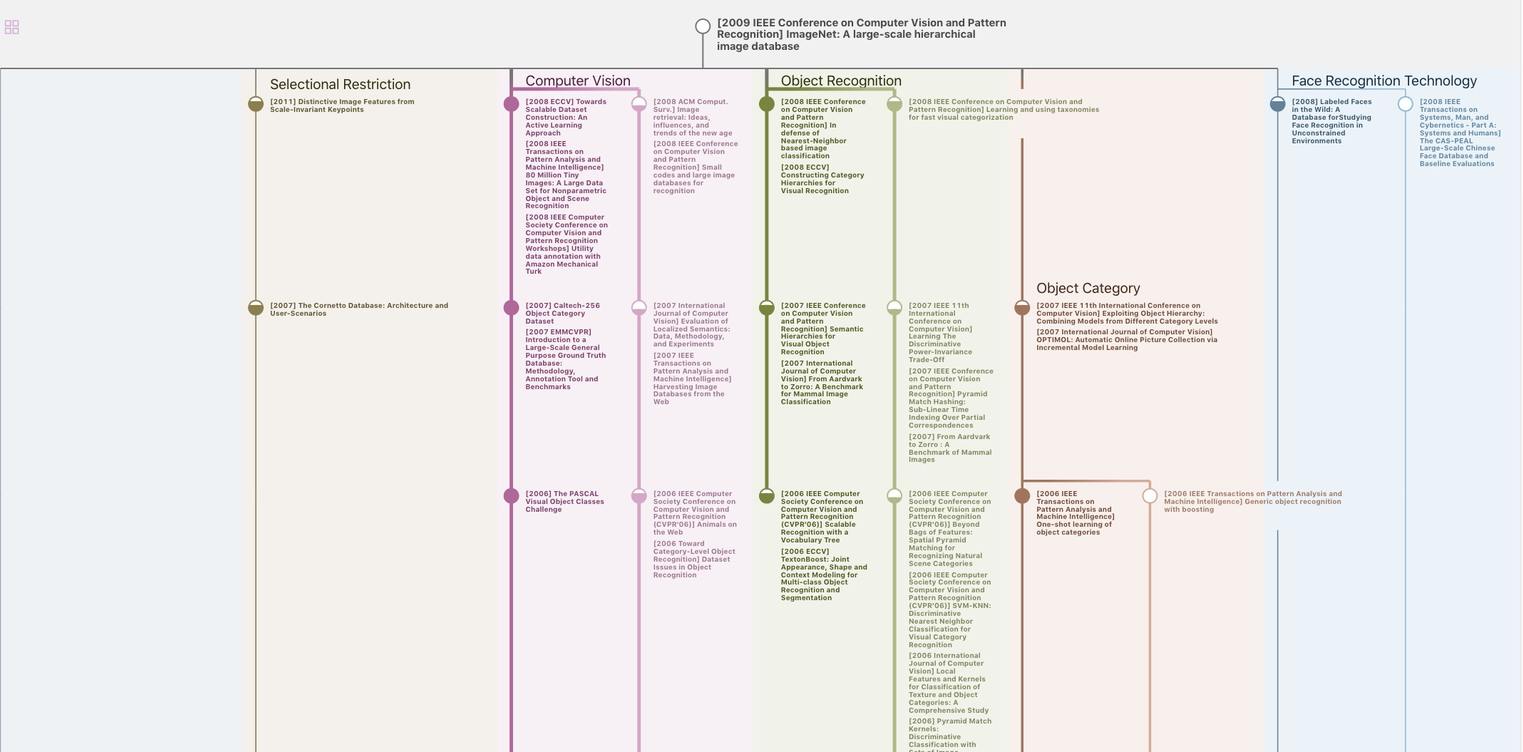
生成溯源树,研究论文发展脉络
Chat Paper
正在生成论文摘要