ATMoN: Adapting the "Temporality" in Large-Scale Dynamic Networks
2018 IEEE 38th International Conference on Distributed Computing Systems (ICDCS)(2018)
摘要
With the widespread adoption of temporal graphs to study fast evolving interactions in dynamic networks, attention is needed to provide graph metrics in time and at scale. In this paper, we introduce ATMoN, an open-source library developed to computationally offload graph processing engines and ease the communication overhead in dynamic networks over an unprecedented wealth of data. This is achieved, by efficiently adapting, in place and inexpensively, the temporal granularity at which graph metrics are computed based on runtime knowledge captured by a low-cost probabilistic learning model capable of approximating both the metric stream evolution and the volatility of the graph topology. After a thorough evaluation with real-world data from mobile, face-to-face and vehicular networks, results show that ATMoN is able to reduce the compute overhead by at least 76%, data volume by 60% and overall cloud costs by at least 54%, while always maintaining accuracy above 88%.
更多查看译文
关键词
Dynamic Networks,Edge Computing,Temporal Graphs,Adaptive Monitoring
AI 理解论文
溯源树
样例
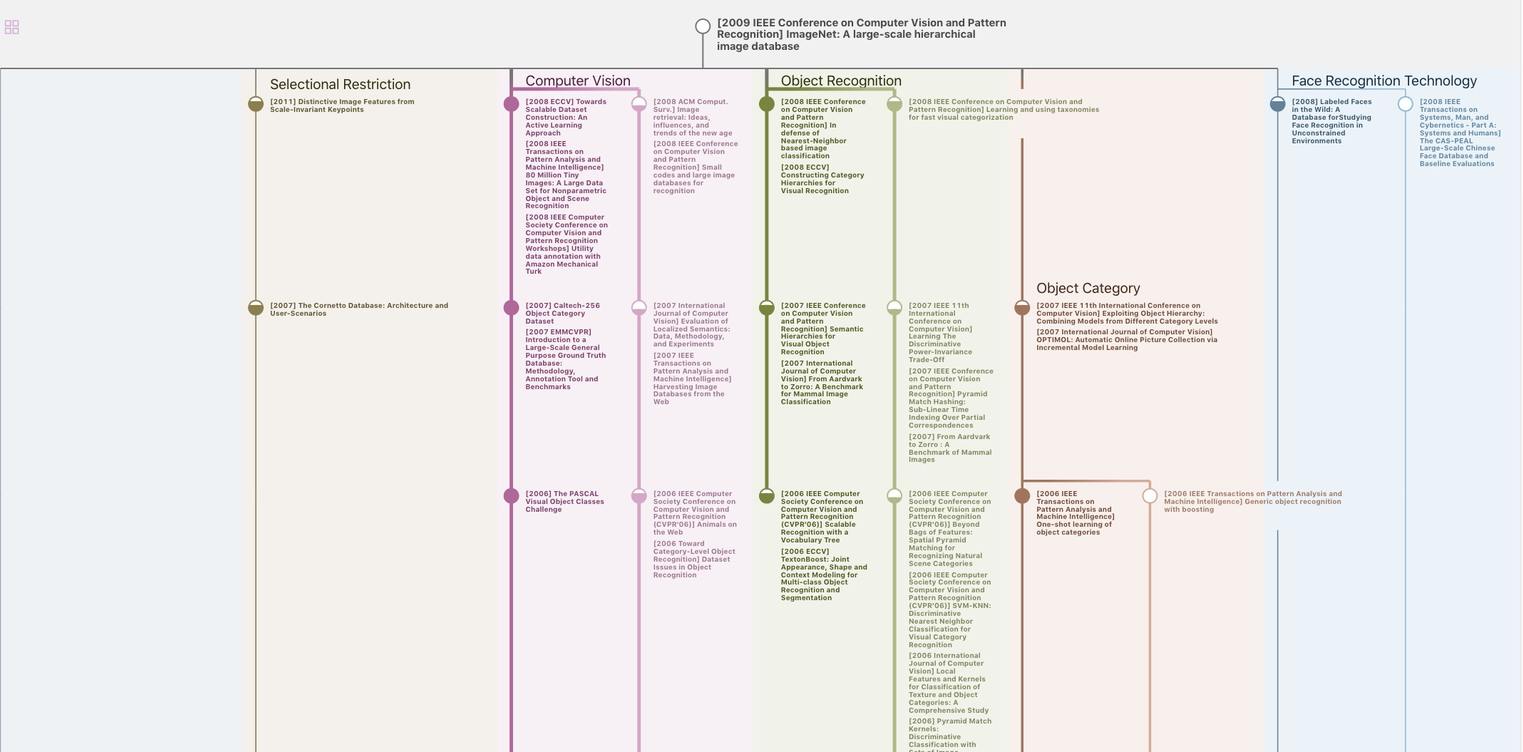
生成溯源树,研究论文发展脉络
Chat Paper
正在生成论文摘要