Zero Shot Transfer Learning For Robot Soccer
PROCEEDINGS OF THE 17TH INTERNATIONAL CONFERENCE ON AUTONOMOUS AGENTS AND MULTIAGENT SYSTEMS (AAMAS' 18)(2018)
摘要
We present a method for doing zero-shot transfer of multi-agent policies as the number of teammates, opponents, and environment size varies. We apply our approach to RoboCup inspired test domains, where it is necessary for policies to adapt to changing numbers of robots due to in-game breakages. We introduce the concept of encoding not only the states as an image, but also the action space as a multi-channel image, which allows the state and action size to remain fixed across team size changes. We also introduce Fully Convolutional Q-Networks, which represent Q-functions in this space using Fully Convolutional Networks. We present results for zero-shot transfer of these policies across team sizes and field sizes, showing that performance remains consistent as both change.
更多查看译文
关键词
Reinforcement Learning, Multiagent learning, Transfer Learning
AI 理解论文
溯源树
样例
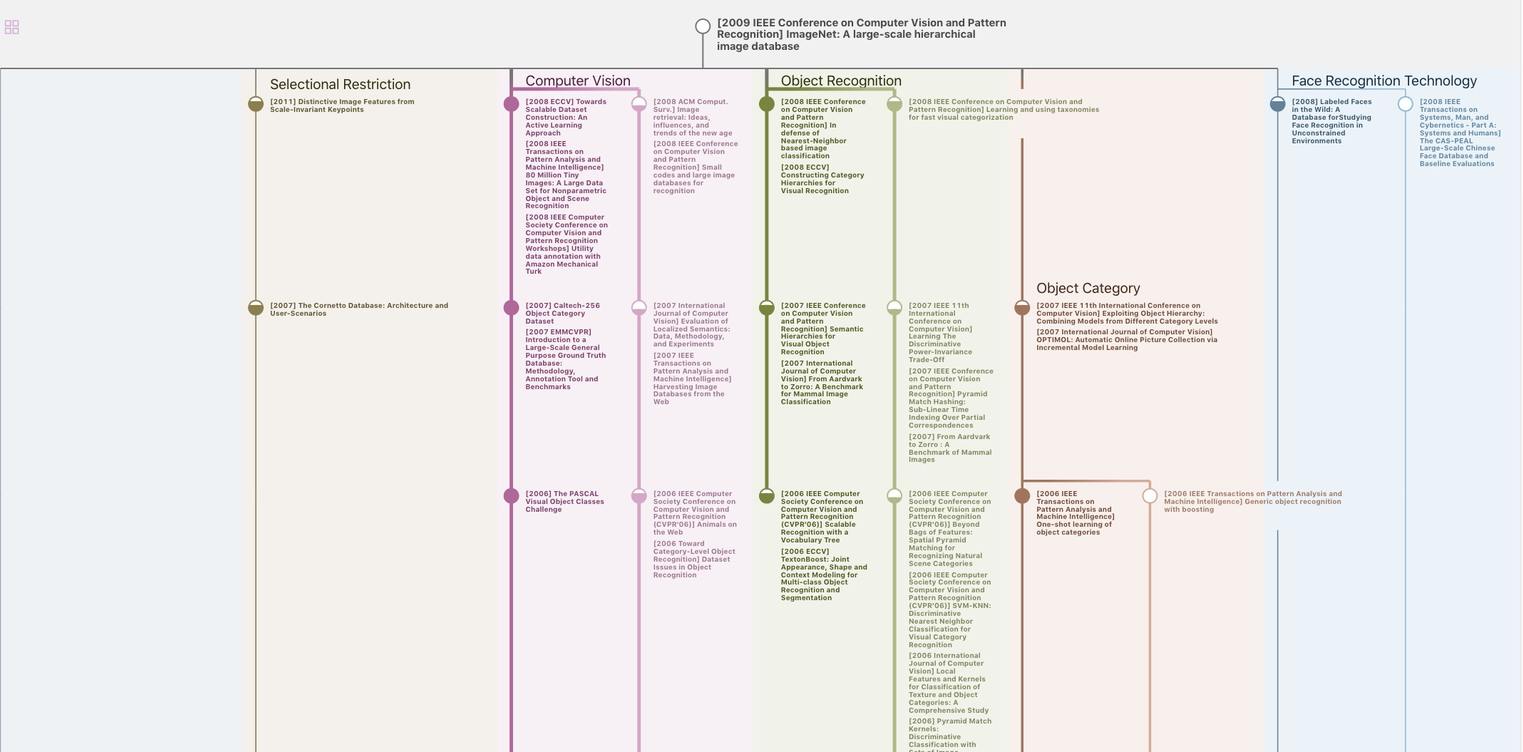
生成溯源树,研究论文发展脉络
Chat Paper
正在生成论文摘要