Representation Learning on Graphs with Jumping Knowledge Networks.
ICML(2018)
摘要
Recent deep learning approaches for representation learning on graphs follow a neighborhood aggregation procedure. We analyze some important properties of these models, and propose a strategy to overcome those. In particular, the range of neighboring nodes that a nodeu0027s representation draws from strongly depends on the graph structure, analogous to the spread of a random walk. To adapt to local neighborhood properties and tasks, we explore an architecture -- jumping knowledge (JK) networks -- that flexibly leverages, for each node, different neighborhood ranges to enable better structure-aware representation. In a number of experiments on social, bioinformatics and citation networks, we demonstrate that our model achieves state-of-the-art performance. Furthermore, combining the JK framework with models like Graph Convolutional Networks, GraphSAGE and Graph Attention Networks consistently improves those modelsu0027 performance.
更多查看译文
AI 理解论文
溯源树
样例
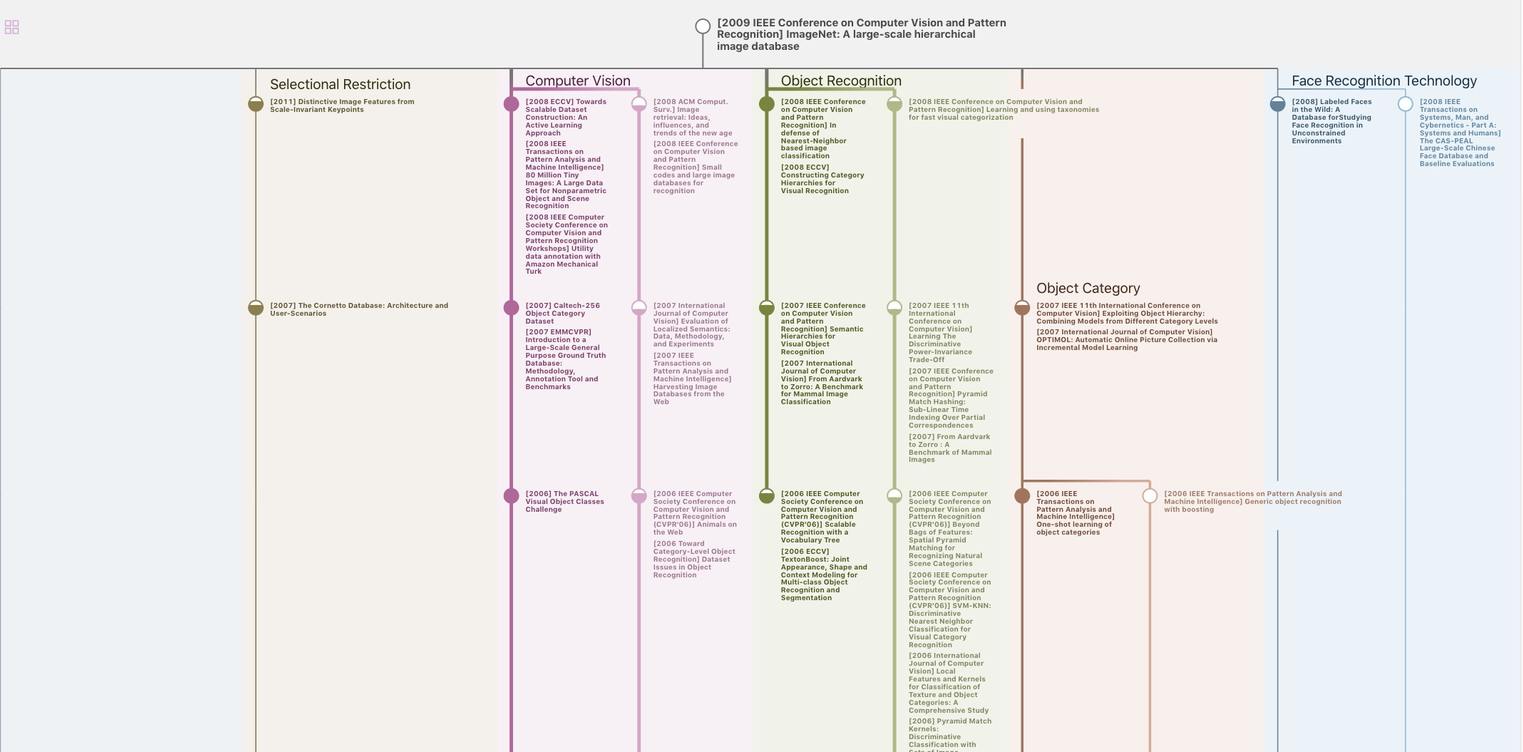
生成溯源树,研究论文发展脉络
Chat Paper
正在生成论文摘要