Subspace Embedding and Linear Regression with Orlicz Norm.
ICML(2018)
摘要
We consider a generalization of the classic linear regression problem to the case when the loss is an Orlicz norm. An Orlicz norm is parameterized by a non-negative convex function $G:mathbb{R}_+rightarrowmathbb{R}_+$ with $G(0)=0$: the Orlicz norm of a vector $xinmathbb{R}^n$ is defined as $ |x|_G=infleft{alphau003e0largemidsum_{i=1}^n G(|x_i|/alpha)leq 1right}. $ We consider the cases where the function $G(cdot)$ grows subquadratically. Our main result is based on a new oblivious embedding which embeds the column space of a given matrix $Ainmathbb{R}^{ntimes d}$ with Orlicz norm into a lower dimensional space with $ell_2$ norm. Specifically, we show how to efficiently find an embedding matrix $Sinmathbb{R}^{mtimes n},mu003cn$ such that $forall xinmathbb{R}^{d},Omega(1/(dlog n)) cdot |Ax|_Gleq |SAx|_2leq O(d^2log n) cdot |Ax|_G.$ By applying this subspace embedding technique, we show an approximation algorithm for the regression problem $min_{xinmathbb{R}^d} |Ax-b|_G$, up to a $O(dlog^2 n)$ factor. As a further application of our techniques, we show how to also use them to improve on the algorithm for the $ell_p$ low rank matrix approximation problem for $1leq pu003c2$.
更多查看译文
关键词
orlicz norm,linear regression
AI 理解论文
溯源树
样例
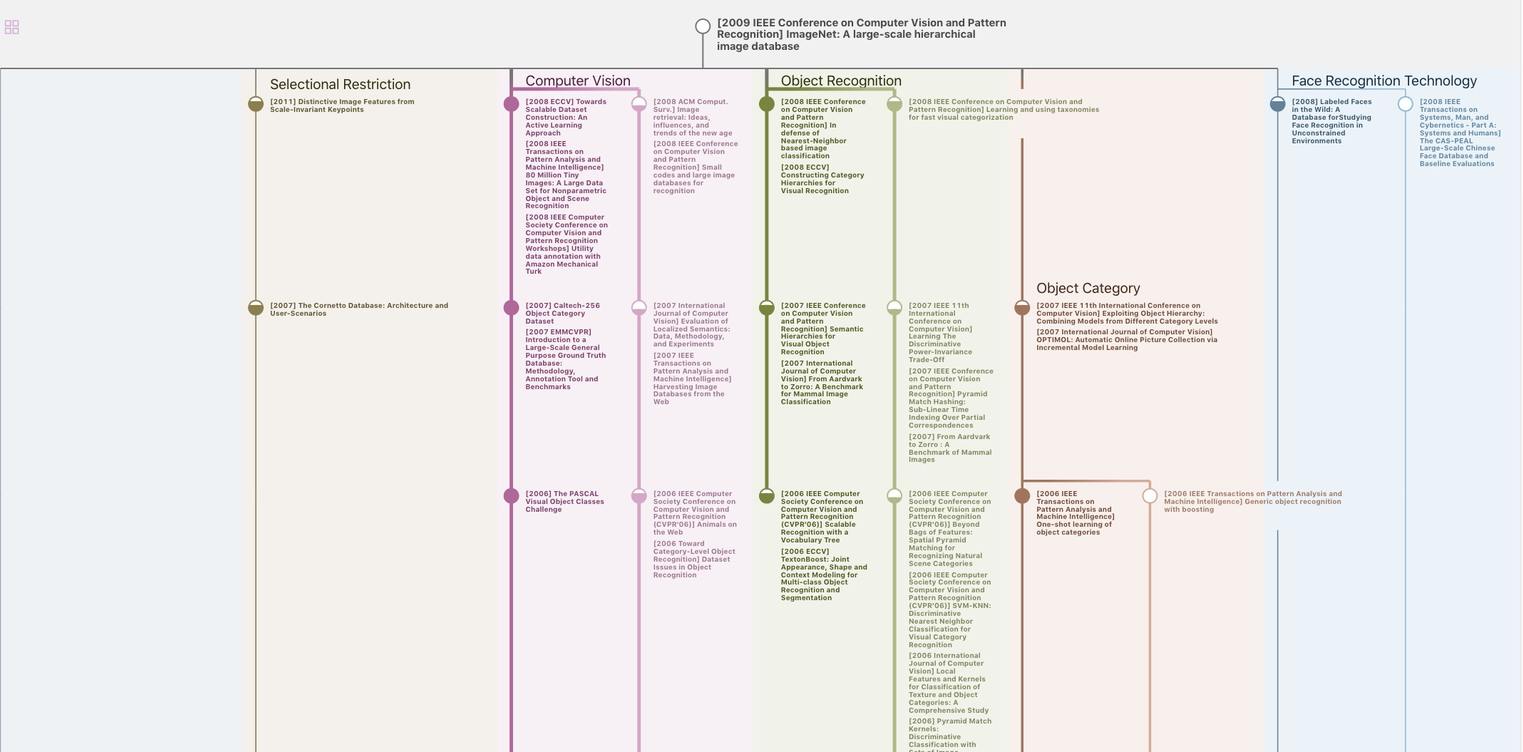
生成溯源树,研究论文发展脉络
Chat Paper
正在生成论文摘要