Deep Domain Adaptation Based on Multi-layer Joint Kernelized Distance.
SIGIR(2018)
摘要
Domain adaptation refers to the learning scenario where a model learned from the source data is applied on the target data which have the same categories but different distributions. In information retrieval, there exist application scenarios like cross domain recommendation characterized similarly. In this paper, by utilizing deep features extracted from the deep networks, we proposed to compute the multi-layer joint kernelized mean distance between the k th target data predicted as the i th category and all the source data of the j th category $d_ij ^k$. Then, target data $T_m$ that are most likely to belong to the i th category can be found by calculating the relative distance $d_ii ^k/\sum_j d_ij ^k$. By iteratively adding $T_m$ to the training data, the finetuned deep model can adapt on the target data progressively. Our results demonstrate that the proposed method can achieve a better performance compared to a number of state-of-the-art methods.
更多查看译文
AI 理解论文
溯源树
样例
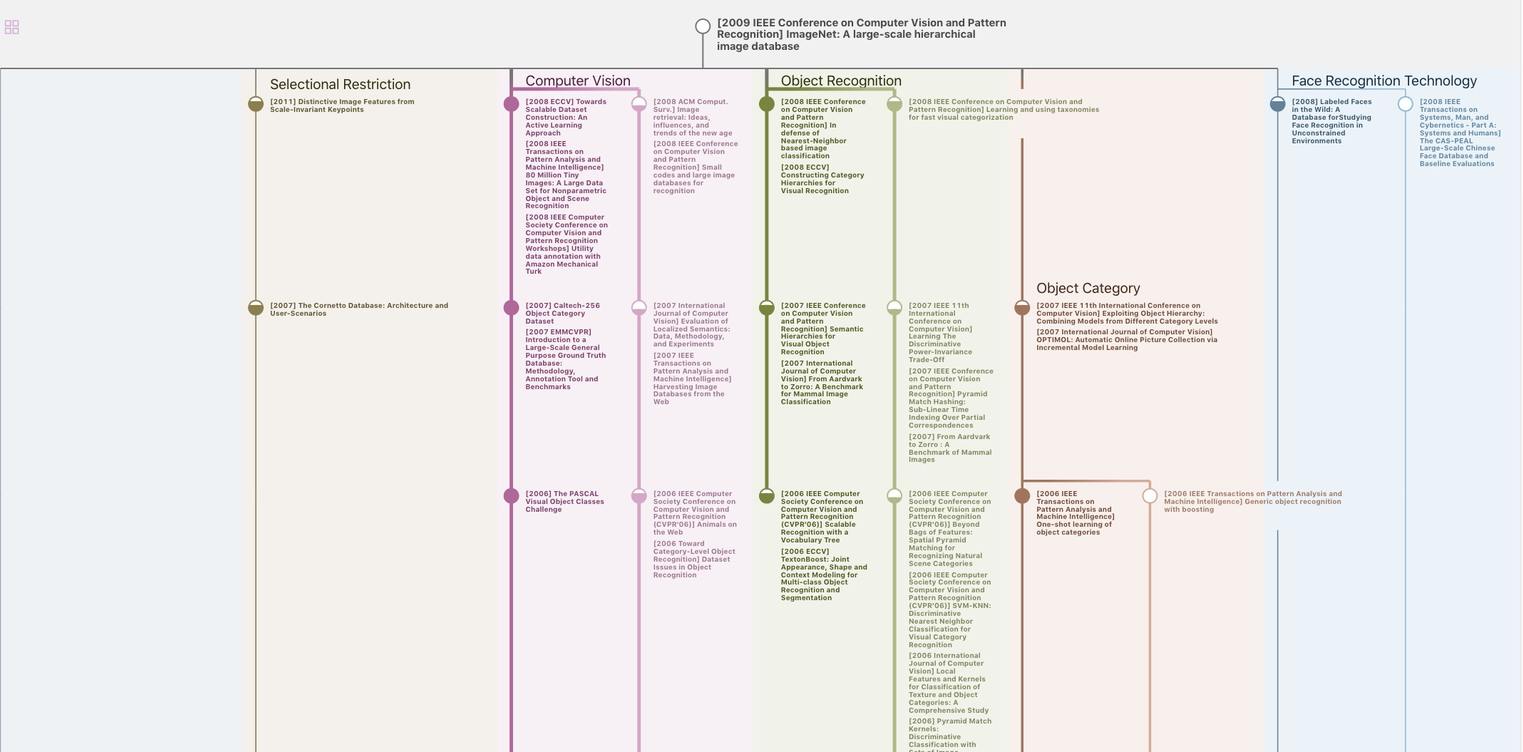
生成溯源树,研究论文发展脉络
Chat Paper
正在生成论文摘要