A Hybrid Neural Machine Translation Technique for Translating Low Resource Languages.
MLDM(2018)
摘要
Neural machine translation (NMT) has produced very promising results on various high resource languages that have sizeable parallel datasets. However, low resource languages that lack sufficient parallel datasets face challenges in the automated translation filed. The main part of NMT is a recurrent neural network, which can work with sequential data at word and sentence levels, given that sequences are not too long. Due to the large number of word and sequence combinations, a parallel dataset is required, which unfortunately is not always available, particularly for low resource languages. Therefore, we adapted a character neural translation model that was based on a combined structure of recurrent neural network and convolutional neural network. This model was trained on the IWSLT 2016 Arabic—English and the IWSLT 2015 English—Vietnamese datasets. The model produced encouraging results particularly on the Arabic datasets, where Arabic is considered a rich morphological language.
更多查看译文
关键词
translation,languages
AI 理解论文
溯源树
样例
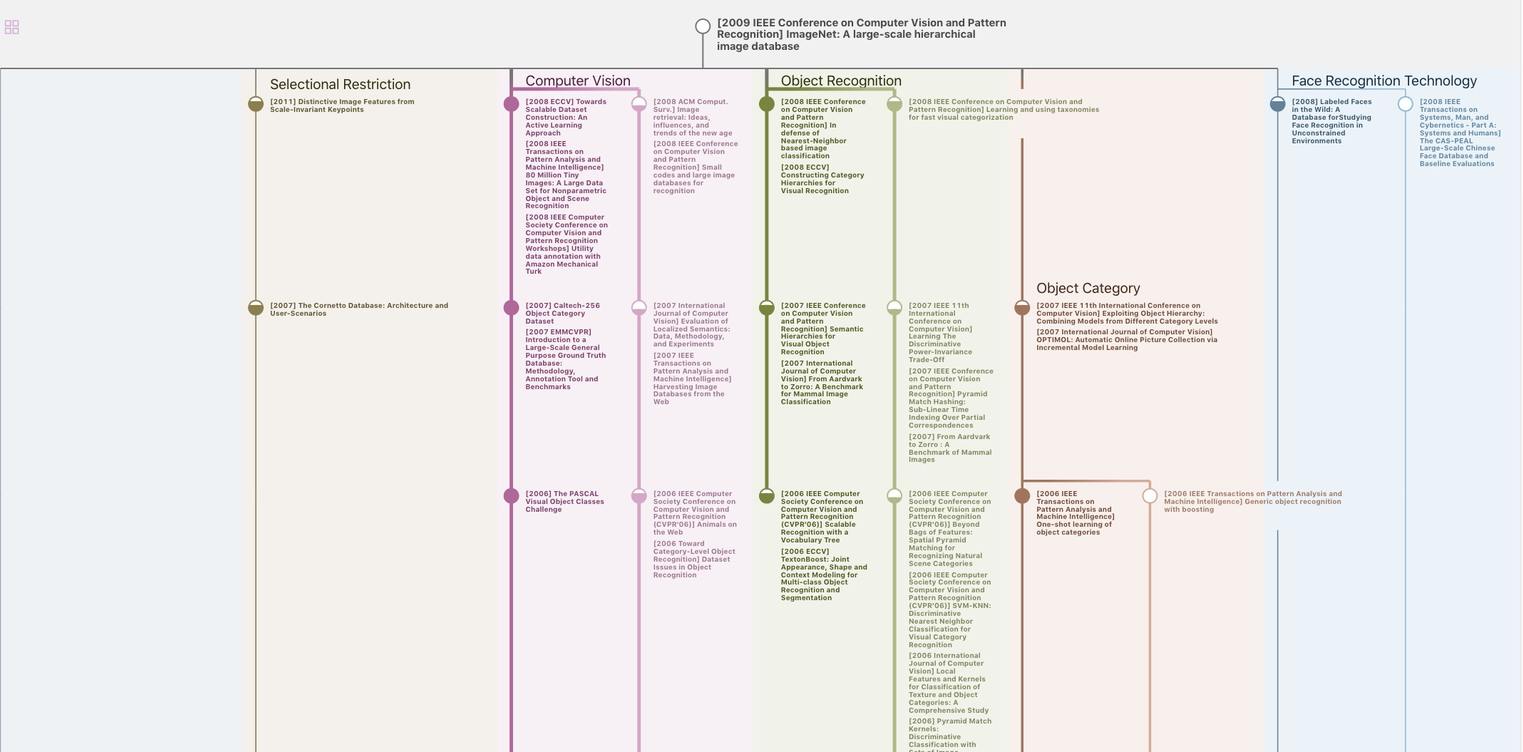
生成溯源树,研究论文发展脉络
Chat Paper
正在生成论文摘要