A generic distributed microservices and container based framework for metaheuristic optimization.
GECCO (Companion)(2018)
摘要
In recent years, metaheuristics have been a convincing solution for solving many types of complex optimization problems. Efficient execution for the most variants of metaheuristics e.g. Evolutionary Algorithms (EAs) or Swarm Optimization can require lots of computational power depending on the complexity of the application. For maximizing performance, parallelization of metaheuristic algorithms represents a meaningful approach, especially for EAs featuring an inherent parallelism. However, the majority of existing distributed optimization frameworks are implemented as monolithic and non-distributed applications running on one computer instead of using the computing power of computer clusters. Additionally, the application typically cannot easily work dynamically together with other tools and services like single simulators or combinations of simulators for multi-domain simulations. In the present paper, a new distributed framework for population-based metaheuristics, which can run highly parallelized optimizations on large scale computing clusters is introduced. The framework exploits and combines two lightweight technologies namely container virtualization and microservices for a fully automated and highly scalable solution. Another main advantage of the framework is that it allows plugging in and out different metaheuristic optimization algorithms and services via its modular distributed architecture. In order to validate the new design and implementation, the EA GLEAM (General Learning Evolutionary Algorithm and Method) [2, 3] is integrated into the framework and deployed on a cluster with 4 nodes and 128 cores for benchmarking. The tested master-slave parallelization of this EA shows considerable low overhead times and hence paves the way for future applications in, e.g., smart energy systems.
更多查看译文
AI 理解论文
溯源树
样例
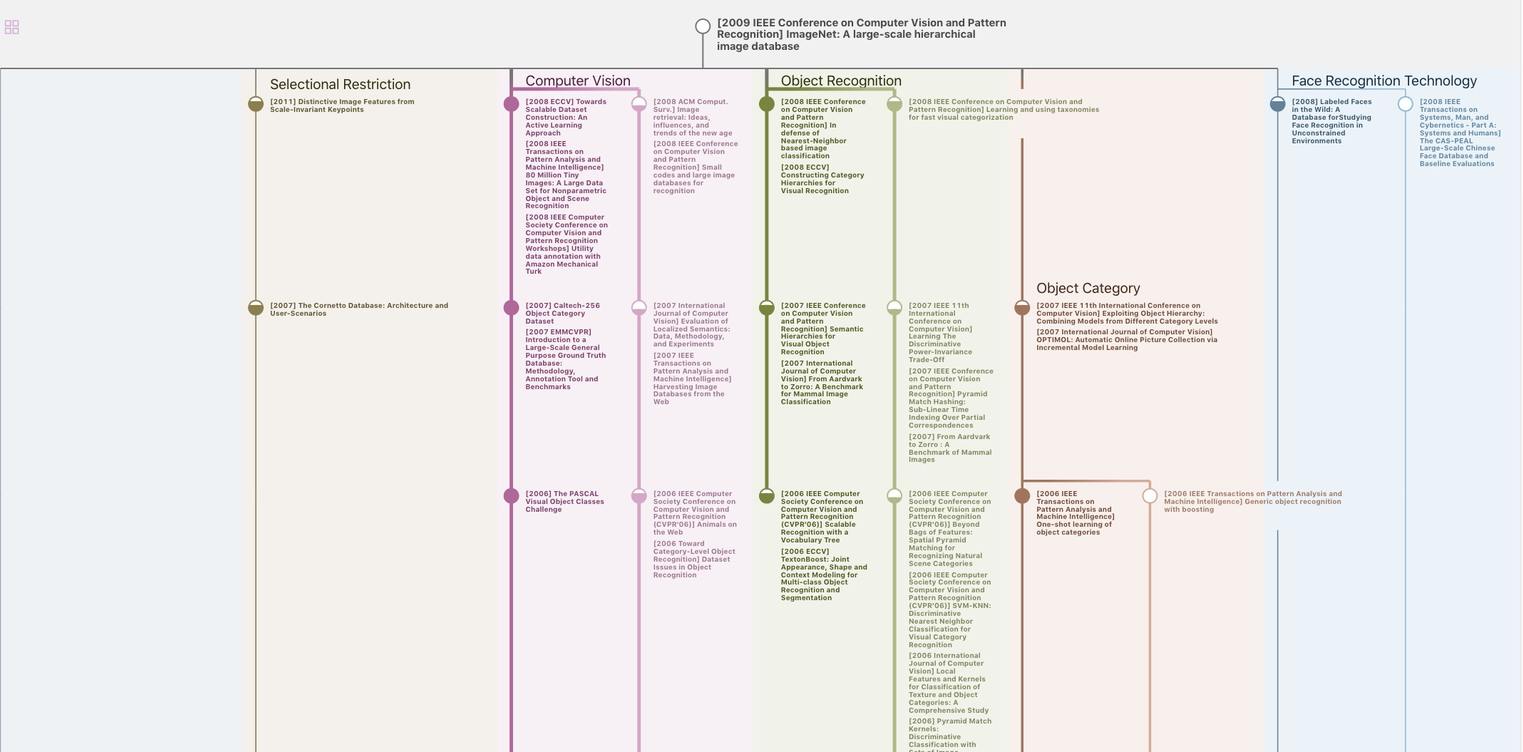
生成溯源树,研究论文发展脉络
Chat Paper
正在生成论文摘要