A new analysis method for evolutionary optimization of dynamic and noisy objective functions.
GECCO(2018)
摘要
Evolutionary algorithms, being problem-independent and randomized heuristics, are generally believed to be robust to dynamic changes and noisy access to the problem instance. We propose a new method to obtain rigorous runtime results for such settings. In contrast to many previous works, our new approach mostly relies on general parameters of the dynamics or the noise models, such as the expected change of the dynamic optimum or the probability to have a dynamic change in one iteration. Consequently, we obtain bounds which are valid for large varieties of such models. Despite this generality, for almost all particular models regarded in the past our bounds are stronger than those given in previous works. As one particular result, we prove that the (1 + λ) EA can optimize the OneMax benchmark function efficiently despite a constant rate of 1-bit flip noise. For this, a logarithmic size offspring population suffices (the previous-best result required a super-linear value of λ). Our results suggest that the typical way to find the optimum in such adverse settings is not via a steady approach of the optimum, but rather via an exceptionally fast approach after waiting for a rare phase of low dynamic changes or noise.
更多查看译文
关键词
Dynamic optimization, noisy optimization, runtime analysis
AI 理解论文
溯源树
样例
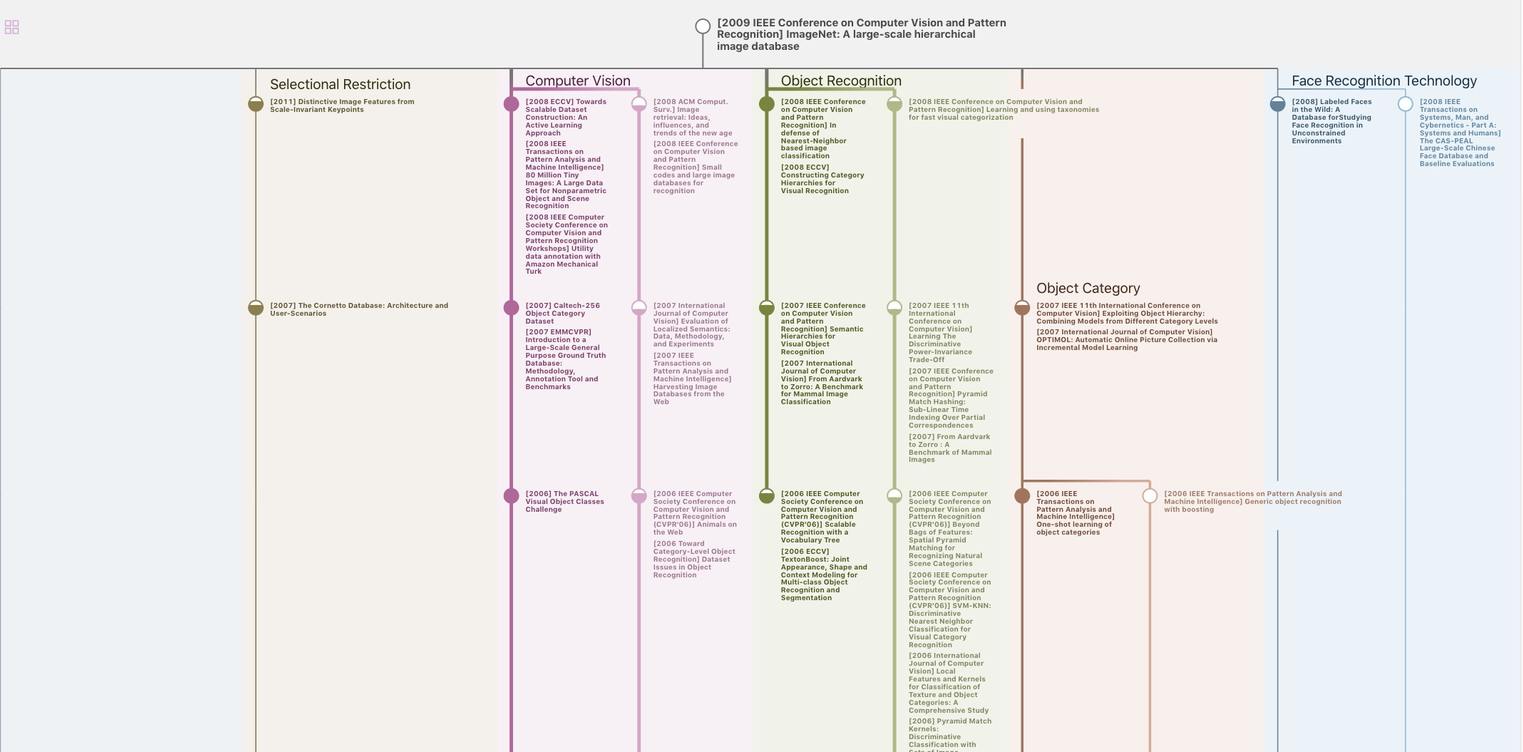
生成溯源树,研究论文发展脉络
Chat Paper
正在生成论文摘要