A Multi-Task Approach To Learning Multilingual Representations
PROCEEDINGS OF THE 56TH ANNUAL MEETING OF THE ASSOCIATION FOR COMPUTATIONAL LINGUISTICS, VOL 2(2018)
摘要
We present a novel multi-task modeling approach to learning multilingual distributed representations of text. Our system learns word and sentence embeddings jointly by training a multilingual skipgram model together with a cross-lingual sentence similarity model. Our architecture can transparently use both monolingual and sentence aligned bilingual corpora to learn multilingual embeddings, thus covering a vocabulary significantly larger than the vocabulary of the bilingual corpora alone. Our model shows competitive performance in a standard crosslingual document classification task. We also show the effectiveness of our method in a limited resource scenario.
更多查看译文
AI 理解论文
溯源树
样例
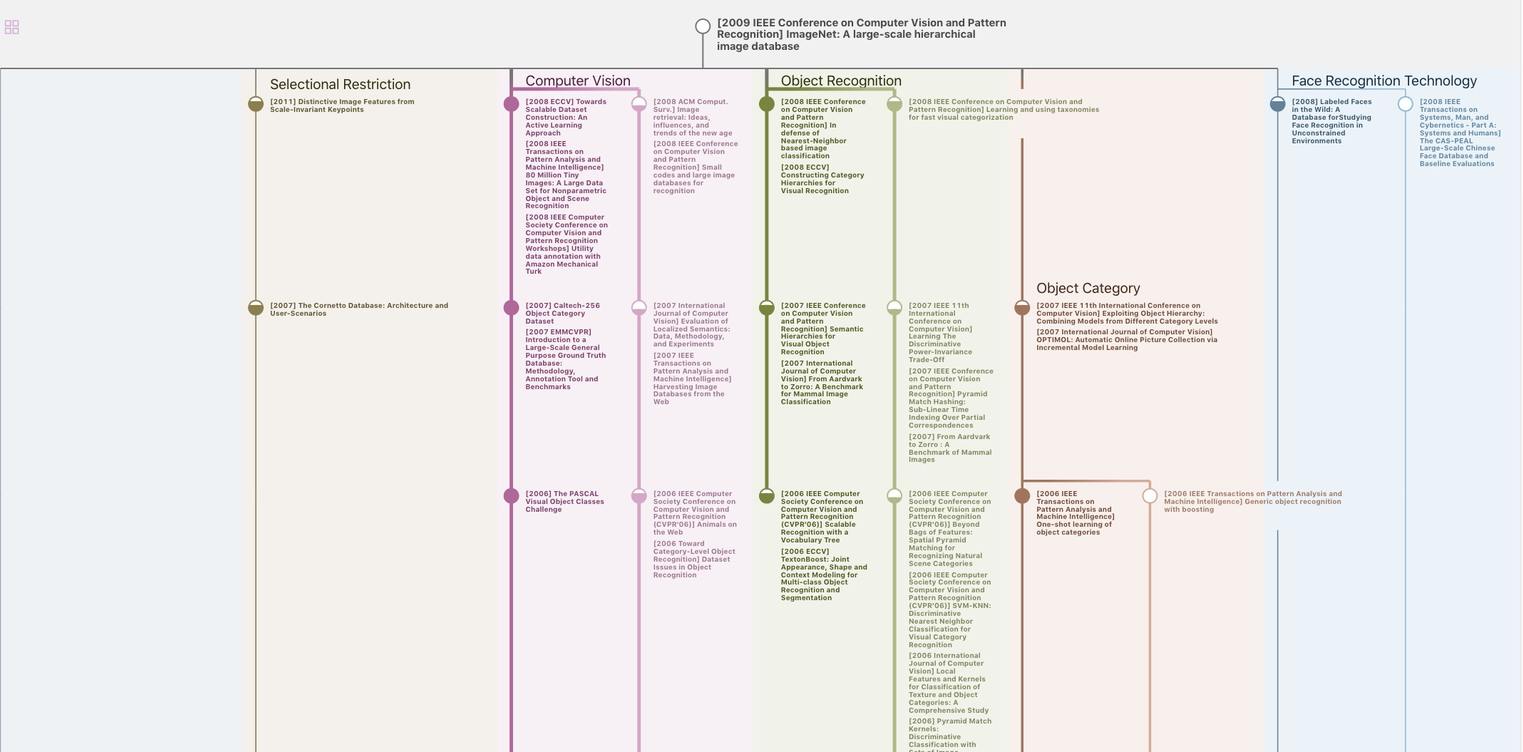
生成溯源树,研究论文发展脉络
Chat Paper
正在生成论文摘要