Collaborative Filtering With Behavioral Models
PROCEEDINGS OF THE 26TH CONFERENCE ON USER MODELING, ADAPTATION AND PERSONALIZATION (UMAP'18)(2018)
摘要
Collaborative iltering (CF) has made it possible to build personalized recommendation models leveraging the collective data of large user groups, albeit with prescribed models that cannot easily leverage the existence of known behavioral models in particular settings. In this paper, we facilitate the combination of CF with existing behavioral models by introducing Bayesian Behavioral Collaborative Filtering (BBCF). BBCF works by embedding arbitrary (black-box) probabilistic models of human behavior in a latent variable Bayesian framework capable of collectively leveraging behavioral models trained on all users for personalized recommendation. There are three key advantages of BBCF compared to traditional CF and non-CF methods: (1) BBCF can leverage highly specialized behavioral models for speciic CF use cases that may outperform existing generic models used in standard CF, (2) the behavioral models used in BBCF may ofer enhanced intepretability and explainability compared to generic CF methods, and (3) compared to non-CF methods that would train a behavioral model per speciic user and thus may sufer when individual user data is limited, BBCF leverages the data of all users thus enabling strong performance across the data availability spectrum including the near cold-start case. Experimentally, we compare BBCF to individual and global behavioral models as well as CF techniques; our evaluation domains span sequential and non-sequential tasks with a range of behavioral models for individual users, tasks, or goal-oriented behavior. Our results demonstrate that BBCF is competitive if not better than existing methods while still ofering the interpretability and explainability beneits intrinsic to many behavioral models.
更多查看译文
关键词
collaborative filtering, behavioral modeling, bayesian model averaging
AI 理解论文
溯源树
样例
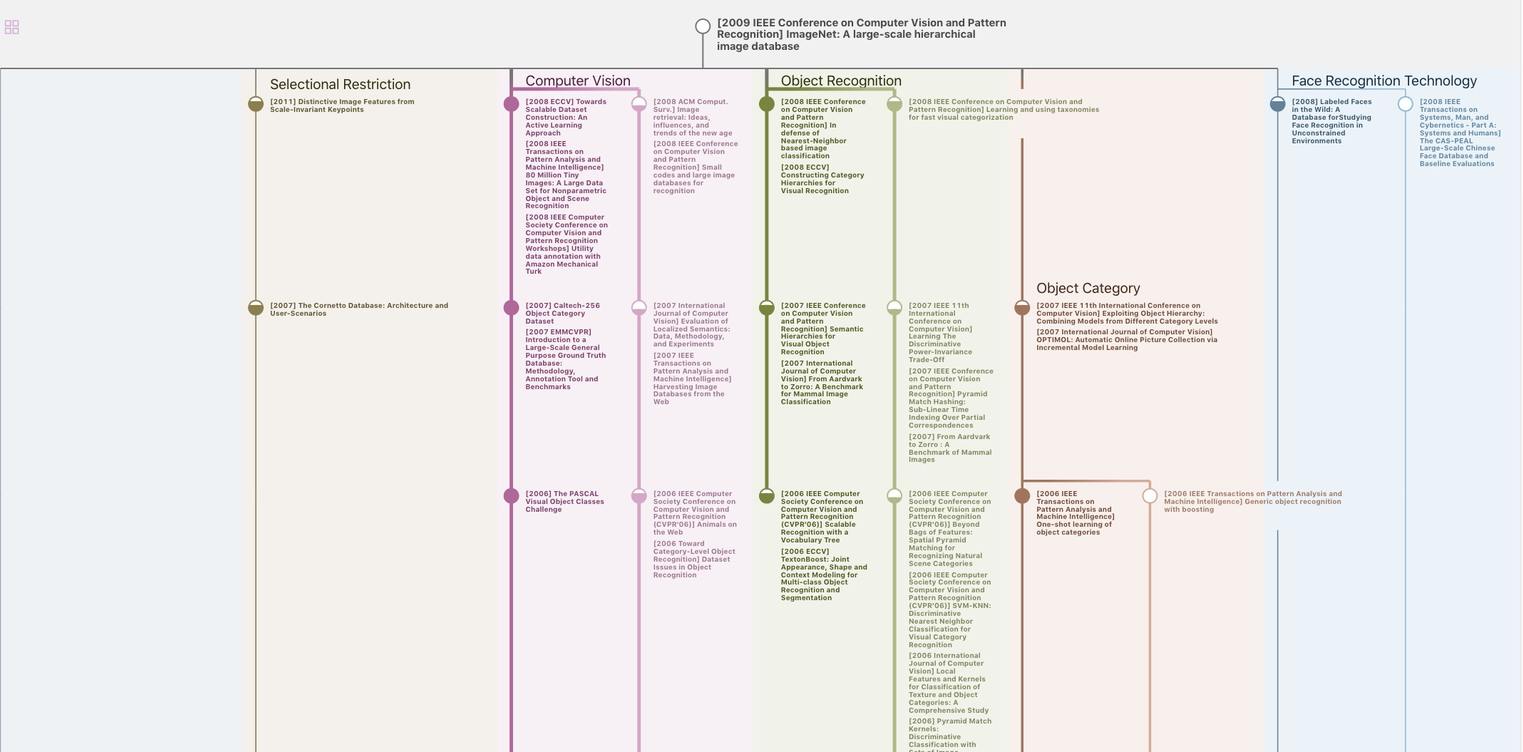
生成溯源树,研究论文发展脉络
Chat Paper
正在生成论文摘要