Acgail: Imitation Learning About Multiple Intentions With Auxiliary Classifier Gans
PRICAI 2018: TRENDS IN ARTIFICIAL INTELLIGENCE, PT I(2018)
摘要
As an important solution to decision-making problems, imitation learning learns expert behavior from example demonstrations provided by experts, without the necessity of a predefined reward function as in reinforcement learning. Traditionally, imitation learning assumes that demonstrations are generated from single latent expert intention. One promising method in this line is generative adversarial imitation learning (GAIL), designed to work in large environments. It can be thought as a model-free imitation learning built on top of generative adversarial networks (GANs). However, GAIL fails to learn well when handling expert demonstrations under multiple intentions, which can be labeled by latent intentions. In this paper, we propose to add an auxiliary classifier model to GAIL, from which we derive a novel variant of GAIL, named ACGAIL, allowing label conditioning in imitation learning about multiple intentions. Experimental results on several MuJoCo tasks indicate that ACGAIL can achieve significant performance improvements over existing methods, e.g., GAIL and InfoGAIL, when dealing with label-conditional imitation learning about multiple intentions.
更多查看译文
关键词
Imitation learning, Generative adversarial networks, Multiple intentions, Auxiliary classifier
AI 理解论文
溯源树
样例
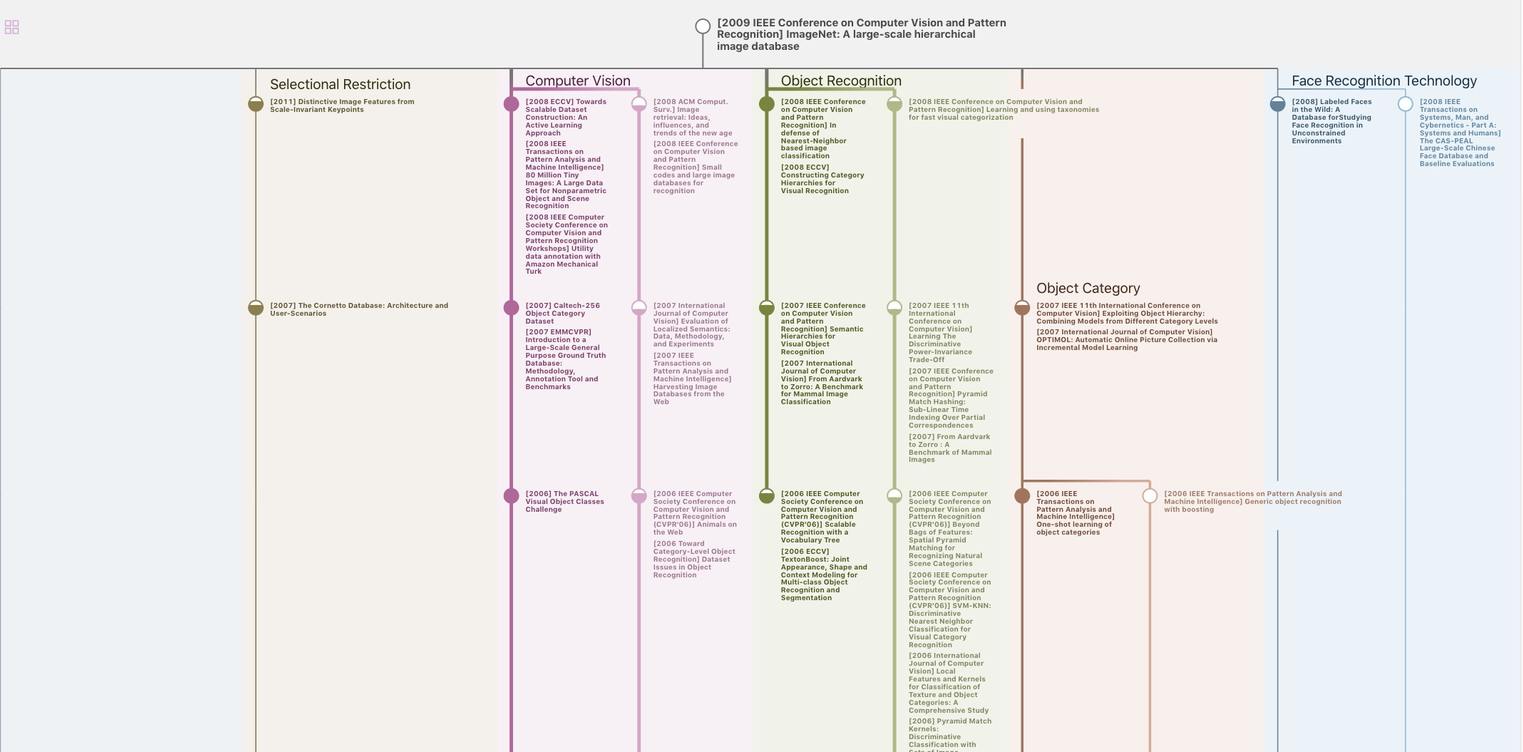
生成溯源树,研究论文发展脉络
Chat Paper
正在生成论文摘要