Multiagent Reinforcement Learning Methods To Resolve Demand Capacity Balance Problems
10TH HELLENIC CONFERENCE ON ARTIFICIAL INTELLIGENCE (SETN 2018)(2018)
摘要
In this article, we explore the computation of joint policies for autonomous agents to resolve congestions problems in the air traffic management (ATM) domain. Agents, representing flights, have limited information about others' payoffs and preferences, and need to coordinate to achieve their tasks while adhering to operational constraints. We formalize the problem as a multiagent Markov decision process (MDP) towards deciding flight delays to resolve demand and capacity balance (DCB) problems in ATM. To this end, we present multiagent reinforcement learning methods that allow agents to interact and form own policies in coordination with others. Experimental study on real-world cases, confirms the effectiveness of our approach in resolving the demand-capacity balance problem.
更多查看译文
关键词
Multi-agent reinforcement learning, congestion problems, Air Traffic Management, Demand Capacity Balance
AI 理解论文
溯源树
样例
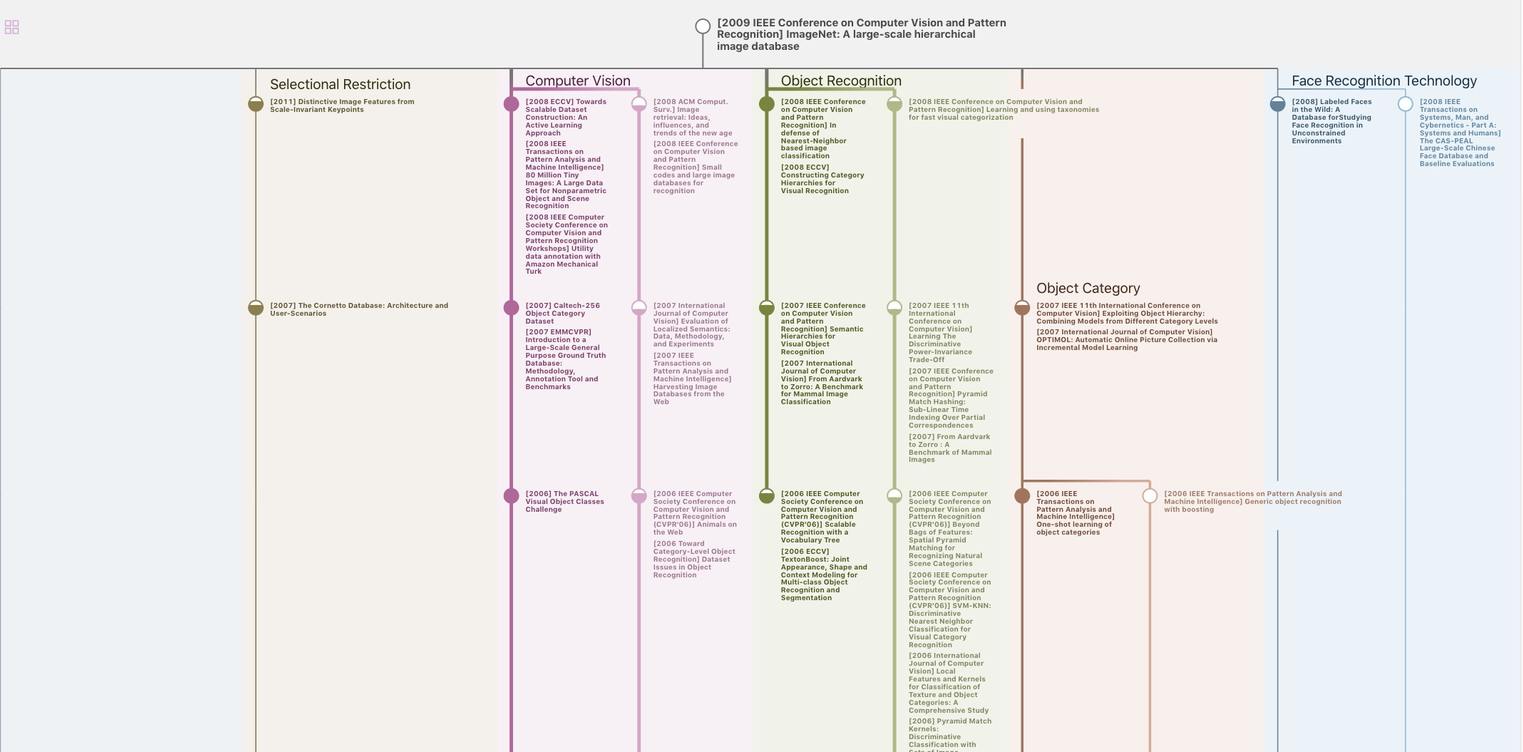
生成溯源树,研究论文发展脉络
Chat Paper
正在生成论文摘要