Self Inertia Weight Adaptation for the Particle Swarm Optimization.
LOPAL(2018)
摘要
Particle swarm optimization is a stochastic population-based metaheuristic algorithm, it been successful in solving a height range of real-world problems. The primary challenge present in PSO is to balance between global and local search during the optimization process. The classical PSO algorithm applies the same search scheme for all particles, which implies that all the swarm uses the same strategy. This monotonic learning design produces the be short of learning ability for a single particle that makes the classical PSO unable to deal with diverse and complex optimization problems. In this paper, we present a new learning design for particles in the PSO algorithm. In the proposed approach, each particle balances between four learning strategies to influence and adapt its inertia weight. The self-adaptation is controlled by a Hidden Markov Chain of each particle's states, which allows incorporating both the particle history and the current situation of the search process in the individual learning level of the swarm. The experimental study on a set of several benchmark functions shows remarkable performances in comparison with other PSO variances from literature.
更多查看译文
AI 理解论文
溯源树
样例
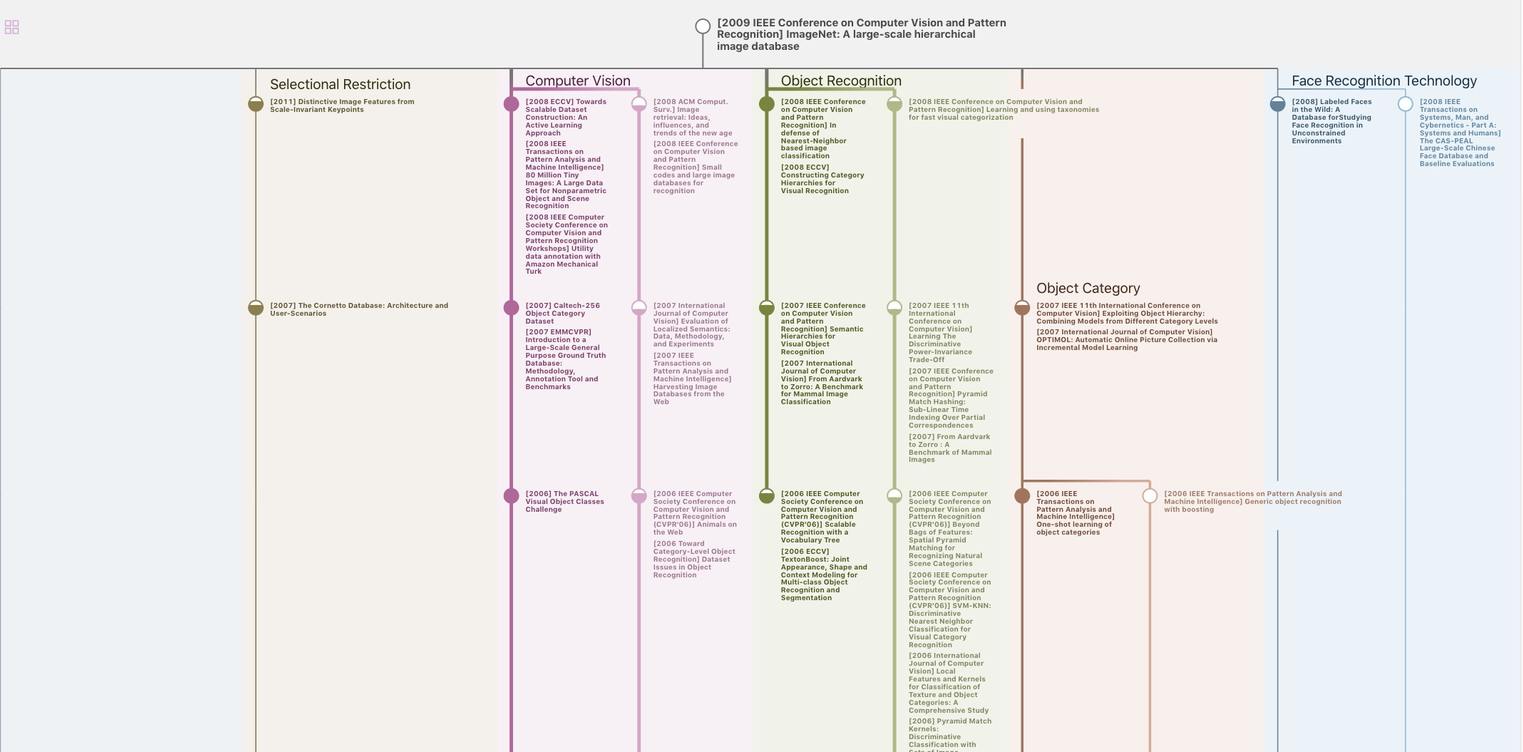
生成溯源树,研究论文发展脉络
Chat Paper
正在生成论文摘要