A Normalized Convolutional Neural Network for Guided Sparse Depth Upsampling.
IJCAI(2018)
摘要
Guided sparse depth upsampling aims to upsample an irregularly sampled sparse depth map when an aligned high-resolution color image is given as guidance. When deep convolutional neural networks (CNNs) become the optimal choice to many applications nowadays, how to deal with irregular and sparse data still remains a non-trivial problem. Inspired by the classical normalized convolution operation, this work proposes a normalized convolutional layer (NCL) implemented in CNNs. Sparse data are therefore explicitly considered in CNNs by the separation of both data and filters into a signal part and a certainty part. Based upon NCLs, we design a normalized convolutional neural network (NCNN) to perform guided sparse depth upsampling. Experiments on both indoor and outdoor datasets show that the proposed NCNN models achieve state-of-the-art upsampling performance. Moreover, the models using NCLs gain a great generalization ability to different sparsity levels.
更多查看译文
AI 理解论文
溯源树
样例
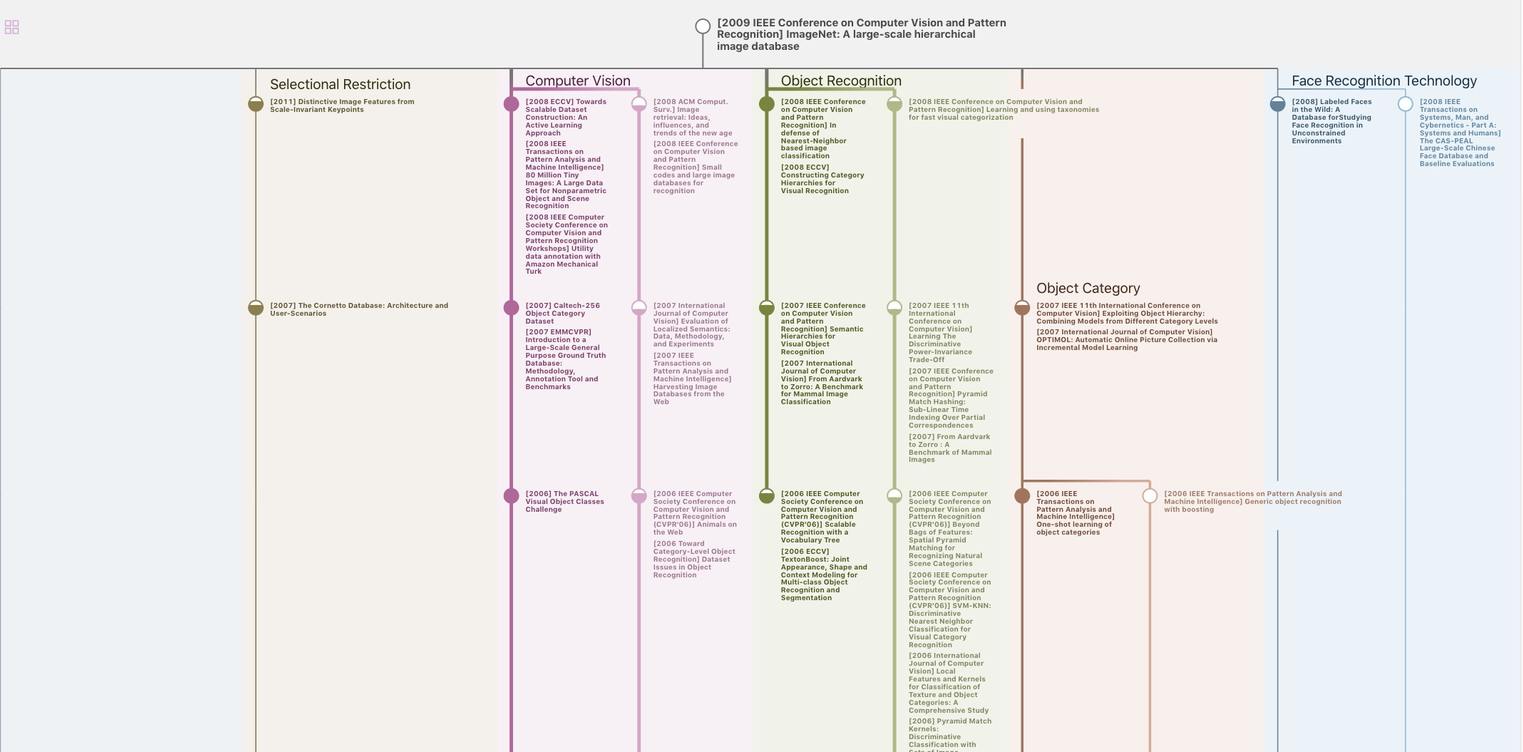
生成溯源树,研究论文发展脉络
Chat Paper
正在生成论文摘要