Crowdlearning: Crowded Deep Learning with Data Privacy
2018 15th Annual IEEE International Conference on Sensing, Communication, and Networking (SECON)(2018)
摘要
Deep Learning has shown promising performance in a variety of pattern recognition tasks owning to large quantities of training data and complex structures of neural networks. However conventional deep neural network (DNN) training involves centrally collecting and storing the training data, and then centrally training the neural network, which raises much privacy concerns for the data producers. In this paper, we study how to enable deep learning without disclosing individual data to the DNN trainer. We analyze the risks in conventional deep learning training, then propose a novel idea - Crowdlearning, which decentralizes the heavy- load training procedure and deploys the training into a crowd of computation-restricted mobile devices who generate the training data. Finally, we propose SliceNet, which ensures mobile devices can afford the computation cost and simultaneously minimize the total communication cost. The combination of Crowdlearning and SliceNet ensures the sensitive data generated by mobile devices never leave the devices, and the training procedure will hardly disclose any inferable contents. We numerically simulate our prototype of SliceNet which crowdlearns an accurate DNN for image classification, and demonstrate the high performance, acceptable calculation and communication cost, satisfiable privacy protection, and preferable convergence rate, on the benchmark DNN structure and dataset.
更多查看译文
关键词
data privacy,conventional deep neural network training,conventional deep learning training,crowdlearning,crowded deep learning,computation-restricted mobile devices,SliceNet
AI 理解论文
溯源树
样例
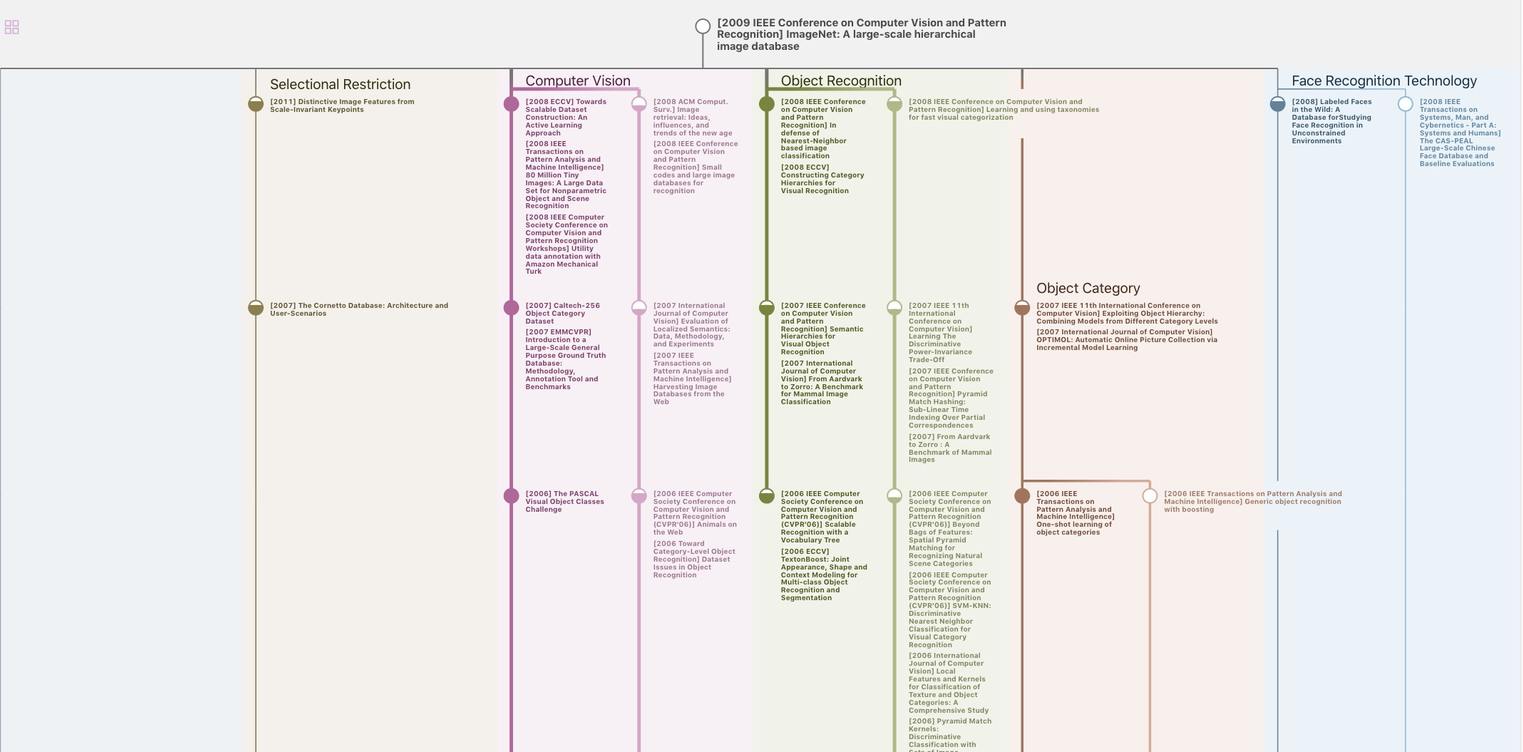
生成溯源树,研究论文发展脉络
Chat Paper
正在生成论文摘要