A label ranking approach for selecting rankings of collaborative filtering algorithms.
SAC 2018: Symposium on Applied Computing Pau France April, 2018(2018)
摘要
The large amount of Recommender System algorithms makes the selection of the most suitable algorithm for a new dataset a difficult task. Metalearning has been successfully used to deal with this problem. It works by mapping dataset characteristics with the predictive performance obtained by a set of algorithms. The models built on this data are capable of predicting the best algorithm for a new dataset. However, typical approaches try only to predict the best algorithm, overlooking the performance of others. This study focus on the use of Metalearning to select the best ranking of CF algorithms for a new recommendation dataset. The contribution lies in the formalization and experimental validation of using Label Ranking to select a ranked list of algorithms. The experimental procedure proves the superior performance of the proposed approach regarding both ranking accuracy and impact on the baselevel performance. Furthermore, it draws and compares the knowledge regarding metafeature importance for both classification and Label Ranking tasks in order to provide guidelines for the design of algorithms in the Recommender System community.
更多查看译文
关键词
Collaborative Filtering, Metalearning, Label Ranking
AI 理解论文
溯源树
样例
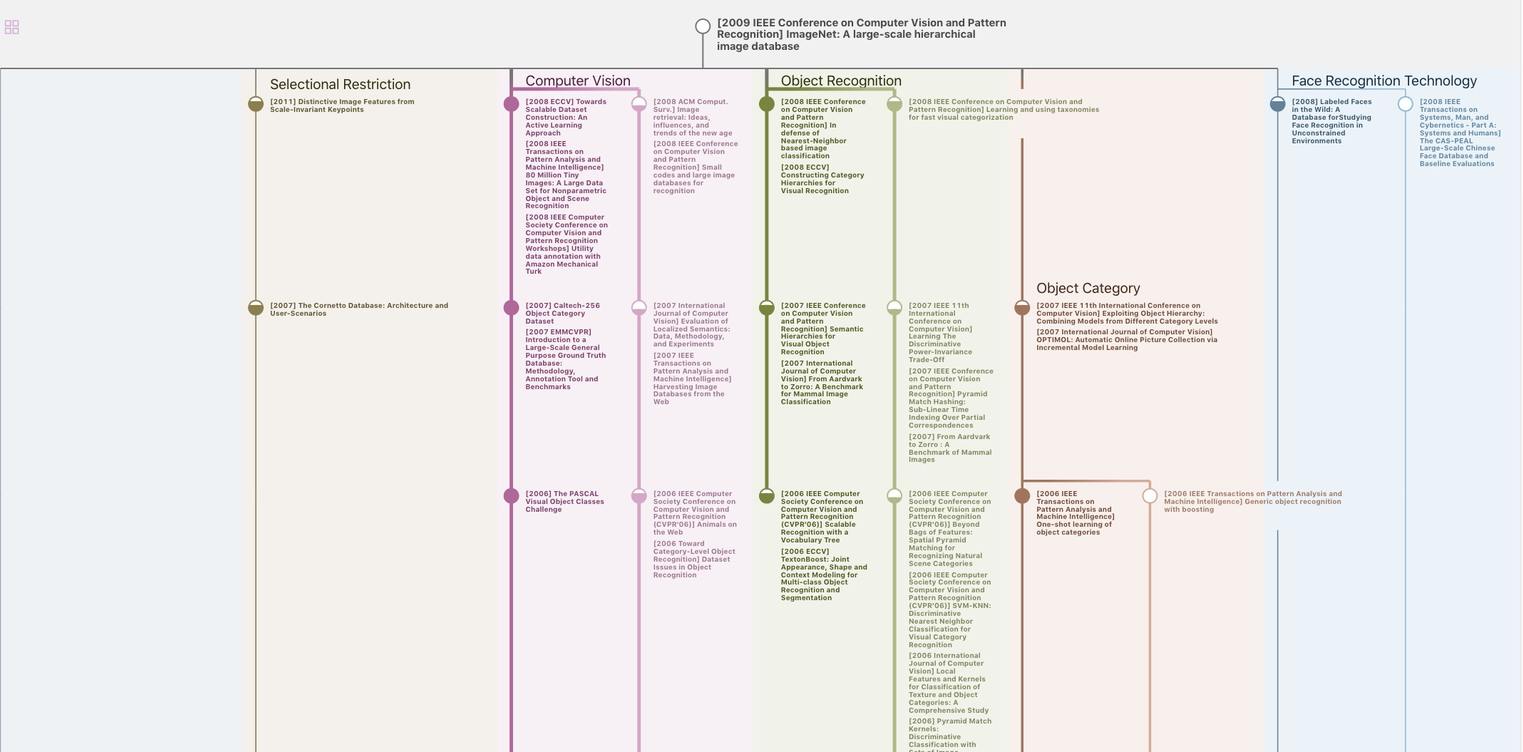
生成溯源树,研究论文发展脉络
Chat Paper
正在生成论文摘要