Unsupervised author disambiguation using Dempster–Shafer theory
Scientometrics(2014)
摘要
The name ambiguity problem presents many challenges for scholar finding, citation analysis and other related research fields. To attack this issue, various disambiguation methods combined with separate disambiguation features have been put forward. In this paper, we offer an unsupervised Dempster–Shafer theory (DST) based hierarchical agglomerative clustering algorithm for author disambiguation tasks. Distinct from existing methods, we exploit the DST in combination with Shannon’s entropy to fuse various disambiguation features and come up with a more reliable candidate pair of clusters for amalgamation in each iteration of clustering. Also, some solutions to determine the convergence condition of the clustering process are proposed. Depending on experiments, our method outperforms three unsupervised models, and achieves comparable performances to a supervised model, while does not prescribe any hand-labelled training data.
更多查看译文
关键词
Author disambiguation,Dempster–Shafer theory of evidence,Hierarchical clustering,Unsupervised
AI 理解论文
溯源树
样例
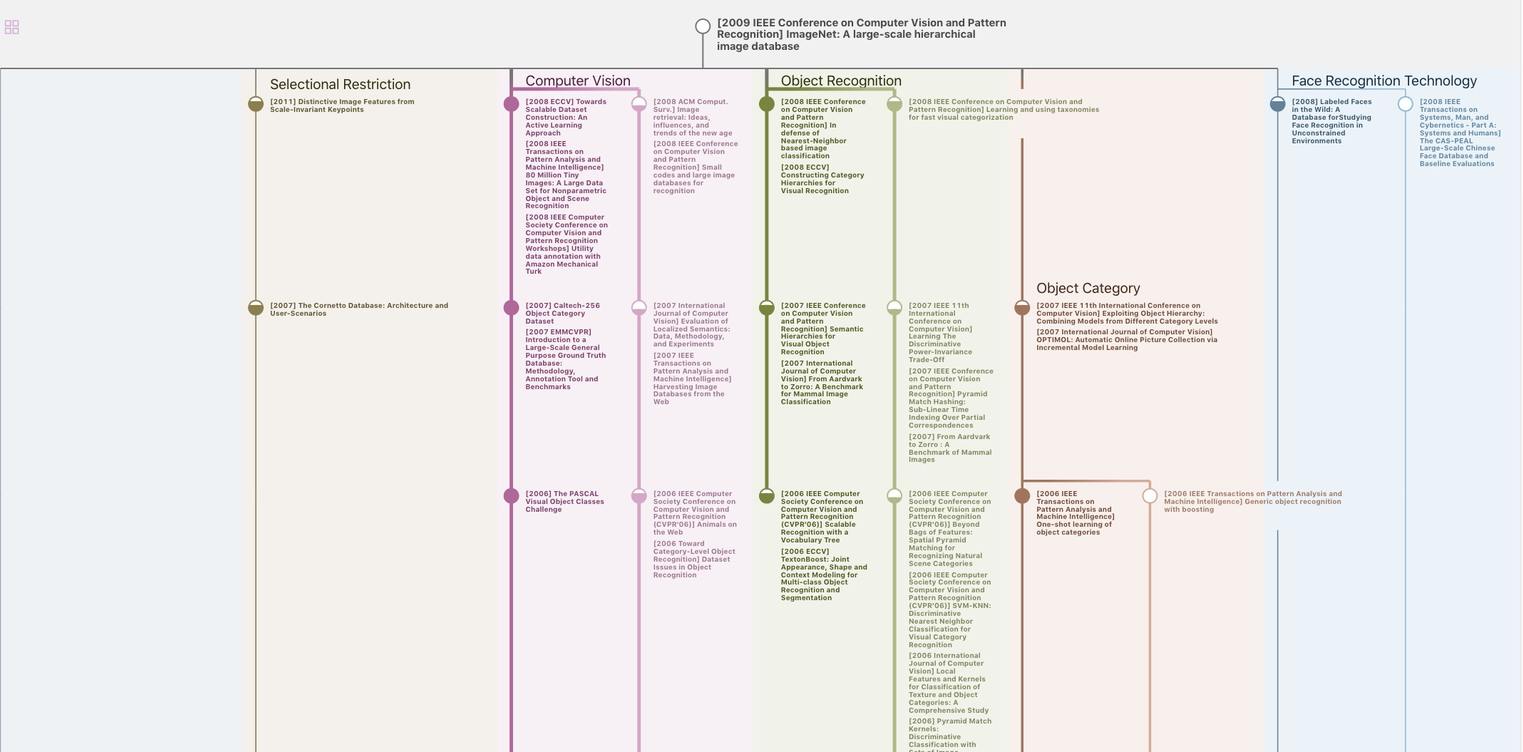
生成溯源树,研究论文发展脉络
Chat Paper
正在生成论文摘要